x = image_crop for block_id, (layer_num, chan_num, pool) in enumerate(zip(layers_per_block, out_chan_list, pool_list), 1): for layer_id in range(layer_num): x = ops.conv_relu(x, 'conv%d_%d' % (block_id, layer_id+1), kernel_size=3, stride=1, out_chan=chan_num, trainable=train) if pool: x = ops.max_pool(x, 'pool%d' % block_id)注释代码
时间: 2024-01-25 16:03:51 浏览: 16
这段代码是使用卷积神经网络对图像进行特征提取和池化操作。其中,通过循环遍历每个模块(block),获取该模块中卷积层数、输出通道数和是否进行池化操作的信息。然后在每个模块中,先进行若干个卷积层,每个卷积层包括一个卷积和ReLU激活函数,然后根据是否进行池化操作的标志进行池化,如果进行池化,则使用最大池化操作。整个过程中,使用了ops模块中的conv_relu和max_pool函数来实现。
相关问题
def load_data( *, data_dir, batch_size, image_size, class_cond=False, deterministic=False, random_crop=False, random_flip=False, ): mage.save_img(image_path, images) if not data_dir: raise ValueError("unspecified data directory") all_files = list_image_files_recursively(data_dir) # 递归的去找到所有图片文件 classes = None if class_cond: # Assume classes are the first part of the filename, # before an underscore. class_names = [bf.basename(path).split("")[0] for path in all_files] sorted_classes = {x: i for i, x in enumerate(sorted(set(class_names)))} # class id 将每一类class做成一个整型变量 classes = [sorted_classes[x] for x in class_names] dataset = ImageDataset( image_size, all_files, classes=classes, shard=MPI.COMM_WORLD.Get_rank(), num_shards=MPI.COMM_WORLD.Get_size(), random_crop=random_crop, random_flip=random_flip, ) if deterministic: loader = DataLoader( dataset, batch_size=batch_size, shuffle=False, num_workers=1, drop_last=True ) else: loader = DataLoader( dataset, batch_size=batch_size, shuffle=True, num_workers=1, drop_last=True ) while True: yield from loader,data = load_data( data_dir="F://diffuse_model_ptcg//picture//test", batch_size=4, image_size=128, class_cond=False, )for batch in data:load_data中第一个batch代表什么
在这段代码中,`load_data`函数返回一个Python生成器对象,使用`yield from loader`语句实现。每次调用这个生成器时,会从数据集中获取一个batch的数据,并将其返回。第一个batch代表的是数据集中的第一个batch,也就是在所有数据中的前`batch_size`个数据。在这个例子中,`batch_size`被设置为4,因此第一个batch代表数据集中的前4个数据。
def load_data( *, data_dir, batch_size, image_size, class_cond=False, deterministic=False, random_crop=False, random_flip=False, ): mage.save_img(image_path, images) if not data_dir: raise ValueError("unspecified data directory") all_files = _list_image_files_recursively(data_dir) # 递归的去找到所有图片文件 classes = None if class_cond: # Assume classes are the first part of the filename, # before an underscore. class_names = [bf.basename(path).split("_")[0] for path in all_files] sorted_classes = {x: i for i, x in enumerate(sorted(set(class_names)))} # class id 将每一类class做成一个整型变量 classes = [sorted_classes[x] for x in class_names] dataset = ImageDataset( image_size, all_files, classes=classes, shard=MPI.COMM_WORLD.Get_rank(), num_shards=MPI.COMM_WORLD.Get_size(), random_crop=random_crop, random_flip=random_flip, ) if deterministic: loader = DataLoader( dataset, batch_size=batch_size, shuffle=False, num_workers=1, drop_last=True ) else: loader = DataLoader( dataset, batch_size=batch_size, shuffle=True, num_workers=1, drop_last=True ) while True: yield from loader,data = load_data( data_dir="F://diffuse_model_ptcg//picture//test", batch_size=4, image_size=128, class_cond=False, )for batch in data:load_data中batch是无尽的吗
是的,load_data函数返回的是一个Python生成器对象,使用yield from语句使其不断地产生新的数据批次。因此,使用for循环遍历batch时可以得到无尽的数据批次,直到程序被手动终止或者发生错误。需要注意的是,由于数据集的大小通常是有限的,因此在使用生成器对象时应该谨慎,避免产生过多的数据批次导致内存溢出等问题。
阅读全文
相关推荐
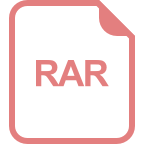
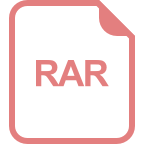



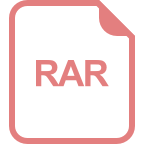
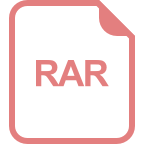
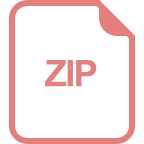
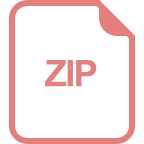
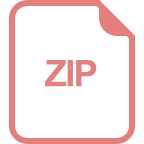
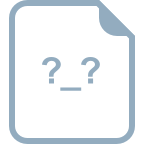
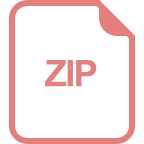
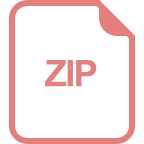
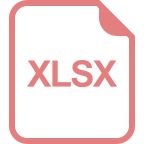