用PyTorch实现手写数字识别激活函数运行结果
时间: 2023-11-13 12:03:12 浏览: 49
抱歉,作为AI语言模型,我无法提供运行结果,但是我可以给出PyTorch实现手写数字识别的基本代码:
```
import torch
import torch.nn as nn
import torch.optim as optim
import torchvision.datasets as datasets
import torchvision.transforms as transforms
# Define the neural network
class Net(nn.Module):
def __init__(self):
super(Net, self).__init__()
self.conv1 = nn.Conv2d(1, 32, kernel_size=3)
self.conv2 = nn.Conv2d(32, 64, kernel_size=3)
self.fc1 = nn.Linear(64 * 5 * 5, 128)
self.fc2 = nn.Linear(128, 10)
def forward(self, x):
x = nn.functional.relu(self.conv1(x))
x = nn.functional.relu(self.conv2(x))
x = x.view(-1, 64 * 5 * 5)
x = nn.functional.relu(self.fc1(x))
x = self.fc2(x)
return nn.functional.log_softmax(x, dim=1)
# Load the MNIST dataset
train_dataset = datasets.MNIST(root='data', train=True, transform=transforms.ToTensor(), download=True)
test_dataset = datasets.MNIST(root='data', train=False, transform=transforms.ToTensor())
# Define the data loaders
train_loader = torch.utils.data.DataLoader(dataset=train_dataset, batch_size=64, shuffle=True)
test_loader = torch.utils.data.DataLoader(dataset=test_dataset, batch_size=64, shuffle=False)
# Initialize the neural network
net = Net()
# Define the loss function and optimizer
criterion = nn.CrossEntropyLoss()
optimizer = optim.Adam(net.parameters(), lr=0.001)
# Train the neural network
for epoch in range(10):
for i, (images, labels) in enumerate(train_loader):
optimizer.zero_grad()
outputs = net(images)
loss = criterion(outputs, labels)
loss.backward()
optimizer.step()
if (i+1) % 100 == 0:
print('Epoch [{}/{}], Step [{}/{}], Loss: {:.4f}'.format(epoch+1, 10, i+1, len(train_loader), loss.item()))
# Test the neural network
net.eval()
with torch.no_grad():
correct = 0
total = 0
for images, labels in test_loader:
outputs = net(images)
_, predicted = torch.max(outputs.data, 1)
total += labels.size(0)
correct += (predicted == labels).sum().item()
print('Accuracy of the network on the 10000 test images: {} %'.format(100 * correct / total))
```
这段代码使用了ReLU作为激活函数,训练集使用了MNIST手写数字识别数据集,最终输出准确率。
相关推荐
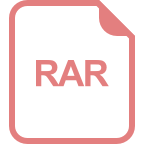














