查找gain的计算方式
时间: 2023-07-29 22:06:29 浏览: 78
"Gain"在不同领域有不同的含义,这里我假设你是指在摄影或摄像领域中,相机或摄像机的增益计算方式。在这种情况下,增益通常是指摄像机或相机增强图像亮度的方法。
在摄影或摄像领域中,增益通常是以dB(分贝)为单位来表示的。增益值越大,图像的亮度就越高。
增益(dB)= 20log10(Vout / Vin)
其中,Vout表示输出信号的电压,Vin表示输入信号的电压。
在相机或摄像机中,增益通常是指图像传感器的电子放大倍数。增益越高,图像噪点就越多,因此需要根据实际情况来调整增益。
相关问题
Openmv查找直线
Openmv可以使用find_lines函数来查找直线。该函数使用霍夫变换返回所有直线对象。可以通过限制寻找直线的角度来找到图像中所有的横线和竖线。可以使用min_degree和max_degree参数来限制直线的角度范围。例如,可以设置min_degree为80,max_degree为100来找到图像中的横线和竖线。可以使用img.draw_line函数将找到的直线绘制在图像上。可以使用img.find_lines函数来找到所有的横线和竖线,并将其存储在heng和shu列表中。[1]
另外,Openmv还提供了其他函数来处理图像,如find_blobs函数用于查找色块,find_line_segments函数用于查找线段,find_circles函数用于查找圆形,find_rects函数用于查找矩形等。可以使用这些函数来进一步处理图像中的其他特征。[2]
以下是一个示例代码,演示了如何使用Openmv进行图像二值化和直线拟合显示:
import sensor
import image
import time
sensor.reset()
sensor.set_pixformat(sensor.GRAYSCALE)
sensor.set_framesize(sensor.QQQVGA)
sensor.skip_frames(time=3000)
sensor.set_auto_gain(False)
sensor.set_auto_whitebal(False)
clock = time.clock()
THRESHOLD = (0, 110) # 二值化阈值(灰度)
while True:
img = sensor.snapshot().binary([THRESHOLD]) # 二值化
line = img.get_regression([(255, 255)], robust=True) # (255,255):追踪的颜色范围 robust=True:使用Theil-Sen线性回归算法
if line: # 如果存在符合要求的直线
rho_err = abs(line.rho()) - img.width() / 2 # rho_err:负值直线在左侧正值在右侧,若为水平线的中垂线则为0
# line.rho():通过霍夫变换拟合出直线相对于原点的距离(像素数)(即r=xcosθ+ysinθ中的r)
# img.width():以像素计图像的宽度
if line.theta() > 90: # line.theta():0-90 Y 半轴和直线的夹角,90-180 Y-半轴和直线的夹角
theta_err = line.theta() - 180
else:
theta_err = line.theta() # 处理后:绝对值为直线同Y轴的夹角,右正左负
img.draw_line(line.line(), color=127)
print(rho_err, line.rho(), line.magnitude(), line.theta(), theta_err)
# line.magnitude():霍夫变换后所得直线的模
这段代码使用sensor模块来初始化摄像头,设置图像格式和大小,并跳过前几帧以使摄像头稳定。然后设置图像的自动增益和自动白平衡。接下来,使用while循环来不断获取图像并进行处理。首先将图像进行二值化处理,然后使用get_regression函数拟合直线。如果存在符合要求的直线,计算直线的偏差和角度,并将直线绘制在图像上。最后打印出直线的偏差、距离、模和角度等信息。[3]
要求学生掌握《人工智能概论》课程中的基本知识点,了解常用的人工智能方法,能够查找资料,完成相关算法设计。基于以上知识点,使用决策树算法(ID3、C4.5、CART三选一)方法对iris数据集进行分类。
好的,基于以上要求,我可以给您提供一个简单的决策树分类算法的代码示例,用来对iris数据集进行分类。这里我选择使用ID3算法。代码如下:
```python
import pandas as pd
import numpy as np
# 导入数据集
url = "https://archive.ics.uci.edu/ml/machine-learning-databases/iris/iris.data"
names = ['sepal-length', 'sepal-width', 'petal-length', 'petal-width', 'class']
dataset = pd.read_csv(url, names=names)
# 划分训练集和测试集
train = dataset.sample(frac=0.8, random_state=1)
test = dataset.drop(train.index)
# 定义节点类
class Node:
def __init__(self, data, labels):
self.data = data # 当前节点的数据集
self.labels = labels # 当前节点的标签列表
self.children = {} # 当前节点的子节点
self.split_feature = None # 当前节点的分裂属性
self.majority_class = None # 当前节点所属的多数类
# 计算信息熵
def entropy(labels):
n_labels = len(labels)
if n_labels <= 1:
return 0
counts = np.bincount(labels)
probs = counts / n_labels
n_classes = np.count_nonzero(probs)
if n_classes <= 1:
return 0
ent = 0.
for i in probs:
ent -= i * np.log2(i)
return ent
# 计算信息增益
def info_gain(data, labels, split_feature):
base_entropy = entropy(labels)
split_entropy = 0.
n_data = len(labels)
for value in set(data[:, split_feature]):
sub_data = data[data[:, split_feature] == value]
sub_labels = labels[data[:, split_feature] == value]
split_entropy += len(sub_labels) / n_data * entropy(sub_labels)
return base_entropy - split_entropy
# 选择最优分裂属性
def choose_feature(node):
data = node.data
labels = node.labels
n_features = data.shape[1]
best_feature = None
best_info_gain = -np.inf
for i in range(n_features):
current_info_gain = info_gain(data, labels, i)
if current_info_gain > best_info_gain:
best_feature = i
best_info_gain = current_info_gain
return best_feature
# 构建决策树
def build_tree(node):
data = node.data
labels = node.labels
n_labels = len(labels)
n_classes = len(set(labels))
if n_labels == 0:
node.majority_class = -1
return
if n_classes == 1:
node.majority_class = labels[0]
return
if data.shape[1] == 0:
node.majority_class = np.argmax(np.bincount(labels))
return
node.split_feature = choose_feature(node)
for value in set(data[:, node.split_feature]):
sub_data = data[data[:, node.split_feature] == value]
sub_labels = labels[data[:, node.split_feature] == value]
node.children[value] = Node(sub_data, sub_labels)
build_tree(node.children[value])
# 预测
def predict(node, sample):
if not node.children:
return node.majority_class
if sample[node.split_feature] not in node.children:
return node.majority_class
return predict(node.children[sample[node.split_feature]], sample)
# 计算准确率
def accuracy(tree, test):
correct = 0
for i in range(test.shape[0]):
if predict(tree, test.iloc[i, :-1]) == test.iloc[i, -1]:
correct += 1
return correct / test.shape[0]
# 主函数
if __name__ == '__main__':
# 划分训练集和测试集
train = dataset.sample(frac=0.8, random_state=1)
test = dataset.drop(train.index)
# 构建决策树
root = Node(train.iloc[:, :-1].values, train.iloc[:, -1].values)
build_tree(root)
# 测试并输出准确率
acc = accuracy(root, test)
print("Accuracy:", acc)
```
需要注意的是,这段代码使用ID3算法来构建决策树,并对iris数据集进行分类。如果您需要使用C4.5或CART算法,只需要在代码中进行相应的修改即可。另外,您还需要安装pandas和numpy这两个库。
阅读全文
相关推荐
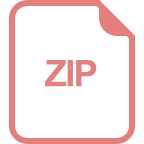
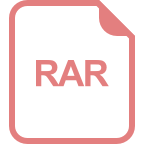
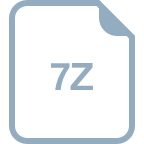
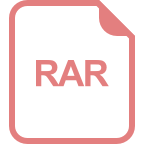
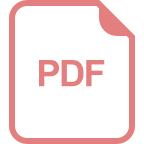
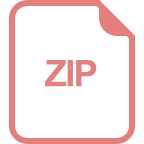
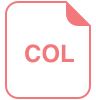
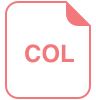
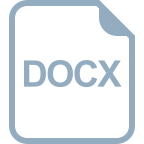
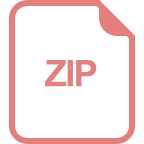
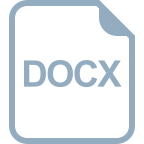
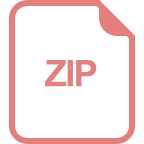