criterion = nn.CrossEntropyLoss()有哪些属性
时间: 2023-12-18 08:04:35 浏览: 30
`nn.CrossEntropyLoss()`是 PyTorch 中的交叉熵损失函数,是一种常用于分类任务的损失函数。它是一个类,具有以下常用属性:
- `weight`: 用于对不同类别的样本赋予不同的权重,是一个张量。
- `ignore_index`: 忽略某些类别的损失计算,是一个整数。
- `reduction`: 指定损失的计算方式,可取值为`'mean'`、`'sum'`或`'none'`。
- `ignore_index`: 指定忽略某些类别的损失计算,是一个整数。
- `size_average`: 已废弃,建议使用`reduction`代替。
- `reduce`: 已废弃,建议使用`reduction`代替。
其中最常用的属性是`weight`和`reduction`。`weight`可以用于处理类别不平衡问题,`reduction`则可以指定损失的计算方式。默认情况下,`weight`和`ignore_index`都为None,`reduction`为'mean',即对每个样本的损失取平均值。
相关问题
File "E:/403/myworld/VGAE/trainTest.py", line 41, in loss_function recon_loss = dgl.losses.binary_cross_entropy(output, adj) # 重构误差 报错:AttributeError: module 'dgl' has no attribute 'losses'
根据错误提示,`dgl`模块中似乎没有名为`losses`的属性,因此无法使用`dgl.losses.binary_cross_entropy`方法。
如果您想使用DGL提供的二元交叉熵损失函数,可以使用`torch.nn.BCEWithLogitsLoss`类,该类已经包含在PyTorch中。
下面是一个使用`torch.nn.BCEWithLogitsLoss`类计算二元交叉熵损失的示例代码:
```python
import torch
import dgl
import numpy as np
with open('graph.bin', 'rb') as f:
graph_data = np.load(f, allow_pickle=True)
graph = dgl.graph((graph_data['edge_index'][0], graph_data['edge_index'][1]))
adj = (graph_data['adjacency_matrix'] != 0).astype(np.float32)
model = MyModel()
output = model(graph)
criterion = torch.nn.BCEWithLogitsLoss()
recon_loss = criterion(output.view(-1), torch.from_numpy(adj).view(-1))
```
在上述示例代码中,我们使用`torch.nn.BCEWithLogitsLoss`类计算二元交叉熵损失,并将输出`output`和邻接矩阵`adj`转换为一维向量进行计算。
需要注意的是,如果您的DGL版本较老,可能没有提供`dgl.losses`模块。如果需要使用DGL提供的损失函数,可以尝试升级DGL到最新版本。
行人属性识别训练5张图片代码
抱歉,由于行人属性识别是一个较为复杂的任务,单单训练5张图片很难得到一个准确的模型。因此,我无法提供训练5张图片的代码。
不过,我可以提供一般性的训练代码供您参考,您可以根据您的实际情况进行修改和调整。以下是使用PyTorch框架实现的一个行人属性识别模型的训练代码示例:
``` python
import torch
import torch.nn as nn
import torch.optim as optim
from torch.utils.data import DataLoader
from torchvision import transforms, datasets
# 定义数据预处理方法
transforms_train = transforms.Compose([
transforms.Resize((224, 224)), # 调整图像大小为224x224
transforms.RandomHorizontalFlip(), # 随机水平翻转
transforms.ToTensor(), # 转换为张量
transforms.Normalize([0.5, 0.5, 0.5], [0.5, 0.5, 0.5]) # 数据归一化
])
# 加载数据集
train_set = datasets.ImageFolder('path/to/train/folder', transform=transforms_train)
train_loader = DataLoader(train_set, batch_size=32, shuffle=True)
# 定义模型
class PedestrianAttributeClassifier(nn.Module):
def __init__(self):
super().__init__()
self.conv1 = nn.Conv2d(3, 64, 3, padding=1)
self.bn1 = nn.BatchNorm2d(64)
self.relu1 = nn.ReLU(inplace=True)
self.pool1 = nn.MaxPool2d(2, stride=2)
self.conv2 = nn.Conv2d(64, 128, 3, padding=1)
self.bn2 = nn.BatchNorm2d(128)
self.relu2 = nn.ReLU(inplace=True)
self.pool2 = nn.MaxPool2d(2, stride=2)
self.conv3 = nn.Conv2d(128, 256, 3, padding=1)
self.bn3 = nn.BatchNorm2d(256)
self.relu3 = nn.ReLU(inplace=True)
self.pool3 = nn.MaxPool2d(2, stride=2)
self.fc1 = nn.Linear(256 * 28 * 28, 1024)
self.bn4 = nn.BatchNorm1d(1024)
self.relu4 = nn.ReLU(inplace=True)
self.fc2 = nn.Linear(1024, 8)
def forward(self, x):
x = self.conv1(x)
x = self.bn1(x)
x = self.relu1(x)
x = self.pool1(x)
x = self.conv2(x)
x = self.bn2(x)
x = self.relu2(x)
x = self.pool2(x)
x = self.conv3(x)
x = self.bn3(x)
x = self.relu3(x)
x = self.pool3(x)
x = x.view(-1, 256 * 28 * 28)
x = self.fc1(x)
x = self.bn4(x)
x = self.relu4(x)
x = self.fc2(x)
return x
# 定义损失函数和优化器
criterion = nn.CrossEntropyLoss()
optimizer = optim.Adam(model.parameters(), lr=0.001)
# 训练模型
for epoch in range(10):
running_loss = 0.0
for i, (inputs, labels) in enumerate(train_loader):
optimizer.zero_grad()
outputs = model(inputs)
loss = criterion(outputs, labels)
loss.backward()
optimizer.step()
running_loss += loss.item()
print('Epoch %d loss: %.3f' % (epoch + 1, running_loss / len(train_loader)))
# 保存模型
torch.save(model.state_dict(), 'path/to/save/model')
```
这段代码中,我们使用了一个卷积神经网络来进行行人属性识别任务。我们使用了一个包含3个卷积层和2个全连接层的简单模型,可以根据实际情况进行调整。我们使用交叉熵作为损失函数,Adam优化器来进行参数优化。在训练过程中,我们将数据集分成了大小为32的批次进行训练,一共训练了10个epoch。最后,我们将训练好的模型保存到了本地文件中。
相关推荐
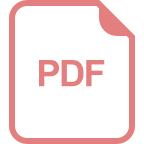
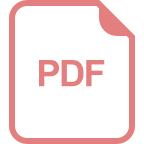
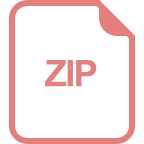












