PHOG特征 python
时间: 2023-10-12 22:06:21 浏览: 123
PHOG(Pyramid Histogram of Oriented Gradients)特征是一种用于图像识别和对象检测的特征描述方法,它是从HOG(Histogram of Oriented Gradients)特征发展而来的。PHOG特征通过将图像划分为不同尺度的金字塔,然后在每个金字塔层级上计算HOG特征,最后将这些特征进行整合。这样可以有效地捕捉图像的局部纹理和全局结构。
在Python中,可以使用一些开源库来计算PHOG特征,如OpenCV和scikit-image。以下是一个简单示例,展示了如何使用scikit-image库计算图像的PHOG特征:
```python
from skimage.feature import hog
from skimage import data, exposure
# 读取图像
image = data.astronaut()
# 计算PHOG特征
features, hog_image = hog(image, orientations=9, pixels_per_cell=(16, 16),
cells_per_block=(2, 2), visualize=True, multichannel=True)
# 对特征进行可视化
hog_image_rescaled = exposure.rescale_intensity(hog_image, in_range=(0, 10))
# 显示原始图像和PHOG特征图
import matplotlib.pyplot as plt
fig, (ax1, ax2) = plt.subplots(1, 2, figsize=(8, 4), sharex=True, sharey=True)
ax1.axis('off')
ax1.imshow(image, cmap=plt.cm.gray)
ax1.set_title('Input image')
ax2.axis('off')
ax2.imshow(hog_image_rescaled, cmap=plt.cm.gray)
ax2.set_title('PHOG')
plt.show()
```
在这个示例中,我们首先使用scikit-image的`hog`函数计算图像的PHOG特征。然后,通过调整`orientations`、`pixels_per_cell`和`cells_per_block`参数,可以控制特征的维度和精度。最后,我们使用matplotlib库将原始图像和PHOG特征图进行可视化。
请注意,这只是一个简单的示例,实际应用中可能需要根据具体问题进行适当的参数选择和特征处理。
阅读全文
相关推荐
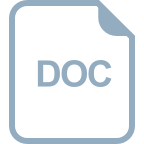
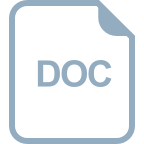
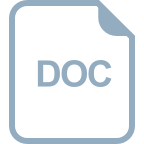
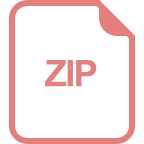
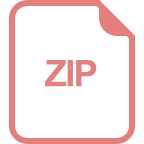
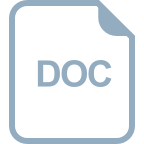
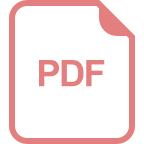
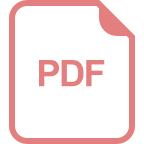
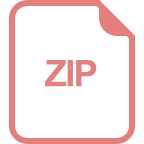
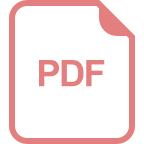
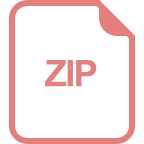
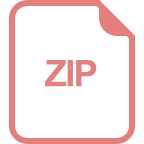
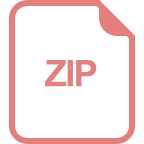
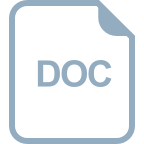
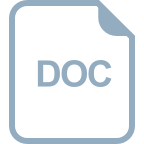
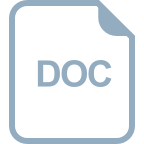
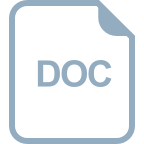

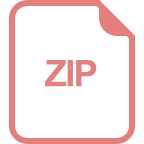