k折交叉验证python代码
时间: 2023-10-01 21:10:18 浏览: 59
以下是一个简单的 k 折交叉验证的 Python 代码示例:
```python
from sklearn.model_selection import KFold
# 设定 k 值
k = 5
# 创建 KFold 对象
kf = KFold(n_splits=k, shuffle=True)
# 定义数据集
data = [1, 2, 3, 4, 5, 6, 7, 8, 9, 10]
# 遍历每一折
for train_index, val_index in kf.split(data):
# 训练集和验证集的索引
X_train, X_val = [data[i] for i in train_index], [data[i] for i in val_index]
print("训练集:", X_train)
print("验证集:", X_val)
```
在上面的代码中,我们首先设定了 k 值为 5,然后创建了一个 KFold 对象,并传入数据集和 k 值。接下来,我们遍历了每一折,并输出了训练集和验证集的索引。你可以根据自己的需求修改代码来适应不同的数据集和模型。
相关问题
K折交叉验证python代码
下面是使用 scikit-learn 库进行 K 折交叉验证的代码示例:
```python
from sklearn.model_selection import KFold
from sklearn.datasets import load_iris
from sklearn.linear_model import LogisticRegression
import numpy as np
# 加载数据集
iris = load_iris()
X = iris.data
y = iris.target
# 定义 K 折交叉验证器
kf = KFold(n_splits=5, shuffle=True, random_state=42)
# 定义模型
model = LogisticRegression()
# 进行 K 折交叉验证
score_list = []
for train_index, test_index in kf.split(X):
# 获取训练集和测试集
X_train, X_test = X[train_index], X[test_index]
y_train, y_test = y[train_index], y[test_index]
# 训练模型
model.fit(X_train, y_train)
# 评估模型
score = model.score(X_test, y_test)
score_list.append(score)
# 计算平均得分和标准差
mean_score = np.mean(score_list)
std_score = np.std(score_list)
print("Mean score:", mean_score)
print("Std score:", std_score)
```
在上面的代码中,我们使用 `load_iris()` 加载了一个经典的鸢尾花数据集,并使用 `KFold` 定义了一个 5 折交叉验证器。然后,我们定义了一个逻辑回归模型,并在 K 折交叉验证过程中对模型进行了训练和评估。最后,我们计算了所有折的得分的平均值和标准差。你可以根据实际情况修改代码,进行 K 折交叉验证。
连续变量回归的k折交叉验证python代码
连续变量回归的k折交叉验证是一种常用的模型评估方法,可以用于评估回归模型的性能。下面是一个使用Python进行连续变量回归的k折交叉验证的示例代码:
```python
import numpy as np
from sklearn.model_selection import KFold
from sklearn.linear_model import LinearRegression
from sklearn.metrics import mean_squared_error
# 假设有一组特征X和目标变量y
X = np.array([[1, 2], [3, 4], [5, 6], [7, 8]])
y = np.array([3, 5, 7, 9])
# 定义k折交叉验证的折数
k = 5
# 初始化模型和评估指标
model = LinearRegression()
mse_scores = []
# 进行k折交叉验证
kf = KFold(n_splits=k)
for train_index, test_index in kf.split(X):
X_train, X_test = X[train_index], X[test_index]
y_train, y_test = y[train_index], y[test_index]
# 训练模型
model.fit(X_train, y_train)
# 预测并计算均方误差
y_pred = model.predict(X_test)
mse = mean_squared_error(y_test, y_pred)
# 将均方误差添加到列表中
mse_scores.append(mse)
# 计算平均均方误差
mean_mse = np.mean(mse_scores)
# 输出结果
print("Mean Squared Error:", mean_mse)
```
阅读全文
相关推荐














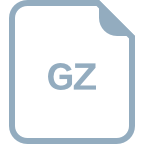