def loc_glo(b = 2,a = 4): global z z += 3 * a + 5 * b return z z = 10 print(z,loc_glo(4,2))
时间: 2024-05-12 15:14:18 浏览: 61
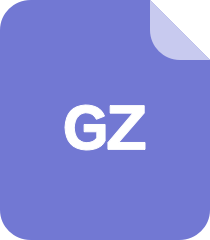
将两个递增的顺序表a,b;把a与b合并到a中,把a打印出来,就是,a与b数据递增的情况
这段代码的输出结果将会是:
```
10 28
```
首先,我们在全局变量中给 z 赋值为 10。然后,我们调用 loc_glo 函数,传入参数 b=4 和 a=2。
在函数内部,我们使用了 global 关键字来声明 z 为全局变量,然后对 z 进行了计算:z += 3 * a + 5 * b,即 z = z + (3 * a + 5 * b) = 10 + (3 * 2 + 5 * 4) = 28。
最后,我们打印出 z 和 loc_glo 函数的返回值,分别为 10 和 28。
阅读全文
相关推荐
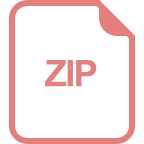

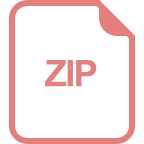
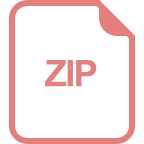
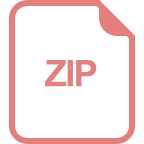
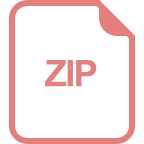
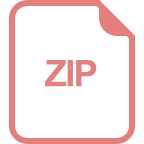
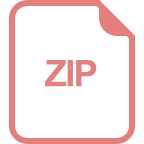
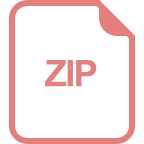
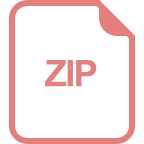
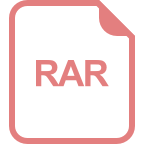
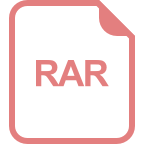
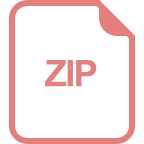
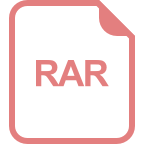
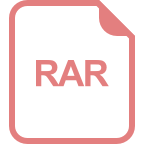
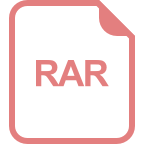