用python实现黑白照片的彩色化,写出完整代码实现其基本功能
时间: 2024-06-09 09:11:10 浏览: 83
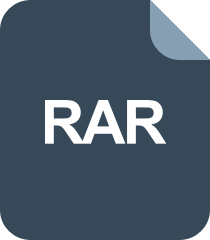
python实现灰度图像彩色化
黑白照片的彩色化是一个比较复杂的问题,需要使用深度学习技术来实现。在这里,我们可以使用一种基于深度学习的方法,称为颜色化自编码器(Colorization Autoencoder)来实现。
首先,我们需要准备一些数据。我们可以使用一些有彩色的图像和相应的黑白图像来训练我们的模型。在这里,我们将使用CIFAR-10数据集来训练我们的模型。
接下来,我们需要定义我们的模型。我们可以使用Keras来实现我们的模型。我们将使用一个编码器和一个解码器来实现我们的自编码器。编码器将黑白图像转换为低维表示,然后解码器将这个低维表示转换回彩色图像。
最后,我们需要训练我们的模型。我们可以使用反向传播算法来训练我们的模型。我们的目标是最小化预测图像与真实图像之间的差异。
以下是实现黑白照片彩色化的基本代码:
```python
# 导入所需库
import keras
from keras.layers import Conv2D, UpSampling2D, InputLayer
from keras.models import Sequential
from keras.preprocessing.image import ImageDataGenerator
import numpy as np
import matplotlib.pyplot as plt
# 加载CIFAR-10数据集
(X_train, y_train), (X_test, y_test) = keras.datasets.cifar10.load_data()
# 将图像转换为灰度图像
X_train_gray = np.dot(X_train[..., :3], [0.2989, 0.5870, 0.1140])
X_test_gray = np.dot(X_test[..., :3], [0.2989, 0.5870, 0.1140])
# 将图像归一化
X_train_gray = X_train_gray / 255
X_test_gray = X_test_gray / 255
# 将图像重塑为(32,32,1)
X_train_gray = X_train_gray.reshape(X_train_gray.shape + (1,))
X_test_gray = X_test_gray.reshape(X_test_gray.shape + (1,))
# 定义自编码器模型
model = Sequential()
model.add(InputLayer(input_shape=(32, 32, 1)))
model.add(Conv2D(64, (3, 3), activation='relu', padding='same'))
model.add(Conv2D(32, (3, 3), activation='relu', padding='same'))
model.add(Conv2D(32, (3, 3), activation='relu', padding='same'))
model.add(Conv2D(64, (3, 3), activation='relu', padding='same'))
model.add(Conv2D(3, (3, 3), activation='sigmoid', padding='same'))
model.compile(optimizer='adam', loss='binary_crossentropy')
# 训练模型
model.fit(x=X_train_gray, y=X_train,
epochs=100,
batch_size=128,
shuffle=True,
validation_data=(X_test_gray, X_test))
# 使用模型进行预测
color_me = []
for i in X_test_gray:
i = np.repeat(i, 3, axis=2)
color_me.append(i)
color_me = np.array(color_me)
color_me = color_me.reshape(color_me.shape[0], color_me.shape[1], color_me.shape[2], color_me.shape[3])
output = model.predict(color_me)
# 显示预测结果
plt.figure(figsize=(20, 4))
for i in range(10):
# 显示原始图像
plt.subplot(2, 10, i+1)
plt.imshow(X_test[i])
plt.axis('off')
# 显示彩色化后的图像
plt.subplot(2, 10, i+11)
plt.imshow(output[i])
plt.axis('off')
plt.tight_layout()
plt.show()
```
在上面的代码中,我们首先加载CIFAR-10数据集,并将图像转换为灰度图像。然后我们将图像归一化,并将它们重塑为(32,32,1)的张量。接下来,我们定义了我们的自编码器模型,并使用反向传播算法对其进行训练。最后,我们使用模型对一些测试图像进行预测,并将预测结果显示出来。
这个模型的训练可能需要一些时间,具体时间取决于你的计算机性能和训练数据的大小。
阅读全文
相关推荐
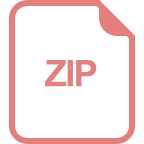
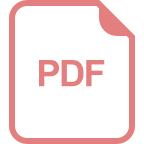
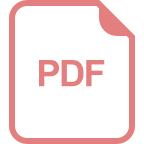
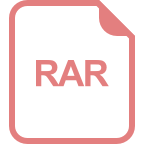
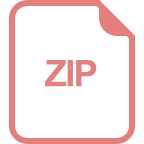
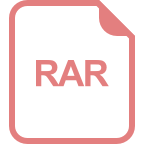
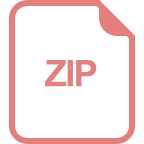
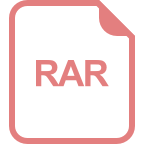
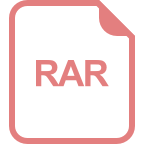
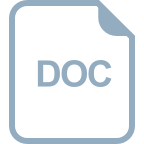
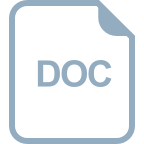
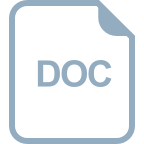
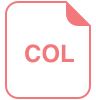
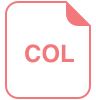
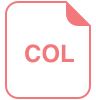
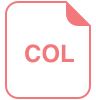