vivado hls::mat
时间: 2023-10-01 17:09:44 浏览: 79
Vivado HLS is a high-level synthesis tool from Xilinx that allows designers to convert C, C++, or SystemC code into RTL (Register Transfer Level) code. The "mat" in "vivado hls::mat" might refer to matrix operations or matrix-related functions in Vivado HLS. It's used to perform matrix computations efficiently by leveraging the hardware capabilities of FPGA devices.
相关问题
vivado hls中hls::scalar的含义和用法
hls::scalar是一个数据类型,表示一个单一的值,用于在Vivado HLS中定义变量。在HLS中,它可以指定变量在实现时被映射到FPGA中的寄存器或内存。使用hls::scalar可以很方便地对变量进行操作和存储。
在Vivado HLS中的Test Bench的.cpp文件如何输出hls::stream<double> data_out数据流
在Vivado HLS中,可以通过在Test Bench的.cpp文件中使用C++标准库中的ofstream类来输出hls::stream<double>数据流。具体步骤如下:
1. 在Test Bench的.cpp文件中包含<fstream>头文件和<ap_axi_sdata.h>头文件:
```c++
#include <fstream>
#include <ap_axi_sdata.h>
```
2. 定义ofstream对象和hls::stream<double>对象:
```c++
ofstream output_file;
hls::stream<ap_axiu<32, 2, 5, 6> > data_in;
```
其中,ap_axiu是Vivado HLS中的数据类型,用于表示带有AXI Stream接口的数据流。
3. 打开输出文件:
```c++
output_file.open("data_out.txt");
```
4. 从hls::stream<double>对象中读取数据,并将数据输出到文件中:
```c++
ap_axiu<32, 2, 5, 6> tmp;
while(data_out.read_nb(tmp)) {
output_file << tmp.data.to_double() << endl;
}
```
其中,to_double()是ap_fixed类型的成员函数,用于将ap_fixed类型数据转换为double类型数据。
5. 关闭输出文件:
```c++
output_file.close();
```
通过以上步骤,就可以在Vivado HLS中的Test Bench的.cpp文件中输出hls::stream<double>数据流了。
相关推荐
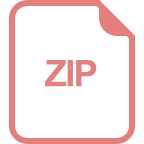
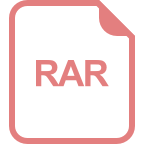












