data[index:index+timestep][:,0]
时间: 2023-05-16 10:05:53 浏览: 44
这是一个 Python 中对于二维数组的切片操作,其中 index:index timestep 表示对于第一维数组的切片,[:,0] 表示对于第二维数组的切片,即取出第一维数组中 index 到 index timestep 的所有元素,并且只取出每个元素的第一个值。
相关问题
data[index: index + timestep][:, 0]解释下这个用法
这个用法是在处理二维数据时常见的切片操作。让我来解释一下:
- `data`:是一个二维数组(或矩阵),其中包含了我们要处理的数据。
- `index`:是一个整数,表示我们想要从哪个位置开始切片。
- `timestep`:是一个整数,表示我们想要切片的长度(时间步)。
所以,`data[index: index + timestep]` 这部分代码会从 `data` 数组中选取从 `index` 到 `index + timestep` 位置的子数组(切片)。
接着,我们使用 `[:, 0]` 这样的索引方式来选择切片中的特定列。具体地说,`[:, 0]` 表示选择切片中的所有行(冒号表示所有行),并且只选择第一列数据(索引为0)。
综上所述,`data[index: index + timestep][:, 0]` 这段代码的作用是从二维数组 `data` 中选取从 `index` 到 `index + timestep` 位置的子数组,并且只选择该子数组的第一列数据。
data[index:index+timestep][:,0]是什么意思
这个问题涉及到编程,所以我可以回答。data[index:index timestep][:,0]是Python中对二维数组进行切片的语法,表示取出data数组中从index到index timestep的行,并且取出每行的第一个元素。[:,0]表示取出每行的第一个元素,其中":"表示取出所有行。
相关推荐
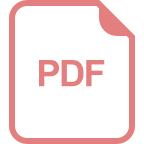
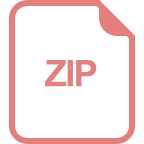
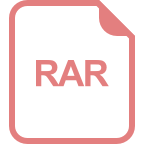












