yolov8推理结果显示
时间: 2024-03-23 19:35:29 浏览: 410
YOLOv8是一种目标检测算法,它是YOLO(You Only Look Once)系列算法的最新版本。YOLOv8通过将图像分成网格,并在每个网格中预测边界框和类别来实现目标检测。推理结果显示了检测到的目标的位置和类别。
推理结果通常以边界框的形式呈现,每个边界框包含目标的位置信息和类别信息。位置信息由边界框的左上角和右下角坐标表示,类别信息表示检测到的目标属于哪个类别。
除了位置和类别信息,推理结果还可以包含置信度或得分,用于表示算法对检测结果的置信程度。较高的置信度意味着算法更加确信该目标存在。
相关问题
yolov8推理代码
这里是YOLOv4的推理代码示例,YOLOv8与YOLOv4的实现类似,你可以根据YOLOv8的网络结构进行相应的调整。
```python
import cv2
import numpy as np
import time
# 加载模型
net = cv2.dnn.readNetFromDarknet('yolov4.cfg', 'yolov4.weights')
# 获取输出层
layer_names = net.getLayerNames()
output_layers = [layer_names[i[0] - 1] for i in net.getUnconnectedOutLayers()]
# 加载类别标签
with open('coco.names', 'r') as f:
classes = [line.strip() for line in f.readlines()]
# 加载图像
image = cv2.imread('image.jpg')
height, width, channels = image.shape
# 图像预处理
blob = cv2.dnn.blobFromImage(image, 1 / 255.0, (416, 416), swapRB=True, crop=False)
net.setInput(blob)
# 前向传播
start_time = time.time()
outputs = net.forward(output_layers)
end_time = time.time()
# 解析输出结果
class_ids = []
confidences = []
boxes = []
for output in outputs:
for detection in output:
scores = detection[5:]
class_id = np.argmax(scores)
confidence = scores[class_id]
if confidence > 0.5:
center_x = int(detection[0] * width)
center_y = int(detection[1] * height)
w = int(detection[2] * width)
h = int(detection[3] * height)
x = int(center_x - w / 2)
y = int(center_y - h / 2)
class_ids.append(class_id)
confidences.append(float(confidence))
boxes.append([x, y, w, h])
# 非最大抑制
indexes = cv2.dnn.NMSBoxes(boxes, confidences, 0.5, 0.4)
# 绘制边界框和标签
font = cv2.FONT_HERSHEY_PLAIN
colors = np.random.uniform(0, 255, size=(len(classes), 3))
for i in range(len(boxes)):
if i in indexes:
x, y, w, h = boxes[i]
label = str(classes[class_ids[i]])
confidence = confidences[i]
color = colors[class_ids[i]]
cv2.rectangle(image, (x, y), (x + w, y + h), color, 2)
cv2.putText(image, f'{label} {confidence:.2f}', (x, y - 5), font, 1, color, 2)
# 显示结果
cv2.imshow('Image', image)
cv2.waitKey(0)
cv2.destroyAllWindows()
# 打印推理时间
print(f'Inference time: {end_time - start_time}s')
```
请确保你已下载YOLOv8的权重文件(`yolov8.weights`),YOLOv8的配置文件(`yolov8.cfg`)和类别标签文件(`coco.names`),并将它们放在相应的路径下。同时,将待检测的图像命名为`image.jpg`并放在同一目录下。
以上代码会读取图像并进行目标检测,将检测结果显示在图像上,并输出推理时间。你可以根据实际情况对代码进行调整和优化。
如何用opencv C++解析YOLOv5推理输出的结果
要使用OpenCV C++解析Yolov5的推理输出结果,需要遵循以下步骤:
1.读取推理输出文件:Yolov5的推理输出通常是保存在一个文本文件中。使用C++的文件操作函数,可以读取该文件并将其存储到内存中。
2.解析输出信息:将存储在内存中的推理输出信息解析为一个包含所有检测目标信息的结构体或向量。每个目标的信息包括目标边界框的位置和大小、目标类别、以及目标置信度等信息。
3.绘制检测结果:使用OpenCV的绘图函数,将解析出的每个目标的信息在图像或视频中绘制出来。绘制的内容包括目标边界框的矩形框、目标类别标签、以及置信度等信息。
以下是一个简单的代码示例,展示了如何使用OpenCV C++解析Yolov5的推理输出结果:
```c++
#include <fstream>
#include <iostream>
#include <opencv2/opencv.hpp>
using namespace std;
using namespace cv;
struct DetectionObject {
Rect bbox;
string label;
float confidence;
};
vector<DetectionObject> parse_yolov5_output(const string& output_file, float confidence_threshold = 0.5) {
vector<DetectionObject> detection_objects;
ifstream ifs(output_file);
string line;
while (getline(ifs, line)) {
vector<string> fields;
stringstream ss(line);
string field;
while (getline(ss, field, ' ')) {
fields.push_back(field);
}
if (fields.size() == 6) {
float confidence = stof(fields[4]);
if (confidence > confidence_threshold) {
Rect bbox(stoi(fields[0]), stoi(fields[1]), stoi(fields[2]), stoi(fields[3]));
string label = fields[5];
detection_objects.push_back({bbox, label, confidence});
}
}
}
return detection_objects;
}
void draw_detection_result(Mat& image, const vector<DetectionObject>& detection_objects) {
for (const auto& obj : detection_objects) {
rectangle(image, obj.bbox, Scalar(0, 0, 255), 2);
putText(image, obj.label, Point(obj.bbox.x, obj.bbox.y - 5), FONT_HERSHEY_SIMPLEX, 0.5, Scalar(0, 0, 255), 1);
putText(image, to_string(obj.confidence), Point(obj.bbox.x + obj.bbox.width, obj.bbox.y - 5), FONT_HERSHEY_SIMPLEX, 0.5, Scalar(0, 0, 255), 1);
}
}
int main(int argc, char** argv) {
string output_file = "yolov5_output.txt";
Mat image = imread("test.jpg");
vector<DetectionObject> detection_objects = parse_yolov5_output(output_file);
draw_detection_result(image, detection_objects);
imshow("Detection Result", image);
waitKey(0);
return 0;
}
```
在上述示例代码中,`parse_yolov5_output`函数用于解析Yolov5的推理输出文件,返回一个包含所有检测目标信息的结构体向量;`draw_detection_result`函数用于将解析出的检测目标信息在图像中绘制出来。最后在`main`函数中,读取图像,并调用上述两个函数进行解析和绘图,并显示结果。
阅读全文
相关推荐
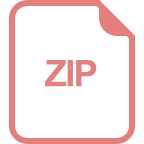
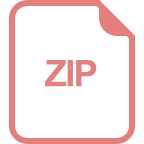
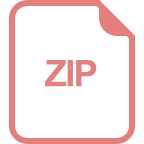
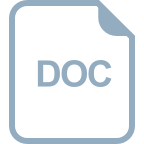











