GCN,我希望输入是一张图,这张图包括若干节点和边,这个图整体有一个标签,也就是说所有的节点和边对应的都是同一个标签,并且每个节点、每个边还有自己的属性。给出代码示例
时间: 2024-02-03 20:13:07 浏览: 73
以下是一个简单的 GCN 模型的代码示例,用于对输入图进行节点分类:
```python
import torch
import torch.nn.functional as F
from torch_geometric.nn import GCNConv
class GCN(torch.nn.Module):
def __init__(self, num_features, hidden_size, num_classes):
super(GCN, self).__init__()
# GCN layer
self.conv1 = GCNConv(num_features, hidden_size)
self.conv2 = GCNConv(hidden_size, num_classes)
def forward(self, x, edge_index):
# Apply GCN layer 1
x = self.conv1(x, edge_index)
x = F.relu(x)
# Apply GCN layer 2
x = self.conv2(x, edge_index)
# Compute softmax over the output logits
x = F.log_softmax(x, dim=1)
return x
```
在这个示例中,`num_features` 表示每个节点的属性数量,`hidden_size` 表示 GCN 层的隐藏层大小,`num_classes` 表示输出的类别数量。输入的图由节点特征张量 `x` 和边索引张量 `edge_index` 组成。节点特征张量 `x` 的形状为 `(num_nodes, num_features)`,表示每个节点的属性。边索引张量 `edge_index` 的形状为 `(2, num_edges)`,表示每条边连接的两个节点的索引。输出是一个形状为 `(num_nodes, num_classes)` 的张量,其中每行是一个节点的分类结果的概率分布。
在训练过程中,可以将输入的图转化为 PyTorch Geometric 中的 `Data` 对象:
```python
from torch_geometric.data import Data
# Define the input graph
x = torch.randn(num_nodes, num_features)
edge_index = torch.tensor(edge_index, dtype=torch.long)
# Create a PyTorch Geometric Data object
data = Data(x=x, edge_index=edge_index, y=y)
```
其中 `y` 是形状为 `(num_nodes,)` 的张量,表示每个节点的标签。然后,可以使用 `torch_geometric.data.DataLoader` 类来加载训练数据:
```python
from torch_geometric.data import DataLoader
# Define the training data loader
train_loader = DataLoader(train_data, batch_size=batch_size, shuffle=True)
```
在每个训练迭代中,可以使用以下代码执行前向传播和反向传播:
```python
# Forward pass
out = model(data.x, data.edge_index)
# Compute the loss
loss = F.nll_loss(out[train_mask], data.y[train_mask])
# Backward pass
optimizer.zero_grad()
loss.backward()
optimizer.step()
```
阅读全文
相关推荐
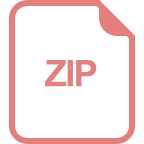

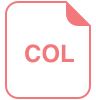



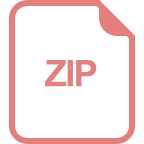
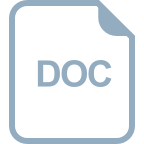
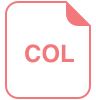







