train_pred = {} test_pred = {} # 将NaN值用中位数填充 X_train = X_train.fillna(X_train.median()) X_test = X_test.fillna(X_train.median()) oof = np.zeros(X_train.shape[0]) prediction = np.zeros(X_test.shape[0]) fold = 5 skf = StratifiedKFold(n_splits=fold, random_state=2, shuffle=True) for index, (train_index, test_index) in enumerate(skf.split(X_train, y)): train_x, test_x, train_y, test_y = X_train[feature_name].iloc[train_index], X_train[feature_name].iloc[test_index], y.iloc[train_index], y.iloc[test_index] rf_model = RandomForestClassifier(**parameters) rf_model.fit(train_x, train_y) oof[test_index] = rf_model.predict_proba(test_x)[:, 1] prediction += rf_model.predict_proba(X_test)[:, 1] / fold del train_x, test_x, train_y, test_y gc.collect() train_pred['rf'] = oof test_pred['rf'] = prediction
时间: 2023-06-26 12:09:41 浏览: 77
这段代码使用了随机森林模型进行分类,首先将数据集中的缺失值用中位数填充。然后使用 StratifiedKFold 进行交叉验证,将数据集分成 5 折,每次使用其中 4 折数据作为训练集,1 折数据作为验证集,训练随机森林模型,并将模型在验证集上的输出保存到 oof 数组中。同时,在测试集上进行预测,并将预测输出保存到 prediction 数组中。最后将训练集和测试集的预测结果分别保存到 train_pred 和 test_pred 字典中,键名为 'rf' 表示使用随机森林模型。这里的 gc.collect() 是 Python 的垃圾回收机制,用于释放内存。
相关问题
y_train_pred = np.argmax(X_train.dot(w.T), axis=1) y_test_pred = np.argmax(X_test.dot(w.T), axis=1)加注释
```
# 对训练集进行预测,得到预测结果向量 y_train_pred
# 预测过程:将训练集数据 X_train 与训练好的权重矩阵 w 相乘,得到一个 (n_train, K) 的矩阵,其中第 i 行的 K 个数值表示第 i 个训练样本属于每个类别的概率
# 然后对每行取最大值所对应的索引,得到一个长度为 n_train 的向量,即为预测结果向量
y_train_pred = np.argmax(X_train.dot(w.T), axis=1)
# 对测试集进行预测,得到预测结果向量 y_test_pred
# 预测过程同上
y_test_pred = np.argmax(X_test.dot(w.T), axis=1)
```
这段代码对训练集和测试集进行了预测,得到了预测结果向量y_train_pred和y_test_pred。具体实现中,将训练集和测试集数据分别与训练好的权重矩阵w相乘,得到一个(n_train, K)和(n_test, K)的矩阵,其中第i行的K个数值表示第i个样本属于每个类别的概率。然后对每行取最大值所对应的索引,得到一个长度为n_train或n_test的向量,即为预测结果向量。最终,可以将预测结果向量与真实标签向量进行比较,评估分类模型的性能。
train_errors = [] test_errors = [] for lambda_val in lambdas: pipeline = Pipeline([ ('polynomial_features', PolynomialFeatures(degree=5)), ('ridge_regression', c.SGDLinearRegressor(batch_size=20, eta=0.01, tau_max=1000, epsilon=0.00001, lambda_=lambda_val, random_state=42))]) train_mse = 0 test_mse = 0 for i in range(10): X_train, y_train = c.generate_data(20) X_test, y_test = c.generate_data(1000) pipeline.fit(X_train.reshape(-1, 1), y_train) y_train_pred = pipeline.predict(X_train.reshape(-1, 1)) y_test_pred = pipeline.predict(X_test.reshape(-1, 1)) train_mse += mean_squared_error(y_train, y_train_pred) test_mse += mean_squared_error(y_test, y_test_pred) train_errors.append(train_mse / 10) test_errors.append(test_mse / 10)给这段代码加注释
下面是加了注释的代码:
```python
# 定义两个空列表,用于存储不同正则化参数下的训练误差和测试误差
train_errors = []
test_errors = []
# 遍历一系列正则化参数值
for lambda_val in lambdas:
# 定义管道对象,包含多项式特征转换和SGD线性回归模型
pipeline = Pipeline([
('polynomial_features', PolynomialFeatures(degree=5)),
('ridge_regression', c.SGDLinearRegressor(batch_size=20, eta=0.01, tau_max=1000,
epsilon=0.00001, lambda_=lambda_val, random_state=42))
])
# 定义训练误差和测试误差
train_mse = 0
test_mse = 0
# 对每个正则化参数值进行10次训练和测试
for i in range(10):
# 生成20个训练样本和对应标签,1000个测试样本和对应标签
X_train, y_train = c.generate_data(20)
X_test, y_test = c.generate_data(1000)
# 在训练集上拟合模型
pipeline.fit(X_train.reshape(-1, 1), y_train)
# 在训练集和测试集上进行预测
y_train_pred = pipeline.predict(X_train.reshape(-1, 1))
y_test_pred = pipeline.predict(X_test.reshape(-1, 1))
# 计算并累加训练误差和测试误差
train_mse += mean_squared_error(y_train, y_train_pred)
test_mse += mean_squared_error(y_test, y_test_pred)
# 将每个正则化参数值下的训练误差和测试误差除以10,并存储到对应的列表中
train_errors.append(train_mse / 10)
test_errors.append(test_mse / 10)
```
注释中解释了代码的每个部分的作用和功能,包括定义列表、遍历正则化参数、定义管道对象、生成数据、拟合模型、预测,以及计算训练误差和测试误差。通过注释,可以更加清晰地理解代码的功能和执行流程。
相关推荐
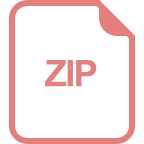












