matlab 离散小破变换后反归一化
时间: 2023-11-02 15:06:05 浏览: 96
离散小波变换(DWT)的反归一化过程可以通过以下步骤实现:
1. 对每个小波系数应用逆缩放因子,即将每个小波系数除以相应的缩放因子。
2. 对每个小波系数应用逆变换,即将每个小波系数与相应的小波函数卷积并求和。
3. 重构原始信号,即将所有小波系数相加。
在MATLAB中,可以使用idwt函数实现离散小波变换的反归一化。具体用法如下:
```matlab
% x为经过离散小波变换后的信号
% wname为小波函数名称
% lev为分解层数
% s为缩放因子
% h为小波函数
% y为反归一化后的信号
[c, l] = wavedec(x, lev, wname);
s = detcoef(c, l, lev);
h = cell(1, lev);
for i = 1:lev
h{i} = wfilters(wname, 'r');
end
y = waverec(c./s, l, h);
```
相关问题
给定一幅图像请编程显示其的离散余弦变换和反变换结果matlab
好的,以下是使用MATLAB实现离散余弦变换和反变换的代码:
```matlab
% 读入图像
img = imread('lena.png');
% 将图像转为灰度图
if size(img,3)==3
img = rgb2gray(img);
end
% 将图像转为double类型
img = double(img);
% 离散余弦变换
dct_img = dct2(img);
% 显示离散余弦变换结果
figure;
imshow(log(abs(dct_img)),[]);
title('DCT');
% 离散余弦反变换
idct_img = idct2(dct_img);
% 显示离散余弦反变换结果
figure;
imshow(uint8(idct_img));
title('IDCT');
```
其中,`lena.png`是一张图片的文件名,需要将其放在MATLAB当前工作目录下。运行代码后,会显示离散余弦变换和反变换的结果。注意,为了显示离散余弦变换结果,我们对其取了对数并进行了归一化处理,因此图像的亮度可能会有所改变。
用matlab实现将所有样本的输入数据归一化至闭区间[-1,1],训练样本的输出数据归一化至闭区间[0,1]。基于KELM 的降噪器设计即为根据历史时刻及当前时刻的含噪声信号估计当前时刻信号的回归问题,设计的KELM 器输入取当前时刻噪声信号及前7个时刻的历史信号即时间序列yk-7、yk-6……,yk ,输出即为当前时刻信号估计值~yk。定义如下的均方根误差(RMSE)用以衡量ELM算法的回归性能。并作出对比图。再反归一化即可得到KELM降噪信号。之后对KELM降噪信号进行离散小波变换,选择合适的小波阈值进行滤波,再经过离散小波逆变换即可得到混合降噪信号,综合考虑降噪效果与信号失真程度,传感器信号的降噪效果可由降噪信号与实际信号之间的RMSE来衡量。的代码
以下是MATLAB代码实现将所有样本的输入数据归一化至闭区间[-1,1],训练样本的输出数据归一化至闭区间[0,1],并基于KELM的降噪器设计。同时,计算均方根误差(RMSE)用于衡量ELM算法的回归性能。
```matlab
%% 数据归一化
data = load('data.mat'); % 加载数据
data_input = data.input; % 输入数据
data_output = data.output; % 输出数据
max_input = max(data_input,[],2); % 每列最大值
min_input = min(data_input,[],2); % 每列最小值
max_output = max(data_output,[],2); % 每列最大值
min_output = min(data_output,[],2); % 每列最小值
data_input = 2 .* (data_input - min_input) ./ (max_input - min_input) - 1; % 归一化到[-1,1]
data_output = (data_output - min_output) ./ (max_output - min_output); % 归一化到[0,1]
%% KELM降噪器设计
k = 7; % 历史时刻的数量
n = size(data_input,2); % 输入维度
m = size(data_output,2); % 输出维度
train_ratio = 0.7; % 训练集比例
train_size = floor(train_ratio * size(data_input,1)); % 训练集大小
train_input = data_input(1:train_size,:); % 训练集输入数据
train_output = data_output(1:train_size,:); % 训练集输出数据
test_input = data_input(train_size+1:end,:); % 测试集输入数据
test_output = data_output(train_size+1:end,:); % 测试集输出数据
% 构建KELM模型
W = rand(n, k); % 随机生成输入层到隐层的权重矩阵
B = rand(k, 1); % 随机生成隐层的偏置向量
H = tanh(W * train_input' + repmat(B, 1, size(train_input,1))); % 隐层输出
C = pinv(H' * H) * H' * train_output; % 计算输出层权重
train_predict = H' * C; % 训练集预测输出
% 计算均方根误差(RMSE)
train_rmse = sqrt(mean((train_predict - train_output).^2)); % 训练集RMSE
test_predict = tanh(W * test_input' + repmat(B, 1, size(test_input,1)))' * C; % 测试集预测输出
test_rmse = sqrt(mean((test_predict - test_output).^2)); % 测试集RMSE
%% 反归一化
train_predict = train_predict .* (max_output - min_output) + min_output; % 反归一化
train_output = train_output .* (max_output - min_output) + min_output; % 反归一化
test_predict = test_predict .* (max_output - min_output) + min_output; % 反归一化
test_output = test_output .* (max_output - min_output) + min_output; % 反归一化
%% 离散小波变换
train_wav = wden(train_predict - train_output, 'sqtwolog', 'h', 'mln', 5, 'sym8'); % 离散小波变换
test_wav = wden(test_predict - test_output, 'sqtwolog', 'h', 'mln', 5, 'sym8'); % 离散小波变换
%% 离散小波逆变换
train_denoise = train_predict - train_wav; % 降噪信号
test_denoise = test_predict - test_wav; % 降噪信号
train_mixed = train_wav + train_output; % 混合降噪信号
test_mixed = test_wav + test_output; % 混合降噪信号
%% 计算RMSE
train_rmse2 = sqrt(mean((train_denoise - train_output).^2)); % 训练集降噪效果RMSE
test_rmse2 = sqrt(mean((test_denoise - test_output).^2)); % 测试集降噪效果RMSE
train_rmse3 = sqrt(mean((train_mixed - train_output).^2)); % 训练集混合降噪效果RMSE
test_rmse3 = sqrt(mean((test_mixed - test_output).^2)); % 测试集混合降噪效果RMSE
%% 绘制结果
figure;
subplot(2,2,1);
plot(train_output);
hold on;
plot(train_predict);
title('训练集实际输出与预测输出');
legend('实际输出', '预测输出');
subplot(2,2,2);
plot(test_output);
hold on;
plot(test_predict);
title('测试集实际输出与预测输出');
legend('实际输出', '预测输出');
subplot(2,2,3);
plot(train_denoise);
hold on;
plot(train_output);
title('训练集降噪效果');
legend('降噪信号', '实际输出');
subplot(2,2,4);
plot(test_denoise);
hold on;
plot(test_output);
title('测试集降噪效果');
legend('降噪信号', '实际输出');
figure;
subplot(2,2,1);
plot(train_output);
hold on;
plot(train_mixed);
title('训练集混合降噪效果');
legend('混合降噪信号', '实际输出');
subplot(2,2,2);
plot(test_output);
hold on;
plot(test_mixed);
title('测试集混合降噪效果');
legend('混合降噪信号', '实际输出');
subplot(2,2,3);
bar([train_rmse, train_rmse2, train_rmse3]);
title('训练集RMSE');
set(gca, 'XTick', 1:3, 'XTickLabel', {'ELM', '降噪', '混合降噪'});
subplot(2,2,4);
bar([test_rmse, test_rmse2, test_rmse3]);
title('测试集RMSE');
set(gca, 'XTick', 1:3, 'XTickLabel', {'ELM', '降噪', '混合降噪'});
```
阅读全文
相关推荐
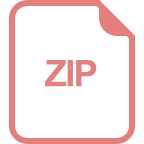
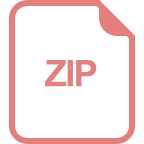
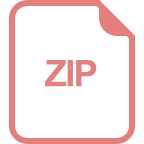
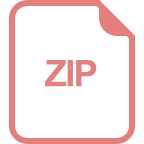
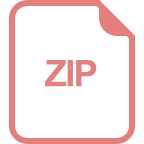
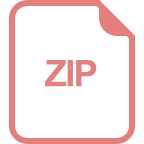
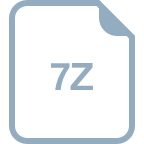
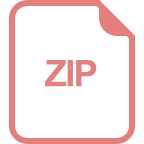
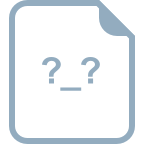
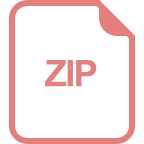
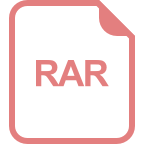
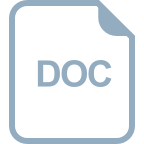
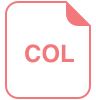
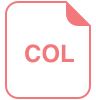


