如何生成one-hot编码
时间: 2023-09-08 16:12:11 浏览: 89
生成one-hot编码可以使用sklearn库中的OneHotEncoder类。以下是一个简单的示例:
```python
from sklearn.preprocessing import OneHotEncoder
import numpy as np
# 创建示例数据
data = np.array([['apple', 'red'], ['banana', 'yellow'], ['orange', 'orange']])
print('原始数据:')
print(data)
# 创建OneHotEncoder对象
encoder = OneHotEncoder()
# 将数据转换为one-hot编码
one_hot = encoder.fit_transform(data).toarray()
print('one-hot编码:')
print(one_hot)
```
输出:
```
原始数据:
[['apple' 'red']
['banana' 'yellow']
['orange' 'orange']]
one-hot编码:
[[1. 0. 0. 1. 0. 0.]
[0. 1. 0. 0. 1. 0.]
[0. 0. 1. 0. 0. 1.]]
```
在这个示例中,我们首先创建了一个包含三行两列的数据数组。然后,我们使用OneHotEncoder类将其转换为one-hot编码。输出结果是一个包含六列的新数组,其中每列代表一个唯一的类别。如果原始数据中包含N个不同的类别,那么one-hot编码后的数据将包含N列。
相关问题
用jupyter生成One-Hot编码构建词向量,包括1.导入库2.输入示例词汇表3.构建One-Hot编码器4.调用编码器输出结果过程。
在Jupyter Notebook中进行One-Hot编码并构建词向量的基本步骤如下:
1. 导入必要的库:
首先,你需要导入`pandas`处理文本数据,`sklearn`库中的`preprocessing`模块提供One-Hot编码功能,以及`numpy`处理数组操作。
```python
import pandas as pd
from sklearn.preprocessing import OneHotEncoder
import numpy as np
```
2. 输入示例词汇表:
假设我们有一个包含单词的列表,可以这样创建:
```python
vocabulary = ['I', 'am', 'a', 'student', 'learning', 'Python']
```
3. 构建One-Hot编码器:
使用`OneHotEncoder`初始化,并指定类别编码策略(默认为序数编码)。这里我们假设每个单词都是唯一的类别。
```python
encoder = OneHotEncoder(sparse=False, categories='auto')
```
4. 转换输入词汇到One-Hot编码:
现在我们可以将词汇表转换为One-Hot编码。这需要我们将词汇作为一系列索引值传递给编码器的`fit_transform()`方法,因为OneHotEncoder需要训练才能确定每个单词对应的编码。
```python
# 假设data是一个DataFrame,每一列是单个单词的出现情况
if isinstance(vocabulary[0], str): # 如果词汇是字符串
data = pd.Series(vocabulary).value_counts().to_frame()
encoded_data = encoder.fit_transform(data.index.values.reshape(-1, 1))
else: # 如果词汇是数字或其他序列
encoded_data = encoder.fit_transform(np.array(vocabulary).reshape(-1, 1))
```
5. 输出结果:
`encoded_data`现在是一个二维数组,其中每行对应一个单词,而列则是二进制表示,1表示该单词在该位置存在,0表示不存在。你可以查看前几行来了解结果。
```python
print(encoded_data[:5])
```
nn.CrossEntropyLoss 会自动将目标标签转换为 one-hot 编码,并计算交叉熵损失。这不是自动将目标标签转换为 one-hot 编码吗?
非常抱歉,我的回答有误。在使用 `nn.CrossEntropyLoss` 计算损失时,不会自动将目标标签转换为 one-hot 编码。相反,它需要整数标签作为目标标签,并将其转换为与预测值相同的张量形状。因此,你在使用 `nn.CrossEntropyLoss` 时,需要将整数标签作为目标标签,而不是 one-hot 编码。
阅读全文
相关推荐
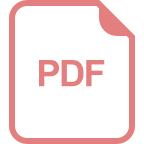












