PGD生成对抗样本 测试集的代码
时间: 2023-10-20 14:07:27 浏览: 137
以下是一个用PGD(Projected Gradient Descent)算法生成对抗样本并在测试集上进行评估的代码示例:
```python
import torch
import torch.nn as nn
def pgd_attack(model, loss_fn, images, labels, epsilon, alpha, num_steps):
perturbed_images = images.clone().detach()
perturbed_images.requires_grad = True
for _ in range(num_steps):
# Forward pass
outputs = model(perturbed_images)
loss = loss_fn(outputs, labels)
# Zeroing out gradients
model.zero_grad()
# Backward pass
loss.backward()
# Collecting the gradient of the input image
grad = perturbed_images.grad.data
# Generating adversarial example
perturbed_images = perturbed_images + alpha * torch.sign(grad)
# Projecting the perturbations to epsilon ball
perturbations = torch.clamp(perturbed_images - images, -epsilon, epsilon)
perturbed_images = torch.clamp(images + perturbations, 0, 1)
# Resetting gradients
perturbed_images.grad.zero_()
return perturbed_images
# 示例用法
# 定义模型和损失函数
model = YourModel()
loss_fn = nn.CrossEntropyLoss()
# 定义输入图像和标签
test_images = torch.tensor(...) # 测试集图像
test_labels = torch.tensor(...) # 测试集标签
# 设置扰动大小 (epsilon)、步长 (alpha) 和迭代次数 (num_steps)
epsilon = 0.1
alpha = 0.01
num_steps = 10
# 生成对抗样本
perturbed_test_images = pgd_attack(model, loss_fn, test_images, test_labels, epsilon, alpha, num_steps)
# 在对抗样本上进行模型评估
outputs = model(perturbed_test_images)
predicted_labels = torch.argmax(outputs, dim=1)
# 计算准确率等评估指标
accuracy = (predicted_labels == test_labels).sum().item() / len(test_labels)
# 其他指标的计算...
# 打印评估结果
print(f"Accuracy: {accuracy:.4f}")
# 其他指标的打印...
```
请注意,这只是一个简单的示例,具体实现可能因你的模型结构和数据类型而有所不同。建议根据你的具体需求和模型架构来进行相应的修改和调整。另外,这里的代码示例是基于PyTorch框架的,如果你使用其他框架,可能需要进行相应的调整。
阅读全文
相关推荐
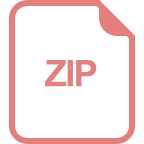
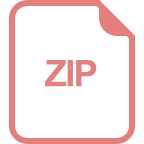




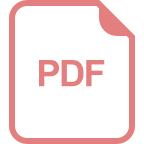
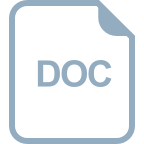
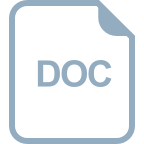
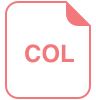
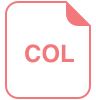
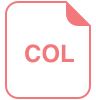
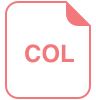
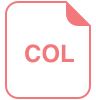
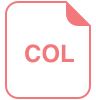


