基于pytorch的unet 和RNN的结合代码
时间: 2023-06-16 16:05:53 浏览: 166
以下是基于PyTorch的UNet和RNN结合的代码示例:
```python
import torch
import torch.nn as nn
import torch.nn.functional as F
class UNetRNN(nn.Module):
def __init__(self, input_channels, hidden_size, num_layers):
super(UNetRNN, self).__init__()
self.input_channels = input_channels
self.hidden_size = hidden_size
self.num_layers = num_layers
# UNet encoder
self.conv1 = nn.Conv2d(input_channels, 64, kernel_size=3, padding=1)
self.conv2 = nn.Conv2d(64, 128, kernel_size=3, padding=1)
self.conv3 = nn.Conv2d(128, 256, kernel_size=3, padding=1)
self.conv4 = nn.Conv2d(256, 512, kernel_size=3, padding=1)
self.conv5 = nn.Conv2d(512, 1024, kernel_size=3, padding=1)
self.pool = nn.MaxPool2d(kernel_size=2, stride=2)
# UNet decoder
self.upconv6 = nn.ConvTranspose2d(1024, 512, kernel_size=2, stride=2)
self.conv6 = nn.Conv2d(1024, 512, kernel_size=3, padding=1)
self.upconv7 = nn.ConvTranspose2d(512, 256, kernel_size=2, stride=2)
self.conv7 = nn.Conv2d(512, 256, kernel_size=3, padding=1)
self.upconv8 = nn.ConvTranspose2d(256, 128, kernel_size=2, stride=2)
self.conv8 = nn.Conv2d(256, 128, kernel_size=3, padding=1)
self.upconv9 = nn.ConvTranspose2d(128, 64, kernel_size=2, stride=2)
self.conv9 = nn.Conv2d(128, 64, kernel_size=3, padding=1)
self.conv10 = nn.Conv2d(64, input_channels, kernel_size=1)
# RNN layers
self.rnn = nn.RNN(input_size=input_channels, hidden_size=hidden_size, num_layers=num_layers, batch_first=True)
self.fc = nn.Linear(hidden_size, input_channels)
def forward(self, x, h0=None):
# UNet encoding
x1 = F.relu(self.conv1(x))
x2 = self.pool(x1)
x2 = F.relu(self.conv2(x2))
x3 = self.pool(x2)
x3 = F.relu(self.conv3(x3))
x4 = self.pool(x3)
x4 = F.relu(self.conv4(x4))
x5 = self.pool(x4)
x5 = F.relu(self.conv5(x5))
# UNet decoding
x6 = self.upconv6(x5)
x6 = torch.cat([x6, x4], dim=1)
x6 = F.relu(self.conv6(x6))
x7 = self.upconv7(x6)
x7 = torch.cat([x7, x3], dim=1)
x7 = F.relu(self.conv7(x7))
x8 = self.upconv8(x7)
x8 = torch.cat([x8, x2], dim=1)
x8 = F.relu(self.conv8(x8))
x9 = self.upconv9(x8)
x9 = torch.cat([x9, x1], dim=1)
x9 = F.relu(self.conv9(x9))
x10 = self.conv10(x9)
# RNN encoding
batch_size, channels, height, width = x10.size()
x10 = x10.permute(0, 2, 3, 1).contiguous().view(batch_size, height, width, channels)
x10 = x10.view(batch_size, height * width, channels)
if h0 is None:
h0 = torch.zeros(self.num_layers, batch_size, self.hidden_size).to(x.device)
x10, hn = self.rnn(x10, h0)
x10 = self.fc(x10)
x10 = x10.view(batch_size, height, width, channels).permute(0, 3, 1, 2)
return x10, hn
```
在这个例子中,我们定义了一个名为`UNetRNN`的类,它包含了一个UNet编码器和解码器,以及一个RNN编码器。在前向传递中,我们首先对输入图像进行UNet编码,然后将编码结果传递给RNN编码器。最后,我们将RNN编码器的输出传递给UNet解码器,以生成最终的输出图像。
注意,在RNN编码器中,我们使用了`nn.RNN`模块,它接受一个批次、序列长度和特征数的输入,并返回一个新的序列和最后一个隐藏状态。我们还添加了一个全连接层,将隐藏状态映射到输出通道数。
此外,在每个UNet解码器中,我们使用了转置卷积层来将特征图的大小增加一倍。我们还使用了`torch.cat`操作来将编码器和解码器的特征图连接起来。
最后,在RNN编码器的第一次迭代中,我们需要初始化隐藏状态。在这个例子中,我们默认使用全零张量作为初始隐藏状态,但你也可以根据需要自定义初始隐藏状态。
阅读全文
相关推荐
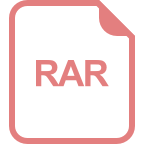
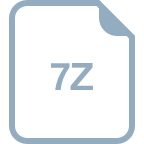

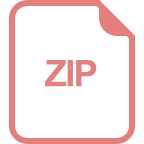
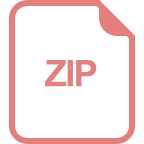
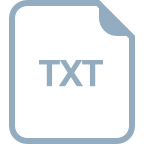
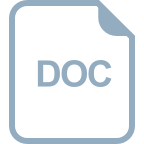
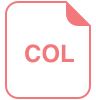

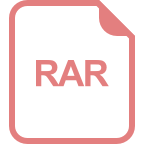
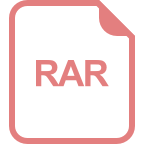
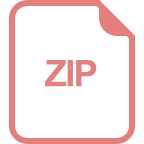
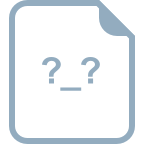
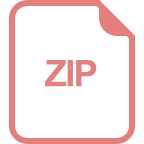
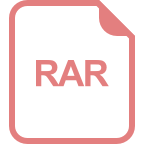