Apriori 算法java
时间: 2024-09-25 12:20:40 浏览: 30
Apriori算法是一种用于关联规则学习(Association Rule Learning)的经典算法,它主要用于数据挖掘领域,尤其是市场篮子分析。该算法的主要目标是从大量交易数据中发现频繁项集(频繁项目组合),进而推导出强关联规则。
在Java中实现Apriori算法通常会包括以下步骤:
1. **数据预处理**:加载交易数据,并将其转换成适合算法处理的形式,如键值对列表或矩阵。
2. **生成候选集**:从频繁一阶项集开始,通过合并形成更高阶的项集候选。
3. **计算支持度**:统计每个候选集在所有交易中的出现次数,得到其支持度。
4. **剪枝和筛选**:基于用户设定的支持度阈值,去除不满足条件的候选集。
5. **生成关联规则**:对于剩下的频繁项集,找出它们之间的关联规则。
以下是使用Java实现Apriori算法的一个简要框架:
```java
import java.util.*;
class Apriori {
// ...其他类方法和变量
public List<Rule> findRules(List<ItemSet> frequentItemsets, double minSupport) {
List<Rule> rules = new ArrayList<>();
for (int i = 1; i < frequentItemsets.size(); i++) {
ItemSet currentSet = frequentItemsets.get(i);
for (ItemSet prevSet : frequentItemsets.subList(0, i)) {
if (currentSet.isSubset(prevSet)) {
double support = calculateSupport(currentSet);
if (support >= minSupport) {
rules.add(new Rule(prevSet, currentSet));
}
}
}
}
return rules;
}
private double calculateSupport(ItemSet itemset) {
// 计算itemset的支持度,例如遍历数据集计数
}
}
// 定义ItemSet类和Rule类
class ItemSet { ... }
class Rule { ... }
```
相关推荐
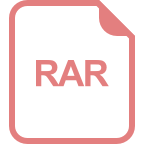
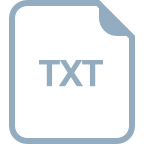
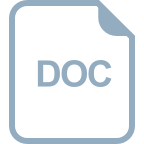
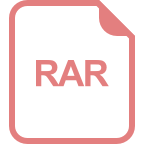
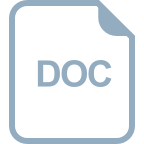
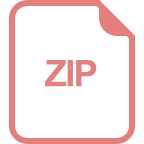
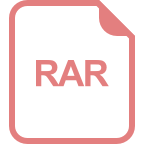
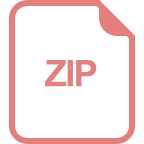
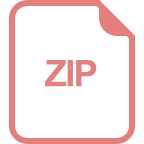
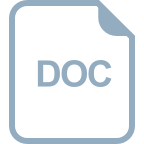
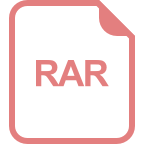
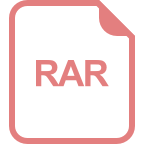

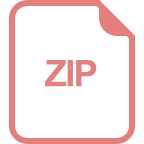
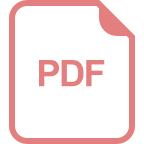
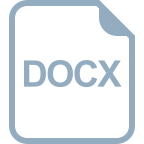