利用决策树算法对鸢尾花数据进行建模分类,用graphviz可视化算法的过程与结合准确率、ROC曲线,F1分数,混淆矩阵,召回率,精确率等结果指标,代码展示加解释
时间: 2024-09-23 22:13:55 浏览: 28
利用决策树算法对鸢尾花数据集进行分类是一个常见的机器学习实践,我们可以使用Python的scikit-learn库来完成这个任务。以下是整个流程的概述:
首先,我们需要导入所需的库:
```python
import numpy as np
import pandas as pd
from sklearn.datasets import load_iris
from sklearn.model_selection import train_test_split
from sklearn.tree import DecisionTreeClassifier
from sklearn.metrics import accuracy_score, roc_auc_score, f1_score, confusion_matrix, recall_score, precision_score
import graphviz
```
然后加载鸢尾花数据并准备数据:
```python
iris = load_iris()
X = iris.data
y = iris.target
# 分割数据为训练集和测试集
X_train, X_test, y_train, y_test = train_test_split(X, y, test_size=0.2, random_state=42)
```
接着,创建决策树模型并拟合数据:
```python
tree_model = DecisionTreeClassifier(random_state=42)
tree_model.fit(X_train, y_train)
```
预测测试集结果,并计算评估指标:
```python
y_pred = tree_model.predict(X_test)
accuracy = accuracy_score(y_test, y_pred)
roc_auc = roc_auc_score(y_test, tree_model.predict_proba(X_test)[:, 1])
f1 = f1_score(y_test, y_pred, average='macro')
conf_mat = confusion_matrix(y_test, y_pred)
recall = recall_score(y_test, y_pred, average='macro')
precision = precision_score(y_test, y_pred, average='macro')
print(f"Accuracy: {accuracy}")
print(f"ROC AUC Score: {roc_auc}")
print(f"F1 Score: {f1}")
print("Confusion Matrix:")
print(conf_mat)
print(f"Recall: {recall}")
print(f"Precision: {precision}")
```
为了可视化决策树,我们使用`sklearn.tree.export_graphviz`函数和Graphviz库:
```python
dot_data = tree_model.export_graphviz(tree_model, out_file=None,
feature_names=iris.feature_names,
class_names=iris.target_names,
filled=True, rounded=True,
special_characters=True)
graph = graphviz.Source(dot_data)
graph.view()
```
这将生成一个可视化的决策树图。
相关推荐
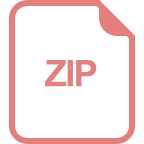
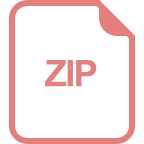
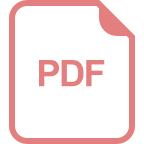














