1.指对minst数据集搭建三层全连接神经网络,隐含层神经元个数20个。 2.借鉴教学案例,采用GridSearchCV 实现网格搜索batch size、epochs、学习速率和动量因子的超参数优化
时间: 2024-11-29 22:38:24 浏览: 3
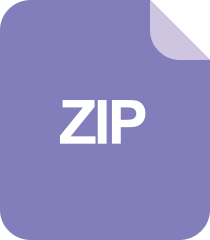
运用神经网络算法和Minst数据集制作的手写数字识别系统.zip
1. 对于MINST数据集(MNIST是一个手写数字图像数据库),构建一个包含三层的全连接神经网络,每层包括20个神经元,可以按照以下步骤操作:
- 首先,加载所需的库如TensorFlow或PyTorch,以及`tensorflow.keras`模块。
- 定义网络结构:创建一个Sequential模型,添加三个隐藏层,每个隐藏层使用ReLU激活函数,最后一层是输出层(对于分类任务通常使用softmax)。例如:
```python
from tensorflow.keras.models import Sequential
from tensorflow.keras.layers import Dense, Flatten
model = Sequential([
Flatten(input_shape=(28, 28)),
Dense(20, activation='relu'), # 第一层,28*28输入,20个神经元
Dense(20, activation='relu'), # 第二层
Dense(10, activation='softmax') # 输出层,10个类别
])
```
- 编译模型,设置损失函数(如categorical_crossentropy)、优化器(如Adam)以及评估指标(accuracy)。
```python
model.compile(optimizer='adam', loss='sparse_categorical_crossentropy', metrics=['accuracy'])
```
2. 使用GridSearchCV进行超参数优化:
- 导入`sklearn.model_selection`模块中的GridSearchCV和相关的预处理方法(如StandardScaler)。
- 确定需要优化的参数范围,比如批量大小(batch_size)、训练轮数(epochs),学习率(lr),和动量(momentum)。例如:
```python
param_grid = {
'batch_size': [32, 64, 128],
'epochs': [50, 100, 150],
'learning_rate': [0.001, 0.01, 0.1],
'momentum': [0.8, 0.9]
}
```
- 创建一个KerasClassifier对象,并传入上面定义的模型,然后应用GridSearchCV:
```python
from sklearn.preprocessing import StandardScaler
from sklearn.pipeline import make_pipeline
from keras.wrappers.scikit_learn import KerasClassifier
scaler = StandardScaler()
model = KerasClassifier(build_fn=model)
pipe = make_pipeline(scaler, model)
grid_search = GridSearchCV(pipe, param_grid, cv=3, scoring='accuracy')
grid_search.fit(X_train, y_train)
```
- 最后,你可以获取最佳参数组合及其对应的性能。
阅读全文
相关推荐
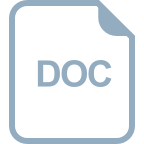
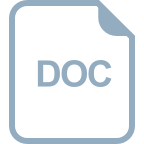
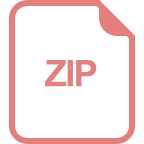
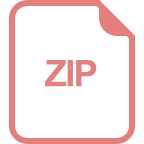
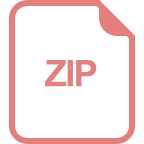
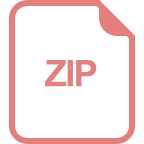
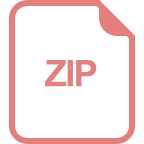
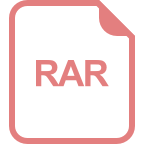
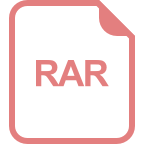
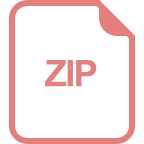
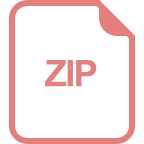
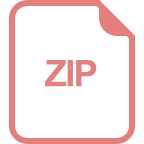
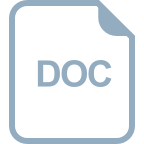


