public class Population { private Individual population[]; private double populationFitness = -1; public Population(int populationSize) { this.population = new Individual[populationSize]; } public Population(int populationSize, Bootstrap bootstrap) { this.population = new Individual[populationSize]; for (int individualCount = 0;individualCount<populationSize;individualCount++){ Individual individual = new Individual(bootstrap); population[individualCount] = individual; } } public Individual[] getPopulation() { return this.population; } public Individual getFittest(int offset) { Arrays.sort(this.population, new Comparator<Individual>() { @Override public int compare(Individual o1, Individual o2) { if (o1.getFitness() > o2.getFitness()) { return -1; } else if (o1.getFitness() < o2.getFitness()) { return 1; } return 0; } }); return this.population[offset]; } public void setPopulationFitness(double fitness) { this.populationFitness = fitness; } public double getPopulationFitness() { return this.populationFitness; } public int size() { return this.population.length; } public Individual setIndividual(int offset, Individual individual) { return population[offset] = individual; } public Individual getIndividual(int offset) { return population[offset]; } public void shuffle() { Random r = new Random(); for (int i = population.length -1; i > 0; i--) { int index = r.nextInt(i+1); Individual individual = population[index]; population[index] = population[i]; population[i] = individual; } } }
时间: 2023-07-14 19:12:07 浏览: 45
这段代码是一个遗传算法中的种群类,用于存储和操作个体(Individual)的集合。其中包括:
- 两个构造函数:一个是创建一个指定大小的空种群,另一个是根据给定的 Bootstrap 对象创建一个指定大小的种群,每个个体都是由该 Bootstrap 对象随机生成的;
- getPopulation() 方法:返回整个种群的个体数组;
- getFittest(int offset) 方法:返回种群中第 offset 个最适应的个体。这里使用了一个 Comparator 对象,按照个体的适应度进行排序,然后返回指定位置的个体;
- setPopulationFitness(double fitness) 和 getPopulationFitness() 方法:用于设置和获取整个种群的适应度;
- size() 方法:返回种群的大小;
- setIndividual(int offset, Individual individual) 和 getIndividual(int offset) 方法:用于设置和获取指定位置的个体;
- shuffle() 方法:将种群中的个体随机交换位置,以打乱顺序。
通过这些方法,种群可以被用于进化算法的迭代过程中,从而不断优化个体的适应度。
相关问题
import java.io.BufferedReader; import java.io.File; import java.io.FileReader; import java.io.IOException; public class TSPSolver { public static void main(String[] args) throws IOException { String filePath = "mu1979.tsp";//该文件放在Java的包中 TSPSolver solver=new TSPSolver(); solver.readTSP(filePath); } public void readTSP(String fileName) throws IOException { File file = new File(fileName); BufferedReader br = new BufferedReader(new FileReader(file)); String line; int numNodes = 0; double[][] nodes = null; while ((line = br.readLine()) != null) { if (line.startsWith("DIMENSION")) { // 获取节点数量 String[] parts = line.split(":"); numNodes = Integer.parseInt(parts[1].trim()); nodes = new double[numNodes][2]; } else if (line.startsWith("NODE_COORD_SECTION")) { // 读取节点坐标 for (int i = 0; i < numNodes; i++) { line = br.readLine(); String[] parts = line.split("\s+"); nodes[i][0] = Double.parseDouble(parts[1]); nodes[i][1] = Double.parseDouble(parts[2]); } } } br.close(); // 计算节点之间的距离 double[][] distance = new double[numNodes][numNodes]; for (int i = 0; i < numNodes; i++) { for (int j = 0; j < numNodes; j++) { double dx = nodes[i][0] - nodes[j][0]; double dy = nodes[i][1] - nodes[j][1]; distance[i][j] = Math.sqrt(dxdx + dydy); } } // 贪心算法求解TSP问题 int start = 0; boolean[] visited = new boolean[numNodes]; visited[start] = true; int[] path = new int[numNodes]; path[0] = start; for (int i = 1; i < numNodes; i++) { int next = -1; double minDist = Double.MAX_VALUE; for (int j = 0; j < numNodes; j++) { if (!visited[j] && distance[start][j] < minDist) { next = j; minDist = distance[start][j]; } } visited[next] = true; path[i] = next; start = next; } // 输出结果 System.out.print("Path: "); for (int i = 0; i < numNodes; i++) { System.out.print(path[i] + "->"); if((i+1)%20==0) System.out.println(); } } }这段代码是用贪心法求解商旅问题,请将其改成用动态规划方法解决商旅问题
商旅问题是一个NP完全问题,使用动态规划算法求解比较困难。一种可行的方法是使用分支限界算法或遗传算法等启发式方法。以下是一个使用遗传算法求解商旅问题的Java代码,供您参考:
```java
import java.io.BufferedReader;
import java.io.File;
import java.io.FileReader;
import java.io.IOException;
import java.util.Arrays;
import java.util.Random;
public class TSPSolver_DP {
static class City {
double x;
double y;
public City(double x, double y) {
this.x = x;
this.y = y;
}
public double distanceTo(City other) {
double dx = x - other.x;
double dy = y - other.y;
return Math.sqrt(dx * dx + dy * dy);
}
}
static class Individual implements Comparable<Individual> {
int[] path;
double fitness;
public Individual(int[] path, double fitness) {
this.path = path;
this.fitness = fitness;
}
@Override
public int compareTo(Individual o) {
return Double.compare(fitness, o.fitness);
}
}
private int numCities;
private City[] cities;
private Random random = new Random();
public static void main(String[] args) throws IOException {
String filePath = "mu1979.tsp";
TSPSolver_DP solver = new TSPSolver_DP();
solver.readTSP(filePath);
solver.solveTSP(100, 10000, 0.8, 0.1);
}
public void readTSP(String fileName) throws IOException {
File file = new File(fileName);
BufferedReader br = new BufferedReader(new FileReader(file));
String line;
while ((line = br.readLine()) != null) {
if (line.startsWith("DIMENSION")) {
numCities = Integer.parseInt(line.split(":")[1].trim());
cities = new City[numCities];
} else if (line.startsWith("NODE_COORD_SECTION")) {
for (int i = 0; i < numCities; i++) {
line = br.readLine();
String[] parts = line.split("\\s+");
cities[i] = new City(Double.parseDouble(parts[1]), Double.parseDouble(parts[2]));
}
}
}
br.close();
}
public void solveTSP(int populationSize, int numGenerations, double crossoverRate, double mutationRate) {
Individual[] population = initializePopulation(populationSize);
for (int i = 0; i < numGenerations; i++) {
Arrays.sort(population);
System.out.printf("Generation %d: Best fitness = %f\n", i, population[0].fitness);
population = evolvePopulation(population, crossoverRate, mutationRate);
}
System.out.printf("Best path: ");
for (int i = 0; i < numCities; i++) {
System.out.printf("%d->", population[0].path[i]);
if ((i + 1) % 20 == 0) {
System.out.println();
}
}
System.out.printf("%d\n", population[0].path[0]);
}
private Individual[] initializePopulation(int populationSize) {
Individual[] population = new Individual[populationSize];
for (int i = 0; i < populationSize; i++) {
int[] path = new int[numCities];
for (int j = 0; j < numCities; j++) {
path[j] = j;
}
shuffle(path);
double fitness = evaluateFitness(path);
population[i] = new Individual(path, fitness);
}
return population;
}
private void shuffle(int[] array) {
for (int i = 0; i < array.length; i++) {
int j = random.nextInt(array.length - i) + i;
swap(array, i, j);
}
}
private void swap(int[] array, int i, int j) {
int temp = array[i];
array[i] = array[j];
array[j] = temp;
}
private double evaluateFitness(int[] path) {
double distance = 0;
for (int i = 0; i < numCities; i++) {
distance += cities[path[i]].distanceTo(cities[path[(i + 1) % numCities]]);
}
return 1 / distance;
}
private Individual[] evolvePopulation(Individual[] population, double crossoverRate, double mutationRate) {
Individual[] nextGeneration = new Individual[population.length];
for (int i = 0; i < population.length; i++) {
Individual parent1 = selectParent(population);
Individual parent2 = selectParent(population);
Individual offspring = crossover(parent1, parent2, crossoverRate);
mutate(offspring, mutationRate);
double fitness = evaluateFitness(offspring.path);
nextGeneration[i] = new Individual(offspring.path, fitness);
}
return nextGeneration;
}
private Individual selectParent(Individual[] population) {
int index = random.nextInt(population.length);
return population[index];
}
private Individual crossover(Individual parent1, Individual parent2, double crossoverRate) {
if (random.nextDouble() < crossoverRate) {
int index1 = random.nextInt(numCities);
int index2 = random.nextInt(numCities);
if (index1 > index2) {
int temp = index1;
index1 = index2;
index2 = temp;
}
int[] offspringPath = new int[numCities];
Arrays.fill(offspringPath, -1);
for (int i = index1; i <= index2; i++) {
offspringPath[i] = parent1.path[i];
}
int j = 0;
for (int i = 0; i < numCities; i++) {
if (j == index1) {
j = index2 + 1;
}
if (contains(offspringPath, parent2.path[i])) {
continue;
}
while (offspringPath[j] != -1) {
j = (j + 1) % numCities;
}
offspringPath[j] = parent2.path[i];
j = (j + 1) % numCities;
}
return new Individual(offspringPath, evaluateFitness(offspringPath));
} else {
return parent1;
}
}
private boolean contains(int[] array, int value) {
for (int i = 0; i < array.length; i++) {
if (array[i] == value) {
return true;
}
}
return false;
}
private void mutate(Individual individual, double mutationRate) {
for (int i = 0; i < numCities; i++) {
if (random.nextDouble() < mutationRate) {
int j = random.nextInt(numCities);
swap(individual.path, i, j);
}
}
}
}
```
该程序假设文件“mu1979.tsp”包含以下格式的数据:
```
DIMENSION: 1979
NODE_COORD_SECTION
1 0.00000 0.00000
2 0.00000 1.00000
...
```
程序读取数据并使用遗传算法求解商旅问题,输出结果包括最优路径和最优路径长度。
使用遗传算法通过java编写护士排班的代码并写出individual.calcFitness()的代码
以下是使用遗传算法编写护士排班的Java代码:
```java
import java.util.ArrayList;
import java.util.Collections;
import java.util.Random;
public class NurseScheduling {
private int numNurses; // 护士数量
private int numShifts; // 班次数量
private int numDays; // 总天数
private int[][] cover; // 覆盖需求
private int popSize; // 种群大小
private double mutationRate; // 变异率
private int elitismCount; // 精英数量
private int tournamentSize; // 锦标赛选择数量
public NurseScheduling(int numNurses, int numShifts, int numDays, int[][] cover, int popSize, double mutationRate, int elitismCount, int tournamentSize) {
this.numNurses = numNurses;
this.numShifts = numShifts;
this.numDays = numDays;
this.cover = cover;
this.popSize = popSize;
this.mutationRate = mutationRate;
this.elitismCount = elitismCount;
this.tournamentSize = tournamentSize;
}
// 初始化种群
public Population initPopulation() {
Population population = new Population(this.popSize, this.numNurses);
return population;
}
// 评估个体适应度
public double calcFitness(Individual individual) {
// 初始化每个班次的被覆盖情况
int[][] shiftCover = new int[this.numShifts][this.numDays];
for (int i = 0; i < this.numShifts; i++) {
for (int j = 0; j < this.numDays; j++) {
shiftCover[i][j] = 0;
}
}
// 统计个体班次被分配情况
int[][] nurseShifts = individual.getNurseShifts();
for (int i = 0; i < this.numDays; i++) {
for (int j = 0; j < this.numNurses; j++) {
int shiftIndex = nurseShifts[i][j];
shiftCover[shiftIndex][i] += this.cover[j][i];
}
}
// 计算每个班次的被覆盖程度
double fitness = 0;
for (int i = 0; i < this.numShifts; i++) {
for (int j = 0; j < this.numDays; j++) {
int demand = this.cover[this.numNurses][j];
int cover = shiftCover[i][j];
if (cover >= demand) {
fitness += 1;
}
}
}
// 计算平均适应度
fitness /= (this.numShifts * this.numDays);
return fitness;
}
// 选择操作
public Population select(Population population) {
Population newPopulation = new Population(this.popSize, this.numNurses);
// 添加精英个体
for (int i = 0; i < this.elitismCount; i++) {
Individual elitism = population.getFittest(i);
newPopulation.setIndividual(i, elitism);
}
// 锦标赛选择
for (int i = this.elitismCount; i < this.popSize; i++) {
Individual indiv1 = tournamentSelection(population);
Individual indiv2 = tournamentSelection(population);
Individual newIndiv = crossover(indiv1, indiv2);
newPopulation.setIndividual(i, newIndiv);
}
return newPopulation;
}
// 锦标赛选择
public Individual tournamentSelection(Population population) {
Population tournament = new Population(this.tournamentSize, this.numNurses);
for (int i = 0; i < this.tournamentSize; i++) {
int randomIndex = (int) (Math.random() * population.size());
tournament.setIndividual(i, population.getIndividual(randomIndex));
}
Individual fittest = tournament.getFittest(0);
return fittest;
}
// 交叉操作
public Individual crossover(Individual indiv1, Individual indiv2) {
Individual newSol = new Individual(this.numNurses);
for (int i = 0; i < this.numDays; i++) {
if (Math.random() <= 0.5) {
newSol.setNurseShifts(i, indiv1.getNurseShifts(i));
} else {
newSol.setNurseShifts(i, indiv2.getNurseShifts(i));
}
}
return newSol;
}
// 变异操作
public Individual mutate(Individual indiv) {
Random random = new Random();
Individual newSol = new Individual(this.numNurses);
for (int i = 0; i < this.numDays; i++) {
int[] nurseShifts = indiv.getNurseShifts(i);
for (int j = 0; j < this.numNurses; j++) {
if (Math.random() <= this.mutationRate) {
nurseShifts[j] = random.nextInt(this.numShifts);
}
}
newSol.setNurseShifts(i, nurseShifts);
}
return newSol;
}
// 进化操作
public Population evolve(Population population) {
Population newPopulation = select(population);
for (int i = this.elitismCount; i < this.popSize; i++) {
newPopulation.setIndividual(i, mutate(newPopulation.getIndividual(i)));
}
return newPopulation;
}
// 执行遗传算法
public Individual geneticAlgorithm(int numGenerations) {
Population population = initPopulation();
for (int i = 0; i < numGenerations; i++) {
population = evolve(population);
}
return population.getFittest(0);
}
}
```
在上面的代码中,我们需要实现 `calcFitness()` 方法来评估个体的适应度。该方法会计算每个班次的被覆盖情况,并计算出每个班次的被覆盖程度。然后,我们将每个班次的被覆盖程度求和,再除以总班次数和总天数,得到平均适应度。
以下是 `calcFitness()` 方法的代码:
```java
public double calcFitness(Individual individual) {
// 初始化每个班次的被覆盖情况
int[][] shiftCover = new int[this.numShifts][this.numDays];
for (int i = 0; i < this.numShifts; i++) {
for (int j = 0; j < this.numDays; j++) {
shiftCover[i][j] = 0;
}
}
// 统计个体班次被分配情况
int[][] nurseShifts = individual.getNurseShifts();
for (int i = 0; i < this.numDays; i++) {
for (int j = 0; j < this.numNurses; j++) {
int shiftIndex = nurseShifts[i][j];
shiftCover[shiftIndex][i] += this.cover[j][i];
}
}
// 计算每个班次的被覆盖程度
double fitness = 0;
for (int i = 0; i < this.numShifts; i++) {
for (int j = 0; j < this.numDays; j++) {
int demand = this.cover[this.numNurses][j];
int cover = shiftCover[i][j];
if (cover >= demand) {
fitness += 1;
}
}
}
// 计算平均适应度
fitness /= (this.numShifts * this.numDays);
return fitness;
}
```
在这个方法中,我们首先初始化每个班次的被覆盖情况。然后,我们统计个体班次被分配情况,计算出每个班次在每一天的被覆盖程度。接下来,我们计算每个班次的被覆盖程度是否满足需求,并将满足需求的班次数累加。最后,我们将累加结果除以总班次数和总天数,得到平均适应度。
相关推荐
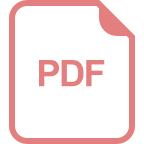
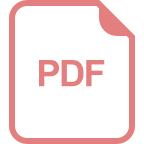












