lstm时间序列预测滚动预测代码
时间: 2024-11-03 09:13:05 浏览: 6
LSTM(长短期记忆网络)常用于时间序列预测,特别是当数据存在长期依赖性时。滚动预测是一种常用的训练策略,它允许模型逐步生成序列,并在每个步骤都更新其内部状态。
以下是一个简单的Python示例,使用Keras库实现LSTM进行滚动预测:
```python
import numpy as np
from keras.models import Sequential
from keras.layers import LSTM, Dense
# 假设你已经有了预处理的时间序列数据X_train和y_train
# X_train.shape应该是(batch_size, sequence_length, input_dim)
# y_train.shape应该是(batch_size, sequence_length, output_dim)
model = Sequential()
model.add(LSTM(64, return_sequences=True, input_shape=(None, X_train.shape[2]))) # 隐藏层大小
model.add(Dense(output_dim, activation='linear')) # 输出层
def rolling_predict(model, x, lookback, horizon):
predictions = []
for t in range(horizon):
# 使用当前时刻之前的lookback步作为输入
x_t = x[:, -lookback:, :]
# 进行预测
yhat = model.predict(x_t)[..., -1]
# 将预测添加到结果列表中
predictions.append(yhat)
# 更新输入序列,移除最旧的数据并追加新的预测结果
x = np.roll(x, shift=1, axis=1)
x[..., -1] = yhat
return np.array(predictions)
lookback = 10 # 滚动窗口大小
horizon = 5 # 预测步数
# 开始滚动预测
predictions = rolling_predict(model, X_train, lookback, horizon)
阅读全文
相关推荐
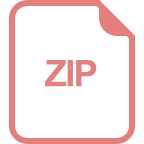
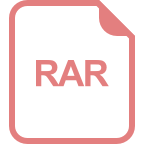
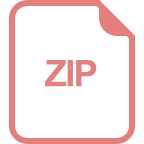

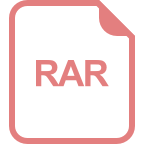
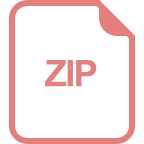
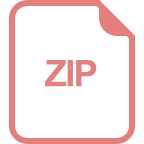
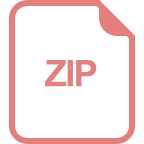
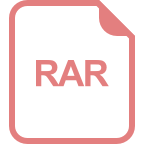
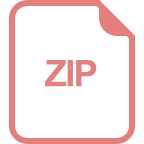
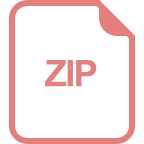
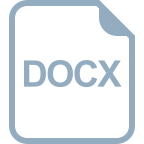
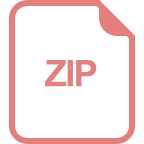
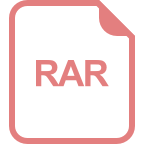
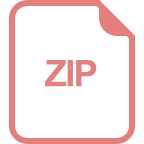
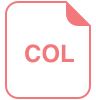
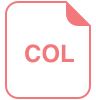
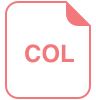