两个image 使用cross attention
时间: 2023-08-24 20:09:28 浏览: 93
对于两个图像之间的跨注意力(cross attention),可以使用注意力机制来实现。在图像处理中,可以通过将图像表示作为查询(query)和键值对(key-value pairs)的形式进行计算。
具体而言,假设有两个图像A和B。首先,将图像A和图像B分别应用卷积神经网络(CNN)并获得它们的特征表示。然后,对于图像A的每个位置上的特征向量a_i,计算其与图像B中所有位置上的特征向量的注意力分数。这可以通过计算查询向量q_i和键值对(k_j, v_j)之间的注意力分数来实现。
注意力分数可以使用点积注意力、缩放点积注意力或其他自定义的注意力机制来计算。然后,可以根据注意力分数对图像B中的特征向量进行加权求和,得到与图像A中的特征向量相关的图像B的全局表示。类似地,可以通过将查询向量与图像B中的特征向量计算注意力分数,并对图像A中的特征向量进行加权求和,得到与图像B相关的图像A的全局表示。
跨注意力机制允许两个图像之间进行信息交互和对齐,从而在各自的特征表示中捕捉到更丰富的信息。这在图像翻译、图像对齐和图像生成等任务中得到广泛应用。
相关问题
写一个文本和图片的cross attention
Cross Attention between Text and Image
Cross attention is a mechanism that allows for the interaction between different modalities, such as text and image. In this context, cross attention can be used to enhance the performance of tasks that require understanding of the relationship between textual and visual information.
In the case of text and image, cross attention can be used to align the relevant parts of the text and the image. For instance, given a caption and an image, the cross attention can be used to identify the objects in the image that correspond to the words in the caption. This can be achieved by computing a similarity score between the features of the text and the image, and using this score to weight the relevant parts of each modality.
In the figure below, we illustrate an example of cross attention between text and image. The text consists of a caption describing the scene, while the image shows the actual scene. The cross attention mechanism is used to identify the relevant parts of the image that correspond to the words in the caption. Specifically, the attention weights are computed by comparing the features of the text and the image, and are used to weight the image features.

In this example, the caption is "A man is playing guitar in a park", and the corresponding parts of the image are highlighted in red. As we can see, the man and the guitar are both correctly identified and highlighted. This allows for a more accurate understanding of the relationship between the text and the image, and can be used to improve the performance of tasks such as image captioning or visual question answering.
Overall, cross attention between text and image is a powerful mechanism that can be used to enhance the performance of tasks that require understanding of the relationship between textual and visual information. By aligning the relevant parts of the text and the image, cross attention can enable more accurate and effective processing of multimodal data.
使用pytorch实现文本和图片的cross attention
首先,我们需要定义一个自定义的CrossModalAttention层,它接收两个输入:文本和图片,然后进行交叉注意力的计算。
```python
import torch
import torch.nn as nn
class CrossModalAttention(nn.Module):
def __init__(self, text_dim, img_dim, hidden_dim):
super(CrossModalAttention, self).__init__()
self.text_dim = text_dim
self.img_dim = img_dim
self.hidden_dim = hidden_dim
self.w_text = nn.Linear(text_dim, hidden_dim)
self.w_img = nn.Linear(img_dim, hidden_dim)
self.softmax = nn.Softmax(dim=1)
def forward(self, text, img):
text_proj = self.w_text(text)
img_proj = self.w_img(img)
scores = torch.matmul(text_proj, img_proj.transpose(1, 2))
text_att = self.softmax(scores)
img_att = self.softmax(scores.transpose(1, 2))
text_weighted = torch.matmul(text_att, img)
img_weighted = torch.matmul(img_att, text)
return text_weighted, img_weighted
```
接下来,我们可以使用这个自定义层来构建一个简单的跨模态交叉注意力模型。
我们使用了一个文本嵌入层和一个图片嵌入层来将输入文本和图片转换为向量表示。然后,我们使用CrossModalAttention层计算交叉注意力,并将结果传递到后续的全连接层进行分类。
```python
import torchvision.models as models
class CrossModalModel(nn.Module):
def __init__(self, text_dim, img_dim, hidden_dim, num_classes):
super(CrossModalModel, self).__init__()
self.text_dim = text_dim
self.img_dim = img_dim
self.hidden_dim = hidden_dim
self.num_classes = num_classes
self.text_embed = nn.Embedding(text_dim, hidden_dim)
self.img_embed = models.resnet18(pretrained=True)
self.img_embed.fc = nn.Linear(512, img_dim)
self.crossmodal_att = CrossModalAttention(text_dim=hidden_dim, img_dim=img_dim, hidden_dim=hidden_dim)
self.fc = nn.Linear(hidden_dim*2, num_classes)
def forward(self, text, img):
text_embedded = self.text_embed(text)
img_embedded = self.img_embed(img)
text_weighted, img_weighted = self.crossmodal_att(text_embedded, img_embedded)
x = torch.cat([text_weighted, img_weighted], dim=1)
out = self.fc(x)
return out
```
现在我们可以使用这个模型来训练文本和图片分类任务了。我们只需要将文本和图片作为输入,然后计算交叉注意力,并将结果传递到分类器中。
```python
import torch.optim as optim
from torch.utils.data import DataLoader
# define your dataset for text and image
dataset = MyDataset(text_data, img_data, labels)
# define your dataloader
dataloader = DataLoader(dataset, batch_size=32, shuffle=True)
# define your cross-modal model
model = CrossModalModel(text_dim=100, img_dim=512, hidden_dim=256, num_classes=10)
# define your loss function and optimizer
criterion = nn.CrossEntropyLoss()
optimizer = optim.Adam(model.parameters(), lr=0.001)
# train the model
for epoch in range(num_epochs):
for text, img, labels in dataloader:
optimizer.zero_grad()
outputs = model(text, img)
loss = criterion(outputs, labels)
loss.backward()
optimizer.step()
```
相关推荐
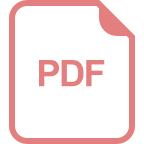
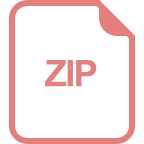












