python metrics
时间: 2024-09-17 14:08:18 浏览: 97
在Python中,"metrics"通常是指用于评估机器学习模型性能的一系列度量标准。它们帮助我们了解模型在训练数据上预测的准确性和稳定性。Python库如`scikit-learn`、`tensorflow`和`keras`等提供了丰富的内置指标:
1. **Accuracy**(准确率):最基础的衡量分类任务正确率的指标,即正确预测的比例。
2. **Precision**(精确率):对于分类任务,表示真正例占所有预测为正例的比例,强调了较少误报的重要性。
3. **Recall**(召回率):也叫查准率,表示真正例占所有实际正例的比例,关注了较少漏报的情况。
4. **F1 Score**:综合考虑precision和recall的加权平均值,用于平衡这两个指标。
5. **AUC-ROC**(Area Under the Receiver Operating Characteristic Curve):用于二分类问题,评估模型在不同阈值下的区分能力。
6. **Mean Squared Error (MSE)**:适用于回归任务,衡量预测值与真实值之间差的平方的平均。
7. **R^2 Score**:回归模型常用的评价指标,表示解释变量对目标变量变化的贡献程度。
使用这些metric时,通常会结合交叉验证(Cross-validation)来得到更稳健的评估结果。如果你需要使用特定的metric,可以查阅相应的库文档,例如`sklearn.metrics`中的函数。
相关问题
python中metrics
在Python中,metrics(度量)是用来衡量模型性能的指标。常见的机器学习和深度学习任务中,我们经常使用各种度量来评估模型的准确性、精确度、召回率等性能指标。下面是一些常见的Python库和函数,用于计算不同类型的度量指标:
1. scikit-learn库:scikit-learn是一个常用的机器学习库,提供了丰富的度量函数来评估分类、回归和聚类模型的性能。例如,可以使用`accuracy_score`函数计算分类模型的准确率,使用`precision_score`和`recall_score`函数计算分类模型的精确度和召回率。
2. TensorFlow库:TensorFlow是一个流行的深度学习库,提供了各种度量函数来评估深度学习模型的性能。例如,可以使用`tf.keras.metrics.Accuracy`类来计算分类模型的准确率,使用`tf.keras.metrics.Precision`和`tf.keras.metrics.Recall`类来计算分类模型的精确度和召回率。
3. PyTorch库:PyTorch是另一个流行的深度学习库,也提供了各种度量函数来评估深度学习模型的性能。例如,可以使用`torchmetrics.Accuracy`类来计算分类模型的准确率,使用`torchmetrics.Precision`和`torchmetrics.Recall`类来计算分类模型的精确度和召回率。
下面是一个示例代码,演示了如何使用scikit-learn库计算分类模型的准确率和精确度:
```python
from sklearn.metrics import accuracy_score, precision_score
# 实际标签
y_true = [0, 1, 1, 0, 1, 0]
# 预测标签
y_pred = [0, 1, 0, 0, 1, 1]
# 计算准确率
accuracy = accuracy_score(y_true, y_pred)
print("Accuracy: ", accuracy)
# 计算精确度
precision = precision_score(y_true, y_pred)
print("Precision: ", precision)
```
metrics python
Kubernetes (k8s) provides a robust monitoring and metrics collection system called the Metrics API. To interact with the Metrics API using Python, you can use the `kubernetes` package. Here's an example of how you can retrieve metrics from a Kubernetes cluster using Python:
```python
from kubernetes import client, config
# Load Kubernetes configuration
config.load_kube_config()
# Create an API client
api_client = client.ApiClient()
# Create a CoreV1Api instance
v1 = client.CoreV1Api(api_client)
# Retrieve metrics for all pods in a namespace
namespace = "your-namespace"
metrics = v1.list_namespaced_pod_metric(namespace=namespace)
# Iterate over the metrics and print them
for metric in metrics.items:
print(metric)
```
This code snippet demonstrates how to retrieve metrics for all pods within a specific namespace. You can customize it further based on your requirements.
To use this code, make sure you have the `kubernetes` package installed (`pip install kubernetes`) and the appropriate access to interact with the Kubernetes cluster.
阅读全文
相关推荐
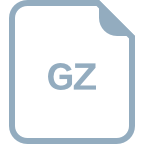
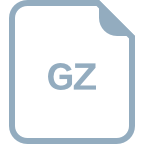




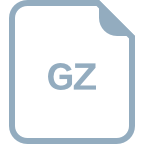

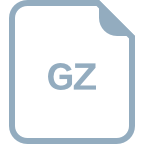







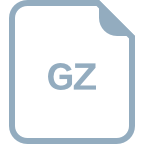