python手写数字识别程序
时间: 2023-09-26 15:11:12 浏览: 272
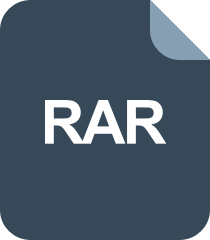
程序实现.rar_K2P_MINST_bp python_python BP_手写数字识别
这里提供一个基于Python的手写数字识别程序实现,使用的是经典的MNIST数据集进行训练和测试。
首先需要安装一些必要的库,包括numpy、tensorflow、keras等。
```python
import numpy as np
import tensorflow as tf
from tensorflow import keras
from keras.datasets import mnist
from keras.models import Sequential
from keras.layers import Dense, Dropout, Flatten, Conv2D, MaxPooling2D
from keras.utils import np_utils
```
接着,我们需要对MNIST数据集进行加载和处理。这里使用keras内置的数据集加载函数,将训练集和测试集分别存储在X_train, y_train, X_test, y_test中。同时,对数据进行预处理,将像素值从0~255的整数转化为0~1之间的浮点数,并将标签进行one-hot编码。
```python
(X_train, y_train), (X_test, y_test) = mnist.load_data()
# 将像素值从0~255的整数转化为0~1之间的浮点数
X_train = X_train.astype('float32') / 255
X_test = X_test.astype('float32') / 255
# 将标签进行one-hot编码
y_train = np_utils.to_categorical(y_train, 10)
y_test = np_utils.to_categorical(y_test, 10)
```
然后,我们可以构建一个卷积神经网络模型,包含两个卷积层和两个全连接层。其中,卷积层的作用是提取图像的特征,而全连接层则用于分类。最后,我们使用交叉熵损失函数和Adam优化器进行模型训练。
```python
model = Sequential([
Conv2D(32, (3, 3), activation='relu', input_shape=(28, 28, 1)),
Conv2D(64, (3, 3), activation='relu'),
MaxPooling2D(pool_size=(2, 2)),
Dropout(0.25),
Flatten(),
Dense(128, activation='relu'),
Dropout(0.5),
Dense(10, activation='softmax')
])
model.compile(loss='categorical_crossentropy',
optimizer='adam',
metrics=['accuracy'])
model.fit(X_train.reshape(-1, 28, 28, 1), y_train,
batch_size=128,
epochs=10,
verbose=1,
validation_data=(X_test.reshape(-1, 28, 28, 1), y_test))
score = model.evaluate(X_test.reshape(-1, 28, 28, 1), y_test, verbose=0)
print('Test loss:', score[0])
print('Test accuracy:', score[1])
```
最后,我们可以使用训练好的模型进行手写数字的识别。具体流程如下:
1. 将手写数字图像转化为28x28的黑白图像,并将像素值转化为0~1之间的浮点数;
2. 将图像输入到模型中进行预测;
3. 输出预测结果。
```python
from PIL import Image
# 读入手写数字图像并进行预处理
img = Image.open('test.png').convert('L').resize((28, 28))
x = np.array(img).reshape(1, 28, 28, 1).astype('float32') / 255
# 使用模型进行预测
y_pred = model.predict(x)
print('Predicted digit:', np.argmax(y_pred))
```
完整的代码实现如下:
```python
import numpy as np
import tensorflow as tf
from tensorflow import keras
from keras.datasets import mnist
from keras.models import Sequential
from keras.layers import Dense, Dropout, Flatten, Conv2D, MaxPooling2D
from keras.utils import np_utils
from PIL import Image
# 加载MNIST数据集
(X_train, y_train), (X_test, y_test) = mnist.load_data()
# 将像素值从0~255的整数转化为0~1之间的浮点数
X_train = X_train.astype('float32') / 255
X_test = X_test.astype('float32') / 255
# 将标签进行one-hot编码
y_train = np_utils.to_categorical(y_train, 10)
y_test = np_utils.to_categorical(y_test, 10)
# 构建模型
model = Sequential([
Conv2D(32, (3, 3), activation='relu', input_shape=(28, 28, 1)),
Conv2D(64, (3, 3), activation='relu'),
MaxPooling2D(pool_size=(2, 2)),
Dropout(0.25),
Flatten(),
Dense(128, activation='relu'),
Dropout(0.5),
Dense(10, activation='softmax')
])
model.compile(loss='categorical_crossentropy',
optimizer='adam',
metrics=['accuracy'])
model.fit(X_train.reshape(-1, 28, 28, 1), y_train,
batch_size=128,
epochs=10,
verbose=1,
validation_data=(X_test.reshape(-1, 28, 28, 1), y_test))
score = model.evaluate(X_test.reshape(-1, 28, 28, 1), y_test, verbose=0)
print('Test loss:', score[0])
print('Test accuracy:', score[1])
# 进行手写数字识别
img = Image.open('test.png').convert('L').resize((28, 28))
x = np.array(img).reshape(1, 28, 28, 1).astype('float32') / 255
y_pred = model.predict(x)
print('Predicted digit:', np.argmax(y_pred))
```
阅读全文
相关推荐
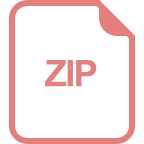
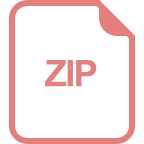
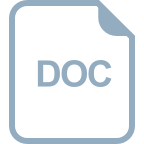
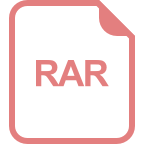
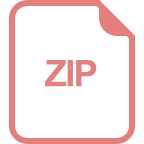
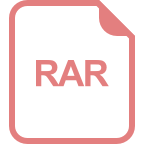
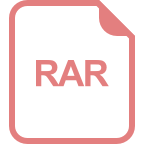
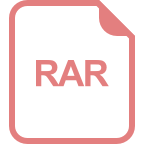
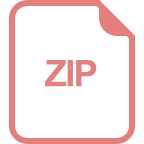
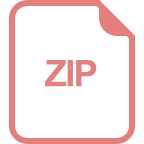
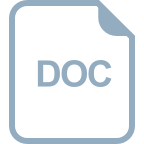
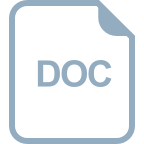
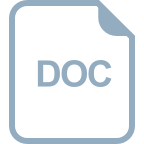
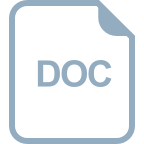
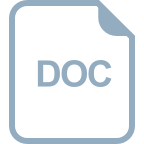
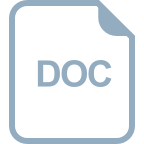
