screeplot(PCA,type="lines") Error in plot.new() : figure margins too large
时间: 2024-01-22 13:21:01 浏览: 158
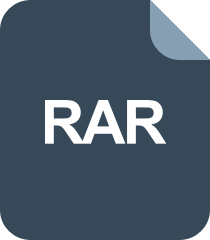
PCA.rar_pca设计英文_site:www.pudn.com
这个错误通常是由于图形区域的边界框太大而导致的。可以通过增加边界框的大小来解决此问题。尝试使用par()函数来设置边界框的大小,例如:
```
par(mar=c(5,5,2,2)) # 设置边界框的大小
screeplot(PCA,type="lines")
```
这将设置边界框的边距,左、右、上、下为5、5、2和2。你可以根据需要更改这些值。
阅读全文
相关推荐
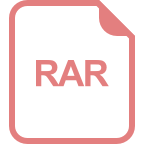
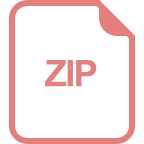
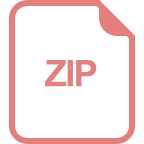
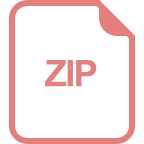
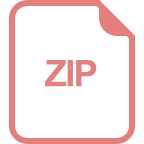
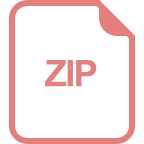
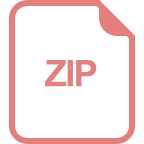
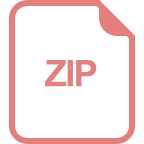
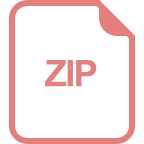
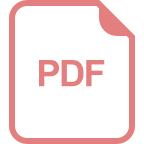
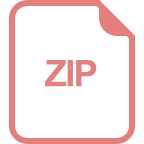
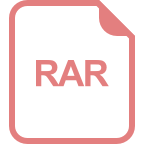
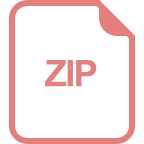
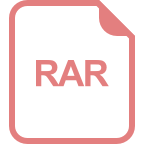