用python实现支持向量机分类
时间: 2024-10-24 07:04:04 浏览: 15
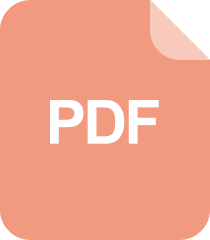
Python 支持向量机分类器的实现

在Python中,我们可以使用scikit-learn库来实现支持向量机(SVM)分类。以下是基本步骤:
1. **安装必要的库**:
首先需要安装`scikit-learn`,可以使用pip命令:`pip install scikit-learn`
2. **导入所需模块**:
导入`sklearn`中的`svm`模块和其他数据处理模块:
```python
from sklearn import svm
from sklearn.model_selection import train_test_split
from sklearn.preprocessing import StandardScaler
import numpy as np
```
3. **准备数据集**:
加载数据,划分特征(X)和标签(y),并将其划分为训练集和测试集:
```python
X = ... # 你的特征数据
y = ... # 你的目标变量
X_train, X_test, y_train, y_test = train_test_split(X, y, test_size=0.2, random_state=42)
```
4. **数据预处理**:
对数据进行标准化处理,确保所有特征在同一尺度上:
```python
scaler = StandardScaler()
X_train = scaler.fit_transform(X_train)
X_test = scaler.transform(X_test)
```
5. **创建并训练模型**:
创建svm分类器,选择合适的核函数(如线性、多项式或RBF),然后训练模型:
```python
model = svm.SVC(kernel='linear') # 或者 'poly', 'rbf'
model.fit(X_train, y_train)
```
6. **预测**:
使用训练好的模型对测试集进行预测:
```python
predictions = model.predict(X_test)
```
7. **评估模型**:
可以使用各种评价指标(如accuracy、precision、recall等)来评估模型性能:
```python
from sklearn.metrics import accuracy_score
print("Accuracy:", accuracy_score(y_test, predictions))
```
8. **(可选)调整超参数**:
如果你想优化模型性能,可以使用`GridSearchCV`或`RandomizedSearchCV`调整 SVM 的超参数。
以上就是一个简单的SVM分类器在Python中的实现流程。记得根据实际数据和需求进行适当修改。
阅读全文
相关推荐
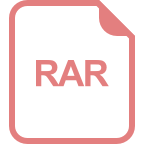
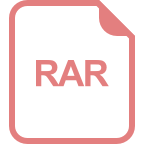


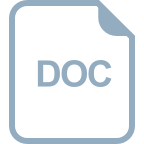

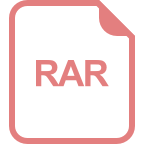
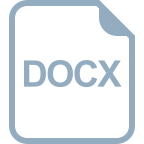
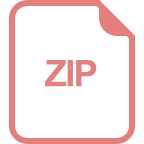
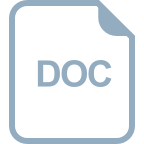
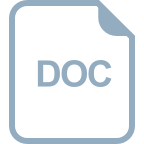
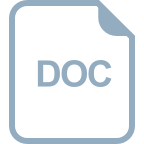
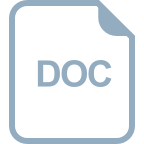




