使用Pytorch完成逻辑回归问题 1.创建一些随机数据,并将其转换为Tensor类型 随机数据代码: np.random.seed(0) X = np.random.randn(100, 2) Y = np.zeros((100,)) Y[X[:,0] + X[:,1] > 0] = 1
时间: 2024-03-01 07:54:07 浏览: 120
好的,下面是根据您提供的代码创建随机数据并将其转换为Tensor类型的代码:
```python
import numpy as np
import torch
# 创建随机数据
np.random.seed(0)
X = np.random.randn(100, 2)
Y = np.zeros((100,))
Y[X[:,0] + X[:,1] > 0] = 1
# 将数据转换为Tensor类型
x_data = torch.from_numpy(X).float()
y_data = torch.from_numpy(Y).float().view(-1, 1)
```
在这个例子中,我们使用NumPy生成了100个二维随机向量和它们的标签(根据两个特征相加是否大于0来判断标签)。然后,我们使用PyTorch的`torch.from_numpy()`方法将数据转换为Tensor类型,并使用`.float()`方法将数据类型转换为浮点型(默认情况下,`torch.from_numpy()`方法会生成双精度型数据)。最后,我们使用`.view()`方法将标签数据的形状从`(100,)`转换为`(100, 1)`。
阅读全文
相关推荐
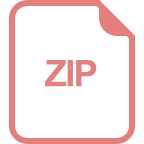
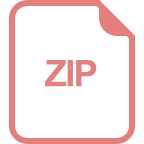
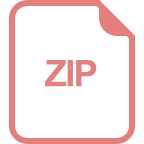
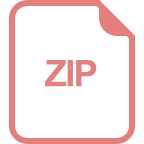
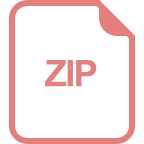
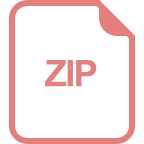
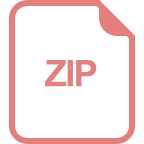
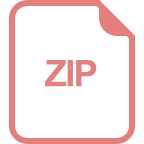
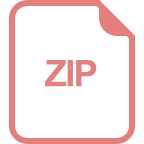
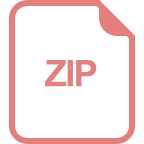
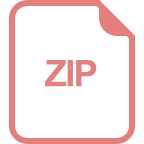
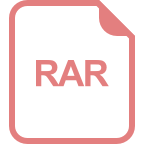
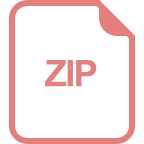