ID3决策树算法matlab代码
时间: 2023-07-24 09:59:31 浏览: 78
以下是一个简单的使用MATLAB实现ID3决策树算法的示例代码:
```matlab
function [tree] = id3_decision_tree(data, attributes, target_attribute)
% 如果数据集中的所有实例都属于同一类别,则返回单节点树并将该类别作为标签
if all(data(:, target_attribute) == data(1, target_attribute))
tree = struct('class', data(1, target_attribute));
return;
end
% 如果属性集为空,则返回单节点树并将数据集中最常见的类别作为标签
if isempty(attributes)
class_counts = histc(data(:, target_attribute), unique(data(:, target_attribute)));
[~, idx] = max(class_counts);
tree = struct('class', unique(data(:, target_attribute))(idx));
return;
end
% 选择最佳属性作为当前节点
best_attribute = choose_best_attribute(data, attributes, target_attribute);
tree = struct('attribute', best_attribute, 'children', []);
% 对于每个属性值,递归构建子树
attribute_values = unique(data(:, best_attribute));
for i = 1:length(attribute_values)
value = attribute_values(i);
data_subset = data(data(:, best_attribute) == value, :);
if isempty(data_subset)
% 如果数据集为空,则返回单节点树并将数据集中最常见的类别作为标签
class_counts = histc(data(:, target_attribute), unique(data(:, target_attribute)));
[~, idx] = max(class_counts);
subtree = struct('class', unique(data(:, target_attribute))(idx));
else
% 否则,
相关推荐
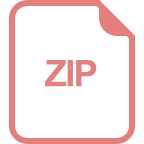














