使用pytorch编写一个attention U-NET 模型
时间: 2024-01-14 09:02:52 浏览: 92
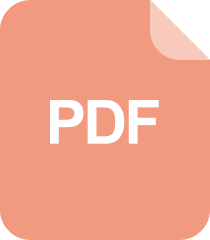
Pytorch 实现注意力机制
好的,我来解答你的问题。
首先,我们需要先了解一下Attention U-Net模型的结构。它是一种基于U-Net的图像分割模型,它利用了注意力机制来增强模型的分割能力。具体来说,Attention U-Net模型包含了编码器和解码器两个部分,其中编码器部分采用了类似U-Net的结构,而解码器部分则引入了注意力机制。
下面是使用PyTorch实现Attention U-Net模型的代码:
```python
import torch
import torch.nn as nn
class ConvBlock(nn.Module):
def __init__(self, in_channels, out_channels):
super(ConvBlock, self).__init__()
self.conv = nn.Sequential(
nn.Conv2d(in_channels, out_channels, kernel_size=3, padding=1),
nn.BatchNorm2d(out_channels),
nn.ReLU(inplace=True),
nn.Conv2d(out_channels, out_channels, kernel_size=3, padding=1),
nn.BatchNorm2d(out_channels),
nn.ReLU(inplace=True)
)
def forward(self, x):
return self.conv(x)
class UpConvBlock(nn.Module):
def __init__(self, in_channels, out_channels):
super(UpConvBlock, self).__init__()
self.up = nn.Sequential(
nn.Upsample(scale_factor=2, mode='bilinear', align_corners=True),
nn.Conv2d(in_channels, out_channels, kernel_size=1),
)
self.conv = ConvBlock(out_channels*2, out_channels)
def forward(self, x, x_down):
x_up = self.up(x)
x_concat = torch.cat([x_down, x_up], dim=1)
return self.conv(x_concat)
class AttentionBlock(nn.Module):
def __init__(self, in_channels):
super(AttentionBlock, self).__init__()
self.conv = nn.Conv2d(in_channels, in_channels//2, kernel_size=1)
self.activation = nn.Sigmoid()
def forward(self, x):
x_gap = nn.AdaptiveAvgPool2d((1, 1))(x)
x_conv = self.conv(x_gap)
x_activation = self.activation(x_conv)
x_attention = x * x_activation
return x_attention
class AttentionUNet(nn.Module):
def __init__(self, in_channels, out_channels):
super(AttentionUNet, self).__init__()
self.down1 = ConvBlock(in_channels, 64)
self.pool1 = nn.MaxPool2d(kernel_size=2, stride=2)
self.down2 = ConvBlock(64, 128)
self.pool2 = nn.MaxPool2d(kernel_size=2, stride=2)
self.down3 = ConvBlock(128, 256)
self.pool3 = nn.MaxPool2d(kernel_size=2, stride=2)
self.down4 = ConvBlock(256, 512)
self.pool4 = nn.MaxPool2d(kernel_size=2, stride=2)
self.bridge = ConvBlock(512, 1024)
self.up1 = UpConvBlock(1024, 512)
self.att1 = AttentionBlock(512)
self.up2 = UpConvBlock(512, 256)
self.att2 = AttentionBlock(256)
self.up3 = UpConvBlock(256, 128)
self.att3 = AttentionBlock(128)
self.up4 = UpConvBlock(128, 64)
self.out = nn.Conv2d(64, out_channels, kernel_size=1)
def forward(self, x):
x1 = self.down1(x)
x_pool1 = self.pool1(x1)
x2 = self.down2(x_pool1)
x_pool2 = self.pool2(x2)
x3 = self.down3(x_pool2)
x_pool3 = self.pool3(x3)
x4 = self.down4(x_pool3)
x_pool4 = self.pool4(x4)
x_bridge = self.bridge(x_pool4)
x_up1 = self.up1(x_bridge, x4)
x_att1 = self.att1(x_up1)
x_up2 = self.up2(x_att1, x3)
x_att2 = self.att2(x_up2)
x_up3 = self.up3(x_att2, x2)
x_att3 = self.att3(x_up3)
x_up4 = self.up4(x_att3, x1)
x_out = self.out(x_up4)
return x_out
```
在上面的代码中,我们定义了ConvBlock、UpConvBlock、AttentionBlock以及AttentionUNet这几个模块。ConvBlock和UpConvBlock分别用于构建编码器和解码器中的卷积块和上采样卷积块,AttentionBlock用于实现注意力机制,AttentionUNet则是整个Attention U-Net模型的实现。
在AttentionUNet中,我们首先定义了编码器部分,它由4个卷积块和4个池化层组成。接着是桥接器,它是一个卷积块,用于将编码器的输出转换为解码器的输入。解码器部分由4个上采样卷积块和4个注意力块组成,注意力块用于增强模型的分割能力。最后,我们通过一个卷积层将模型的输出转换为与分割目标相同大小的二进制掩模。
希望这个代码能够对你有所帮助!
阅读全文
相关推荐
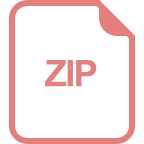
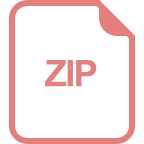
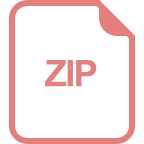
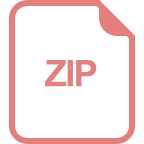
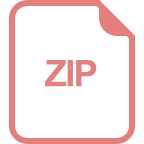
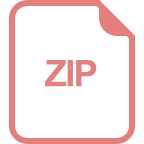
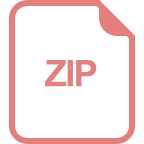
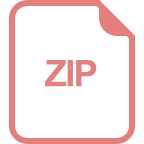
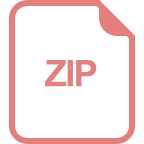
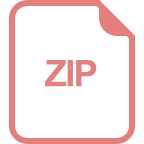
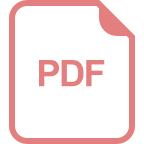
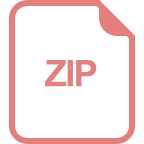
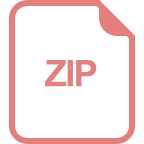
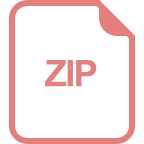