Python编写程序,对彩色图像进行线性变换,分析斜率和截距对图像变换的影响
时间: 2024-09-20 14:05:56 浏览: 36
在Python中,我们可以使用PIL(Python Imaging Library)或其升级版Pillow库处理彩色图像,对于线性变换,常用的函数有`ImageEnhance`模块下的`ColorEnhancer`类,它可以调整图像的颜色饱和度、亮度等,本质上都是线性变换的一种。
对于斜率和截距,通常我们提到的是灰度图像中的线性变换,如灰度线性拉伸(Linear Grayscale Stretching),在这种情况下,你可以通过修改像素值的映射规则来进行。例如,假设原始灰度值范围是0到255,通过斜率k和截距b可以计算新的灰度值:
```python
def linear_transform(image, slope, intercept):
pixel_values = image.histogram()
new_values = [int((value - intercept) * slope + intercept) for value in pixel_values]
transformed_image = Image.fromarray(new_values, mode='L')
return transformed_image
```
在这个例子中,`slope`决定了新像素值变化的速度,而`intercept`则会平移整个灰度值范围。如果用于彩色图像,可能需要将这个操作应用到每个颜色通道上(红、绿、蓝RGB)。
相关问题
python线性回归怎么获取斜率和截距
在 Python 中,可以使用 scikit-learn 库中的 LinearRegression 模块来实现线性回归并获取斜率和截距。具体步骤如下:
1. 导入 LinearRegression 模块
```python
from sklearn.linear_model import LinearRegression
```
2. 准备数据
假设我们有一个包含自变量 x 和因变量 y 的数据集,可以将其分别存储在两个数组中(比如 numpy 数组)。
```python
import numpy as np
# 自变量 x
x = np.array([1, 2, 3, 4, 5])
# 因变量 y
y = np.array([2, 4, 5, 4, 5])
```
3. 创建 LinearRegression 模型
```python
model = LinearRegression()
```
4. 训练模型并获取斜率和截距
```python
# 训练模型
model.fit(x.reshape(-1, 1), y)
# 获取斜率和截距
slope = model.coef_[0]
intercept = model.intercept_
print("斜率:", slope)
print("截距:", intercept)
```
其中,`coef_` 属性返回的是一个数组,因为在多元线性回归中,每个自变量都有一个对应的系数。而在这个例子中,只有一个自变量 x,因此可以直接取出第一个元素。
值得注意的是,由于 LinearRegression 模块要求输入的自变量和因变量都是二维数组,因此需要使用 `reshape(-1, 1)` 将 x 转化为二维数组。
python如何求两个变量线性回归的斜率,截距以及均方误差?请给出实现函数。
可以使用 `sklearn` 库中的 `LinearRegression` 模型来求解线性回归的斜率、截距和均方误差。具体实现函数如下:
```python
from sklearn.linear_model import LinearRegression
from sklearn.metrics import mean_squared_error
def linear_regression(x, y):
# 创建线性回归模型
lr = LinearRegression()
# 训练模型
lr.fit(x, y)
# 获取斜率和截距
slope = lr.coef_[0]
intercept = lr.intercept_
# 预测结果
y_pred = lr.predict(x)
# 计算均方误差
mse = mean_squared_error(y, y_pred)
return slope, intercept, mse
```
其中,`x` 和 `y` 分别为两个变量的样本数据,`slope` 表示斜率,`intercept` 表示截距,`mse` 表示均方误差。使用方法如下:
```python
import numpy as np
# 生成样本数据
x = np.array([[1], [2], [3], [4], [5], [6], [7], [8], [9], [10]])
y = np.array([2, 4, 5, 7, 8, 10, 11, 13, 14, 16])
# 计算线性回归
slope, intercept, mse = linear_regression(x, y)
# 输出结果
print("斜率:", slope)
print("截距:", intercept)
print("均方误差:", mse)
```
相关推荐
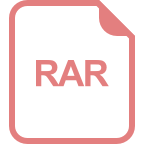
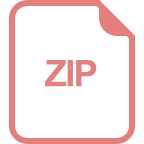
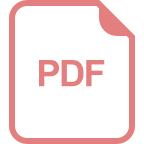
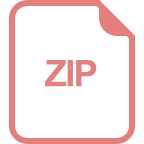
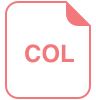










