elif count == 7: x_train_one = np.reshape(x_train[:, :, 0], [len(x_train), cold, 1]) x_train_two = np.reshape(x_train[:, :, 1], [len(x_train), cold, 1]) x_train_three = np.reshape(x_train[:, :, 2], [len(x_train), cold, 1]) x_train_four = np.reshape(x_train[:, :, 3], [len(x_train), cold, 1]) x_train_five = np.reshape(x_train[:, :, 4], [len(x_train), cold, 1]) x_train_six = np.reshape(x_train[:, :, 5], [len(x_train), cold, 1]) x_train_seven = np.reshape(x_train[:, :, 6], [len(x_train), cold, 1]) x_test_one = np.reshape(x_test[:, :, 0], [len(x_test), cold, 1]) x_test_two = np.reshape(x_test[:, :, 1], [len(x_test), cold, 1]) x_test_three = np.reshape(x_test[:, :, 2], [len(x_test), cold, 1]) x_test_four = np.reshape(x_test[:, :, 3], [len(x_test), cold, 1]) x_test_five = np.reshape(x_test[:, :, 4], [len(x_test), cold, 1]) x_test_six = np.reshape(x_test[:, :, 5], [len(x_test), cold, 1]) x_test_seven = np.reshape(x_test[:, :, 6], [len(x_test), cold, 1]) input_out = [x_train_one, x_train_two, x_train_three, x_train_four, x_train_five, x_train_six, x_train_seven] layer_out = [x_test_one, x_test_two, x_test_three, x_test_four, x_test_five, x_test_six, x_test_seven] return input_out, layer_out
时间: 2023-10-25 12:09:03 浏览: 43
这段代码是用于将输入数据进行预处理的部分。根据`count`的值,它根据输入数据的维度进行了相应的重塑操作。
首先,对于训练集数据`x_train`,它将第一维度的数据(即[:, :, 0])进行重塑,使得每个样本的维度变为[cold, 1],然后分别赋值给`x_train_one`、`x_train_two`、`x_train_three`等变量。同样的操作也对测试集数据`x_test`进行了处理。
最后,它将处理后的数据存放在`input_out`和`layer_out`列表中,并通过`return`语句将它们作为函数的输出返回。
这段代码的目的是将多维的输入数据转换为适合模型输入的格式,可能是为了满足某个模型或算法的输入要求。具体的处理逻辑可能需要结合代码的上下文来进行更准确的理解。
相关问题
#!/usr/bin/python3 stopword = '' stri = '' try: for line in iter(input, stopword): stri += line + '\n' except EOFError: pass stri = stri[0:-1] # do something... int1_count = 0 str_count = 0 other_count = 0 kong_count = 0 for i in stri: if i.isdigit(): int1_count += 1 elif i.isalnum(): str_count += 1 elif i == ' ': kong_count += 1 else: other_count += 1 print('%d spaces, %d numbers, %d letters, %d other characters.' %(kong_count, int1_count ,str_count,other_count))注释一下
这是一个Python脚本,主要功能是统计用户输入的字符串中包含的空格、数字、字母和其他字符的数量,并输出统计结果。以下是代码注释:
```python
#!/usr/bin/python3 # 声明解释器类型为Python3
stopword = '' # 停止输入的标志字符串
stri = '' # 存储用户输入的字符串
try: # 开始输入循环,直到遇到停止符为止
for line in iter(input, stopword):
stri += line + '\n'
except EOFError: # 如果输入结束,则跳过输入循环
pass
stri = stri[0:-1] # 去掉字符串末尾的换行符
# 统计空格、数字、字母和其他字符的数量
int1_count = 0 # 记录数字的数量
str_count = 0 # 记录字母的数量
other_count = 0 # 记录其他字符的数量
kong_count = 0 # 记录空格的数量
for i in stri:
if i.isdigit(): # 如果是数字,则数字数量加1
int1_count += 1
elif i.isalnum(): # 如果是字母,则字母数量加1
str_count += 1
elif i == ' ': # 如果是空格,则空格数量加1
kong_count += 1
else: # 否则是其他字符,则其他字符数量加1
other_count += 1
# 输出统计结果
print('%d spaces, %d numbers, %d letters, %d other characters.' %(kong_count, int1_count ,str_count,other_count))
```
在脚本中,我们使用`try...except`语句来实现输入循环,通过`iter()`函数来实现每次输入一行,并将输入的字符串拼接到`stri`变量中。然后,我们使用`isdigit()`和`isalnum()`方法来判断字符是数字还是字母,如果是空格则直接判断,其他字符则使用`else`语句进行判断。最后,我们使用`print()`函数输出统计结果。
def get_k_fold_data(k, i, X, y): assert k > 1 fold_size = X.shape[0] // k X_train, y_train = None, None for j in range(k): idx = slice(j * fold_size, (j + 1) * fold_size) X_part, y_part = X[idx,:], y[idx] if j == i: X_valid, y_valid = X_part, y_part elif X_train is None: X_train, y_train = X_part, y_part else: X_train = nd.concat(X_train, X_part, dim=0) y_train = nd.concat(y_train, y_part, dim=0) return X_train, y_train, X_valid, y_valid 对代码进行注释
# 定义一个函数,用于生成 k 折交叉验证数据集
# k: 折数
# i: 当前为第 i 折作为验证集
# X: 特征数据
# y: 标签数据
def get_k_fold_data(k, i, X, y):
# 断言 k 的值必须大于 1
assert k > 1
# 计算每一折数据集的大小
fold_size = X.shape[0] // k
# 初始化训练集和验证集的特征数据和标签数据
X_train, y_train = None, None
# 遍历每一折数据集
for j in range(k):
# 计算当前折数据集的索引范围
idx = slice(j * fold_size, (j + 1) * fold_size)
# 划分出当前折的特征数据和标签数据作为验证集
X_part, y_part = X[idx,:], y[idx]
if j == i:
# 如果当前折是验证集,则将其作为验证集
X_valid, y_valid = X_part, y_part
elif X_train is None:
# 如果当前训练集为空,则将当前折的特征数据和标签数据作为训练集
X_train, y_train = X_part, y_part
else:
# 如果当前训练集不为空,则在训练集的特征数据和标签数据后面拼接上当前折的特征数据和标签数据
X_train = nd.concat(X_train, X_part, dim=0)
y_train = nd.concat(y_train, y_part, dim=0)
# 返回训练集和验证集的特征数据和标签数据
return X_train, y_train, X_valid, y_valid
相关推荐
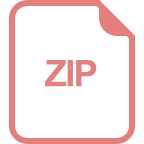
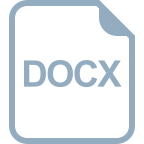
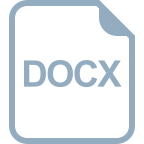











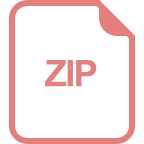
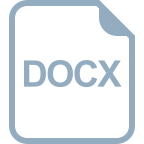