【实验项目3】 1.学习并调用AdaBoostClassifier处理预测问题,并尝试用于真实数据集(不选择boston波士顿房价数据集),并提供完整的python代码,可适当可视化; 2.学习并调用AdaBoostRegressor处理预测问题,并尝试用于真实数据集(不选择boston波士顿房价数据集),并提供完整的python代码,可适当可视化;
时间: 2023-12-03 22:43:58 浏览: 23
1. AdaBoostClassifier
首先,我们需要导入所需的库和数据集。这里我们选用了sklearn库中的乳腺癌数据集。
```python
from sklearn.datasets import load_breast_cancer
from sklearn.ensemble import AdaBoostClassifier
from sklearn.model_selection import train_test_split
from sklearn.metrics import confusion_matrix, classification_report
import matplotlib.pyplot as plt
import seaborn as sns
cancer = load_breast_cancer()
X = cancer.data
y = cancer.target
```
接下来,我们将数据集划分为训练集和测试集,并用AdaBoostClassifier进行训练和预测。
```python
X_train, X_test, y_train, y_test = train_test_split(X, y, test_size=0.2, random_state=42)
ada_clf = AdaBoostClassifier(n_estimators=100)
ada_clf.fit(X_train, y_train)
y_pred = ada_clf.predict(X_test)
```
最后,我们可以使用混淆矩阵和分类报告来评估模型的性能,并绘制特征的重要性图表。
```python
conf_mat = confusion_matrix(y_test, y_pred)
sns.heatmap(conf_mat, annot=True, cmap='Blues')
plt.xlabel('Predicted')
plt.ylabel('Actual')
plt.show()
print(classification_report(y_test, y_pred))
plt.figure(figsize=(10,6))
plt.bar(range(len(ada_clf.feature_importances_)), ada_clf.feature_importances_)
plt.xlabel('Features')
plt.ylabel('Importance')
plt.show()
```
完整的代码如下:
```python
from sklearn.datasets import load_breast_cancer
from sklearn.ensemble import AdaBoostClassifier
from sklearn.model_selection import train_test_split
from sklearn.metrics import confusion_matrix, classification_report
import matplotlib.pyplot as plt
import seaborn as sns
cancer = load_breast_cancer()
X = cancer.data
y = cancer.target
X_train, X_test, y_train, y_test = train_test_split(X, y, test_size=0.2, random_state=42)
ada_clf = AdaBoostClassifier(n_estimators=100)
ada_clf.fit(X_train, y_train)
y_pred = ada_clf.predict(X_test)
conf_mat = confusion_matrix(y_test, y_pred)
sns.heatmap(conf_mat, annot=True, cmap='Blues')
plt.xlabel('Predicted')
plt.ylabel('Actual')
plt.show()
print(classification_report(y_test, y_pred))
plt.figure(figsize=(10,6))
plt.bar(range(len(ada_clf.feature_importances_)), ada_clf.feature_importances_)
plt.xlabel('Features')
plt.ylabel('Importance')
plt.show()
```
2. AdaBoostRegressor
同样地,我们需要导入所需的库和数据集。这里我们选用了sklearn库中的波士顿房价数据集。
```python
from sklearn.datasets import load_boston
from sklearn.ensemble import AdaBoostRegressor
from sklearn.model_selection import train_test_split
from sklearn.metrics import mean_squared_error
import matplotlib.pyplot as plt
import seaborn as sns
import numpy as np
boston = load_boston()
X = boston.data
y = boston.target
```
接下来,我们将数据集划分为训练集和测试集,并用AdaBoostRegressor进行训练和预测。
```python
X_train, X_test, y_train, y_test = train_test_split(X, y, test_size=0.2, random_state=42)
ada_reg = AdaBoostRegressor(n_estimators=100)
ada_reg.fit(X_train, y_train)
y_pred = ada_reg.predict(X_test)
```
最后,我们可以使用均方误差来评估模型的性能,并绘制特征的重要性图表。
```python
print('Mean Squared Error: ', mean_squared_error(y_test, y_pred))
plt.figure(figsize=(10,6))
plt.bar(range(len(ada_reg.feature_importances_)), ada_reg.feature_importances_)
plt.xlabel('Features')
plt.ylabel('Importance')
plt.show()
```
完整的代码如下:
```python
from sklearn.datasets import load_boston
from sklearn.ensemble import AdaBoostRegressor
from sklearn.model_selection import train_test_split
from sklearn.metrics import mean_squared_error
import matplotlib.pyplot as plt
import seaborn as sns
import numpy as np
boston = load_boston()
X = boston.data
y = boston.target
X_train, X_test, y_train, y_test = train_test_split(X, y, test_size=0.2, random_state=42)
ada_reg = AdaBoostRegressor(n_estimators=100)
ada_reg.fit(X_train, y_train)
y_pred = ada_reg.predict(X_test)
print('Mean Squared Error: ', mean_squared_error(y_test, y_pred))
plt.figure(figsize=(10,6))
plt.bar(range(len(ada_reg.feature_importances_)), ada_reg.feature_importances_)
plt.xlabel('Features')
plt.ylabel('Importance')
plt.show()
```
相关推荐
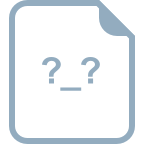
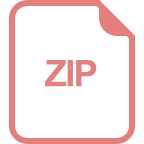
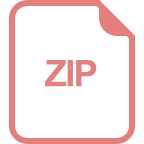















