Transformer 图注意力网络 异常检测 代码
时间: 2024-11-29 07:01:54 浏览: 4
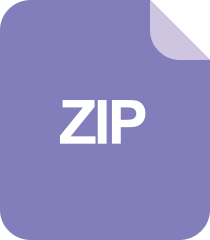
基于transformer的多模态anomaly detection(异常检测)内含数据集和教程.zip
Transformer图注意力网络(Graph Attention Networks, GAT)是一种应用于图数据的深度学习模型,尤其适用于处理节点分类、链接预测等图上结构的学习任务。GAT引入了注意力机制到传统的图神经网络中,使得模型能够对图中的边和节点进行加权,更关注于重要特征或邻域。
在异常检测方面,GAT可以通过学习正常模式下的节点特征分布,然后在新的数据点上计算其相似度得分,如果得分显著低于预期,就可能标记为异常。以下是一个简单的使用PyTorch实现GAT进行异常检测的大致框架:
```python
import torch
from torch_geometric.nn import GraphAttentionLayer
import torch.nn.functional as F
# 假设我们已经有了预处理过的图数据 (edge_index, x)
edge_index, x = ...
# 定义GAT层
num_heads = 8 # 设置注意力头的数量
dropout = 0.6 # 随机失活的比例
attention_layer = GraphAttentionLayer(x.size(-1), num_heads, dropout=dropout)
# 初始化权重
attention_layer.reset_parameters()
# 创建隐藏状态并应用注意力机制
h = x
for i in range(num_layers): # num_layers是你想要的GAT层数量
h = F.relu(attention_layer(h, edge_index)) # 注意力机制
h = F.dropout(h, p=dropout, training=self.training) # 随机失活
# 计算每个节点的异常程度分数
out = attention_layer(h, edge_index) # 最后一层的输出,可以看作是异常程度的估计
scores = F.softmax(out, dim=1) # 归一化,用于判断异常程度
# 你可以根据scores选择阈值来进行异常检测
threshold = 0.5 # 举例阈值
is_anomaly = scores[:, -1] < threshold # 标记异常节点
```
请注意,这只是一个基本示例,实际应用中可能需要对数据进行预处理、训练验证和调整超参数,如添加残差连接、使用卷积层与GAT结合等。
阅读全文
相关推荐
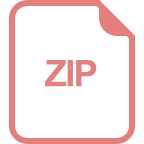
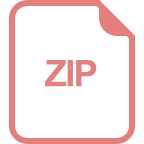
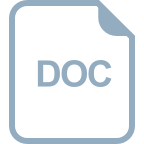
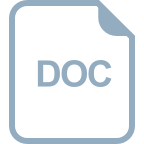
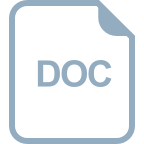
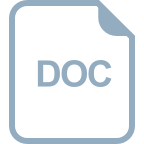

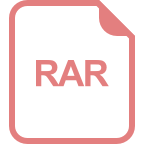
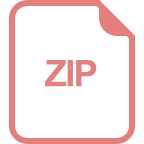
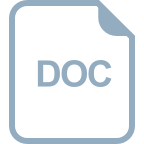
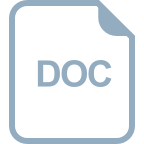
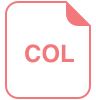
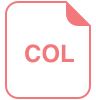
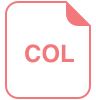
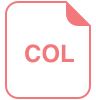
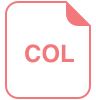
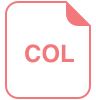
