pd.read_excel(converts)
时间: 2023-10-29 09:52:21 浏览: 99
pd.read_excel() 是 pandas 库中的一个函数,用于读取 Excel 文件并将其转换为 DataFrame 对象。
在函数中,converts 参数是一个可选的参数,用于指定数据类型的转换规则。如果不传入 converts 参数,则默认情况下将会使用 Excel 文件中列的数据类型。converts 参数应该是一个字典,其中键表示要进行数据类型转换的列名,值表示要转换的数据类型。例如,如果要将 'column1' 列的数据类型转换为字符串,可以使用 converts={'column1': str} 。
示例代码如下:
```python
import pandas as pd
# 读取 Excel 文件并指定数据类型转换规则
df = pd.read_excel('file.xlsx', converts={'column1': str, 'column2': int})
# 打印 DataFrame 对象
print(df)
```
请注意,converts 参数在较新版本的 pandas 中已经被废弃,推荐使用 dtype 参数来指定数据类型转换规则。
相关问题
解释import pandas as pd df = pd.read_csv('S12_wearther_central_park.csv') df['DATE'] = pd.to_datetime(df['DATE']) df.set_index('DATE', inplace=True) x = input() year_df = df.loc[str(x), ['PRCP', 'TMIN', 'TMAX']] rainy_days = year_df[year_df['PRCP'] > 1.3] print(rainy_days)
Certainly! Let me explain what each line of the code does:
```python
import pandas as pd
```
This line imports the Pandas library and assigns it the alias `pd`, which is commonly used in Python code.
```python
df = pd.read_csv('S12_wearther_central_park.csv')
```
This line reads the CSV file 'S12_wearther_central_park.csv' into a Pandas DataFrame called `df`. The data in the CSV file is assumed to be comma-separated.
```python
df['DATE'] = pd.to_datetime(df['DATE'])
```
This line converts the 'DATE' column of the DataFrame to a Pandas datetime object. This allows us to perform various operations on the date, such as filtering by year or month.
```python
df.set_index('DATE', inplace=True)
```
This line sets the 'DATE' column as the index of the DataFrame. This is useful for quickly accessing data based on the date.
```python
x = input()
```
This line prompts the user for input and assigns it to the variable `x`. This input is assumed to be a year in the format of a string, e.g. '2010'.
```python
year_df = df.loc[str(x), ['PRCP', 'TMIN', 'TMAX']]
```
This line creates a new DataFrame called `year_df` that contains the precipitation, minimum temperature, and maximum temperature data for the year specified by the user input. The `.loc` method is used to slice the DataFrame by the year, and the square brackets are used to select the columns of interest.
```python
rainy_days = year_df[year_df['PRCP'] > 1.3]
```
This line creates a new DataFrame called `rainy_days` that contains only the rows of `year_df` where the precipitation value is greater than 1.3 inches. This is done by using boolean indexing and comparing the 'PRCP' column to the value 1.3.
```python
print(rainy_days)
```
This line prints the `rainy_days` DataFrame to the console. This DataFrame contains the date, precipitation, minimum temperature, and maximum temperature for the days where precipitation was greater than 1.3 inches.
pd.get_dummies
pd.get_dummies is a Python function from the pandas library that is used to create dummy variables from categorical data. It creates a new column for each unique category of a categorical variable, and assigns a value of 1 or 0 to each row depending on whether that row belongs to that category or not. This is useful for machine learning algorithms that require numerical input, as it converts non-numerical data into a numerical format.
For example, if we have a dataset with a categorical variable "color" that has three categories: red, green, and blue, pd.get_dummies will create three new columns in the dataset called "color_red", "color_green", and "color_blue". Each row will have a value of 1 in the column that corresponds to its color, and 0 in the other two columns.
The syntax for pd.get_dummies is:
```
pd.get_dummies(data, columns=None, prefix=None, prefix_sep='_', dummy_na=False, drop_first=False)
```
- data: the input pandas DataFrame or Series
- columns: the name or list of names of the columns to encode. If not specified, all non-numerical columns will be encoded.
- prefix: the prefix to add to the column names of the new dummy variables
- prefix_sep: the separator to use between the prefix and the original column name
- dummy_na: whether to create an additional column for missing values. If True, a column called "column_name_nan" will be created for each column with missing values.
- drop_first: whether to drop the first column of each set of dummy variables to avoid multicollinearity. If True, the first column will be dropped.
阅读全文
相关推荐









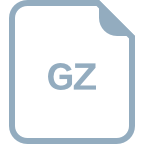






