使用gradio来测试yolov5训练后的模型
时间: 2024-02-05 12:02:46 浏览: 160
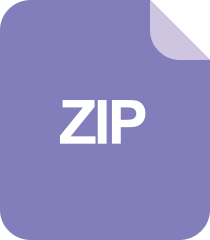
基于Gradio Blocks的YOLOv5目标检测设计源码及演示系统
好的,我们可以使用 Gradio 来测试 YOLOv5 训练后的模型。首先,您需要安装 Gradio 和 YOLOv5。
安装 Gradio:
```
!pip install gradio
```
安装 YOLOv5:
```
!git clone https://github.com/ultralytics/yolov5.git
```
然后,您需要下载 YOLOv5 训练后的模型权重文件(.pt文件)。您可以在 https://github.com/ultralytics/yolov5/releases 下载预训练的模型权重文件,或者使用您自己训练的权重文件。
在您的 Jupyter Notebook 中,您可以使用以下代码来加载模型并使用 Gradio 进行测试:
```python
import gradio as gr
import torch
import cv2
from PIL import Image
from pathlib import Path
# Load YOLOv5 model
model = torch.hub.load('ultralytics/yolov5', 'custom', path_or_model='path/to/your/weights.pt')
# Define prediction function
def predict(input_image):
# Convert Gradio input image to OpenCV format
input_image = cv2.cvtColor(input_image, cv2.COLOR_RGB2BGR)
# Use YOLOv5 model to predict objects
results = model(input_image)
# Draw bounding boxes on image
results.render()
# Convert OpenCV image to PIL format
output_image = Image.fromarray(cv2.cvtColor(results.imgs[0], cv2.COLOR_BGR2RGB))
# Return predicted image
return output_image
# Define Gradio interface
inputs = gr.inputs.Image()
outputs = gr.outputs.Image()
gr_interface = gr.Interface(fn=predict, inputs=inputs, outputs=outputs, title='YOLOv5 Object Detection')
# Launch Gradio interface
gr_interface.launch()
```
在这个例子中,我们使用了 YOLOv5 预训练的模型权重文件。如果您使用自己的权重文件,需要将 `path/to/your/weights.pt` 替换为您的权重文件路径。
阅读全文
相关推荐
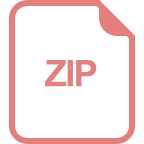
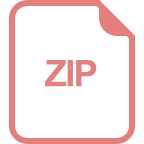















