Multi-Stage Algorithms: A Comprehensive Survey
时间: 2024-04-22 13:26:30 浏览: 24
"Multi-Stage Algorithms: A Comprehensive Survey"(2017)是一篇综合调查多阶段算法的文章。该文章由Y. Zhang, Y. Han, L. Liu等人撰写,并提供了对多阶段算法的定义、分类、性质、应用领域和未来研究方向的综述。
文章首先介绍了多阶段算法的基本概念和定义。它解释了多阶段算法是一种将问题分解为多个阶段并分别解决的方法,每个阶段的解决方案都依赖于前一个阶段的结果。
接下来,文章对多阶段算法进行了分类。它将多阶段算法分为序列决策问题、多层次决策问题和分布式决策问题等不同类型,针对每种类型讨论了其特点和应用。
然后,文章回顾了多阶段算法的性质。它详细探讨了多阶段算法的可行性和最优性等性质,并说明了在不同约束条件下多阶段算法的优化目标和限制条件。
文章接着讨论了多阶段算法在各个领域中的应用。它提到了多阶段算法在机器学习、数据挖掘、网络优化和资源分配等领域的应用案例,并强调了多阶段算法在处理复杂问题和大规模数据时的优势。
最后,文章总结了目前多阶段算法研究的主要趋势和未来的研究方向。它提出了一些未解决的问题,如多阶段算法的实时性、稳定性和鲁棒性等,并呼吁进一步研究多阶段算法的性能分析和优化方法。
"Multi-Stage Algorithms: A Comprehensive Survey"这篇文章提供了对多阶段算法的全面调查,涵盖了其定义、分类、性质、应用领域和未来研究方向等方面。它为研究人员和从业者提供了对多阶段算法的深入了解和指导。
相关问题
flocking for multi-agent dynamic systems:algorithms and theory
多智能体动态系统是指由许多可以相互交互的个体所组成的集合。在这样的系统中,个体之间的互动和协同行为对于系统的整体性能有着重要的影响。其中,群集行为(flocking)被广泛应用于多智能体动态系统中,用于描述个体之间的协同行为。在这种行为中,个体之间会相互靠近,保持一定的距离,并朝着相同的方向运动。
针对群集行为这一问题,学术界提出了许多算法和理论,例如经典的Reynolds算法、Vicsek模型等等。这些算法和模型基本上都是通过设计适当的控制规律来产生群集行为,同时满足一些限制条件,如避免碰撞等。同时,理论上也提出了一些关于这些算法的性质和分析,例如收敛性、稳定性等。然而,由于多智能体动态系统的非线性和复杂性,相应的算法和理论仍存在许多未解决的问题和挑战。
总体来看,群集行为在多智能体动态系统中的应用具有重要的意义,不仅可以用于模拟真实世界中的许多复杂现象,如鸟群、鱼群等,还可以应用于智能交通、机器人协作等领域,有着广泛的应用前景。因此,进一步深入研究群集行为的算法和理论,对于推动多智能体动态系统的发展具有重要的作用。
Write a C program that implements the following disk-scheduling algorithms: a. FCFS b. SSTF c. SCAN d. C-SCAN e. LOOK f. C-LOOK Your program will service a disk with 5,000 cylinders numbered 0 to 4,999. The program will generate a random series of 1,000 cylinder requests and service them according to each of the algorithms listed above. The program will be passed the initial position of the disk head (as a parameter on the command line) and report the total amount of head movement required by each algorithm.
Sure, here's a sample C program that implements the disk-scheduling algorithms you mentioned:
```c
#include <stdio.h>
#include <stdlib.h>
#include <stdbool.h>
#define CYLINDERS 5000
#define REQUESTS 1000
// Struct to represent a disk request
typedef struct {
int cylinder;
bool visited;
} request;
// Function to generate random cylinder requests
void generateRequests(request* queue) {
for (int i = 0; i < REQUESTS; i++) {
queue[i].cylinder = rand() % CYLINDERS;
queue[i].visited = false;
}
}
// FCFS algorithm
int fcfs(request* queue, int head) {
int totalMovement = 0;
for (int i = 0; i < REQUESTS; i++) {
totalMovement += abs(head - queue[i].cylinder);
head = queue[i].cylinder;
}
return totalMovement;
}
// SSTF algorithm
int sstf(request* queue, int head) {
int totalMovement = 0;
for (int i = 0; i < REQUESTS; i++) {
int minDistance = CYLINDERS;
int minIndex = -1;
for (int j = 0; j < REQUESTS; j++) {
if (!queue[j].visited) {
int distance = abs(head - queue[j].cylinder);
if (distance < minDistance) {
minDistance = distance;
minIndex = j;
}
}
}
if (minIndex != -1) {
totalMovement += minDistance;
head = queue[minIndex].cylinder;
queue[minIndex].visited = true;
}
}
return totalMovement;
}
// SCAN algorithm
int scan(request* queue, int head) {
int totalMovement = 0;
int direction = 1; // 1 for up, -1 for down
int currentIndex = -1;
for (int i = 0; i < REQUESTS; i++) {
if (queue[i].cylinder == head) {
currentIndex = i;
break;
}
}
if (currentIndex == -1) {
// Insert head into queue
for (int i = 0; i < REQUESTS; i++) {
if (head < queue[i].cylinder) {
for (int j = REQUESTS - 1; j > i; j--) {
queue[j] = queue[j-1];
}
queue[i].cylinder = head;
queue[i].visited = true;
currentIndex = i;
break;
}
}
}
for (int i = currentIndex; i >= 0 && i < REQUESTS; i += direction) {
if (!queue[i].visited) {
totalMovement += abs(head - queue[i].cylinder);
head = queue[i].cylinder;
queue[i].visited = true;
}
if (i == 0 || i == REQUESTS - 1) {
direction = -direction;
}
}
return totalMovement;
}
// C-SCAN algorithm
int cscan(request* queue, int head) {
int totalMovement = 0;
int currentIndex = -1;
for (int i = 0; i < REQUESTS; i++) {
if (queue[i].cylinder == head) {
currentIndex = i;
break;
}
}
if (currentIndex == -1) {
// Insert head into queue
for (int i = 0; i < REQUESTS; i++) {
if (head < queue[i].cylinder) {
for (int j = REQUESTS - 1; j > i; j--) {
queue[j] = queue[j-1];
}
queue[i].cylinder = head;
queue[i].visited = true;
currentIndex = i;
break;
}
}
}
// Service requests in one direction
for (int i = currentIndex; i >= 0; i--) {
if (!queue[i].visited) {
totalMovement += abs(head - queue[i].cylinder);
head = queue[i].cylinder;
queue[i].visited = true;
}
}
totalMovement += head; // Head movement to go to the end
head = 0;
// Service requests in the other direction
for (int i = REQUESTS - 1; i > currentIndex; i--) {
if (!queue[i].visited) {
totalMovement += abs(head - queue[i].cylinder);
head = queue[i].cylinder;
queue[i].visited = true;
}
}
return totalMovement;
}
// LOOK algorithm
int look(request* queue, int head) {
int totalMovement = 0;
int direction = 1; // 1 for up, -1 for down
int currentIndex = -1;
for (int i = 0; i < REQUESTS; i++) {
if (queue[i].cylinder == head) {
currentIndex = i;
break;
}
}
if (currentIndex == -1) {
// Insert head into queue
for (int i = 0; i < REQUESTS; i++) {
if (head < queue[i].cylinder) {
for (int j = REQUESTS - 1; j > i; j--) {
queue[j] = queue[j-1];
}
queue[i].cylinder = head;
queue[i].visited = true;
currentIndex = i;
break;
}
}
}
for (int i = currentIndex; i >= 0 && i < REQUESTS; i += direction) {
if (!queue[i].visited) {
totalMovement += abs(head - queue[i].cylinder);
head = queue[i].cylinder;
queue[i].visited = true;
}
if (i == 0 || i == REQUESTS - 1) {
direction = -direction;
}
}
return totalMovement;
}
// C-LOOK algorithm
int clook(request* queue, int head) {
int totalMovement = 0;
int currentIndex = -1;
for (int i = 0; i < REQUESTS; i++) {
if (queue[i].cylinder == head) {
currentIndex = i;
break;
}
}
if (currentIndex == -1) {
// Insert head into queue
for (int i = 0; i < REQUESTS; i++) {
if (head < queue[i].cylinder) {
for (int j = REQUESTS - 1; j > i; j--) {
queue[j] = queue[j-1];
}
queue[i].cylinder = head;
queue[i].visited = true;
currentIndex = i;
break;
}
}
}
// Service requests in one direction
for (int i = currentIndex; i >= 0; i--) {
if (!queue[i].visited) {
totalMovement += abs(head - queue[i].cylinder);
head = queue[i].cylinder;
queue[i].visited = true;
}
}
// Service requests in the other direction
for (int i = REQUESTS - 1; i > currentIndex; i--) {
if (!queue[i].visited) {
totalMovement += abs(head - queue[i].cylinder);
head = queue[i].cylinder;
queue[i].visited = true;
}
}
return totalMovement;
}
int main(int argc, char* argv[]) {
if (argc < 2) {
printf("Usage: %s <initial position>\n", argv[0]);
return 1;
}
int initial = atoi(argv[1]);
if (initial < 0 || initial >= CYLINDERS) {
printf("Initial position must be between 0 and %d\n", CYLINDERS - 1);
return 1;
}
request queue[REQUESTS];
generateRequests(queue);
printf("FCFS: %d\n", fcfs(queue, initial));
for (int i = 0; i < REQUESTS; i++) {
queue[i].visited = false;
}
printf("SSTF: %d\n", sstf(queue, initial));
for (int i = 0; i < REQUESTS; i++) {
queue[i].visited = false;
}
printf("SCAN: %d\n", scan(queue, initial));
for (int i = 0; i < REQUESTS; i++) {
queue[i].visited = false;
}
printf("C-SCAN: %d\n", cscan(queue, initial));
for (int i = 0; i < REQUESTS; i++) {
queue[i].visited = false;
}
printf("LOOK: %d\n", look(queue, initial));
for (int i = 0; i < REQUESTS; i++) {
queue[i].visited = false;
}
printf("C-LOOK: %d\n", clook(queue, initial));
return 0;
}
```
To compile and run the program, you can use the following commands:
```sh
$ gcc disk-scheduling.c -o disk-scheduling
$ ./disk-scheduling <initial position>
```
Replace `<initial position>` with the desired starting position of the disk head. The program will generate a random series of 1,000 cylinder requests and service them according to each of the algorithms listed above, reporting the total amount of head movement required by each algorithm.
相关推荐
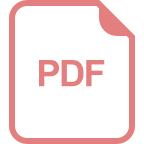
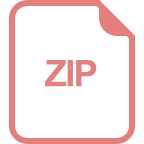
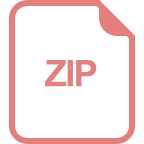













