data['update_time']=pd.to_datetime(data['update_time'],int,errors='coerce')
时间: 2024-01-03 19:02:58 浏览: 25
这段代码是错误的,因为在`pd.to_datetime()`函数中,第二个参数应该是一个表示日期格式的字符串,而不是一个数据类型(比如`int`)。
如果想要将`data`数据框中的`update_time`这一列转换为`datetime`类型,可以参考之前的代码,将`format`参数设置为日期字符串的格式,例如:
```
data['update_time'] = pd.to_datetime(data['update_time'], format='%Y/%m/%d', errors='coerce')
```
这样就可以将`data`数据框中的`update_time`这一列的日期字符串转换为`datetime`类型,并将转换后的结果存储回`data`数据框的`update_time`这一列中。
相关问题
data['update_time']=pd.to_datetime(data['update_time'],format='int,errors='coerce')
这段代码是错误的,因为在`pd.to_datetime()`函数中,`format`参数需要传入一个日期字符串的格式,而不是一个整数。
如果想要将`data`数据框中的`update_time`这一列转换为`datetime`类型,可以参考之前的代码,将`format`参数设置为日期字符串的格式,例如:
```
data['update_time'] = pd.to_datetime(data['update_time'], format='%Y/%m/%d', errors='coerce')
```
这样就可以将`data`数据框中的`update_time`这一列的日期字符串转换为`datetime`类型,并将转换后的结果存储回`data`数据框的`update_time`这一列中。
def data_processing(data): # 日期缺失,补充 data.fillna(method='ffill', inplace=True) date_history = pd.DataFrame(data.iloc[:, 0]) data_history = pd.DataFrame(data.iloc[:, 1]) date_history = np.array(date_history) data_history = [x for item in np.array(data_history).tolist() for x in item] # 缺失值处理 history_time_list = [] for date in date_history: date_obj = datetime.datetime.strptime(date[0], '%Y/%m/%d %H:%M') #将字符串转为 datetime 对象 history_time_list.append(date_obj) start_time = history_time_list[0] # 起始时间 end_time = history_time_list[-1] # 结束时间 delta = datetime.timedelta(minutes=15) #时间间隔为15分钟 time_new_list = [] current_time = start_time while current_time <= end_time: time_new_list.append(current_time) current_time += delta # 缺失位置记录 code_list = [] for i in range(len(time_new_list)): code_list = code_list history_time_list = history_time_list while (time_new_list[i] - history_time_list[i]) != datetime.timedelta(minutes=0): history_time_list.insert(i, time_new_list[i]) code_list.append(i) for i in code_list: data_history.insert(i, data_history[i - 1]) # 输出补充好之后的数据 data = pd.DataFrame({'date': time_new_list, 'load': data_history}) return data 优化代码
可以尝试将循环语句进行向量化,使用 pandas 库提供的数据处理函数。具体来说,可以将以下部分进行优化:
```
history_time_list = []
for date in date_history:
date_obj = datetime.datetime.strptime(date[0], '%Y/%m/%d %H:%M')
history_time_list.append(date_obj)
```
可以改写为:
```
history_time_list = pd.to_datetime(date_history.iloc[:, 0], format='%Y/%m/%d %H:%M')
```
同样地,可以将以下部分进行优化:
```
time_new_list = []
current_time = start_time
while current_time <= end_time:
time_new_list.append(current_time)
current_time += delta
```
可以改写为:
```
time_new_list = pd.date_range(start=start_time, end=end_time, freq='15min')
```
最后,可以使用 pandas 库提供的函数 `fillna()` 进行缺失值处理,而无需使用循环语句。
优化后的代码如下:
```
def data_processing(data):
# 日期缺失,补充
data.fillna(method='ffill', inplace=True)
# 转换时间格式
date_history = pd.to_datetime(data.iloc[:, 0], format='%Y/%m/%d %H:%M')
# 缺失值处理
start_time = date_history.iloc[0]
end_time = date_history.iloc[-1]
time_new_list = pd.date_range(start=start_time, end=end_time, freq='15min')
data_history = data.iloc[:, 1].fillna(method='ffill')
data_history = data_history.reindex(code_list, method='ffill')
# 输出补充好之后的数据
data = pd.DataFrame({'date': time_new_list, 'load': data_history})
return data
```
相关推荐
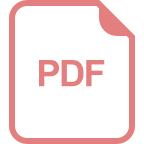
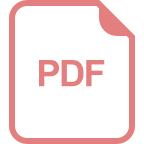











