实验一 利用线性回归预测鲍鱼年龄 实验目的: 1、 理解线性回归基本原理; 2、 学会用python实现线性回归; 3、 学会用sklearn实现线性回归。 实验内容: 1、 用python编写线性回归程序,其中求解目标函数的方法是正规方程法; 2、 应用预测鲍鱼年龄数据集对上述算法进行验证,画出可视化图形,并进行分析。 3、 代码参考《机器学习实战》第8章“预测数值型数据:回归”相关内容。
时间: 2023-05-30 18:03:15 浏览: 515
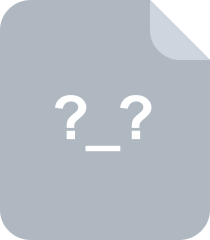
abalone.csv
实验步骤:
1、导入所需库和数据集
```python
import numpy as np
import pandas as pd
import matplotlib.pyplot as plt
from sklearn.linear_model import LinearRegression
df = pd.read_csv('abalone.csv', header=None, names=['Sex', 'Length', 'Diameter', 'Height', 'Whole weight', 'Shucked weight', 'Viscera weight', 'Shell weight', 'Rings'])
```
2、将字符串类型的性别转换成数值型
```python
df['Sex'] = df['Sex'].map({'M': 1, 'F': 2, 'I': 3})
```
3、将数据集分成训练集和测试集
```python
train_df = df[:-1000]
test_df = df[-1000:]
```
4、定义线性回归模型并训练模型
```python
X_train = train_df.drop('Rings', axis=1)
y_train = train_df['Rings']
lr = LinearRegression()
lr.fit(X_train, y_train)
```
5、预测测试集并计算均方误差
```python
X_test = test_df.drop('Rings', axis=1)
y_test = test_df['Rings']
y_pred = lr.predict(X_test)
mse = np.mean((y_test - y_pred) ** 2)
print('Mean squared error:', mse)
```
6、可视化展示预测结果
```python
plt.scatter(y_test, y_pred)
plt.plot([0, 30], [0, 30], '--k')
plt.xlabel('True values')
plt.ylabel('Predictions')
plt.show()
```
7、分析预测结果
从可视化图形上看,预测结果与真实值比较接近,但也存在一些误差。均方误差也比较小,说明模型的预测能力还是比较不错的。但需要注意的是,这里只使用了线性回归模型,可能存在欠拟合的情况,因此可以尝试使用其他更复杂的模型进行预测。
阅读全文
相关推荐
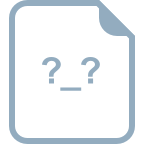
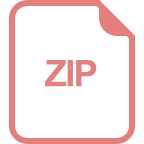
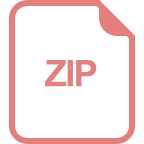
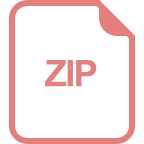
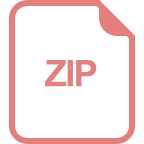
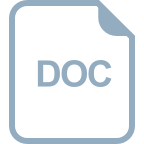
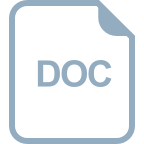
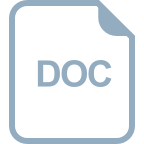
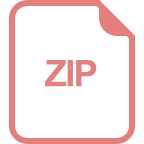
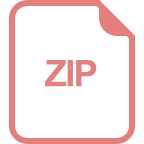
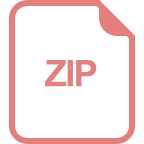
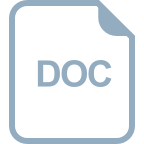
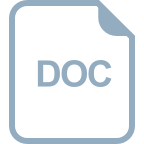
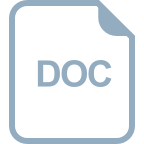
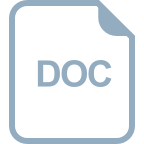

