The current study proposes a new MATLAB code called MSDCBE able to calculate DCBs of GPS satellites and receivers. This code was compared with two other codes and evaluated using IAAC data and, from all the above, we can conclude the following. 1. The estimated DCB results are affected by using a weight function according to satellite elevation angle observations. In addition, results show good agreement with IGS, CODE, and JPL results than using multistation estimation DCB without a weight function. 2. When using multi-station DCB estimation, the number of input stations influences the DCB results. However, it is recommended to enlarge the size of the used network, but it needs high computer requirements and much more analysis time (only one station has more than 20 000 observations per a day). 3. The most effective factor in DCB estimation is using a multi-station network instead of a single station that appeared from results which improved from 1.1866 and 0.7982 ns maximum DCB mean differences for M_DCB and ZDDCBE single-station analysis to 0.1477 ns for MSDCBE. So, using multi-station network DCB estimation – if available – is strongly recommended.
时间: 2024-04-27 16:22:26 浏览: 8
本研究提出了一种名为MSDCBE的新的MATLAB代码,能够计算GPS卫星和接收机的DCB。该代码与其他两个代码进行了比较,并使用IAAC数据进行了评估,从上述结果可以得出以下结论:
1. 使用根据卫星高度角观测值的加权函数影响估计的DCB结果。此外,结果显示,与使用没有加权函数的多站估计DCB相比,与IGS、CODE和JPL结果的一致性更好。
2. 在使用多站DCB估计时,输入站点的数量会影响DCB结果。然而,建议扩大使用的网络规模,但这需要较高的计算机要求和更多的分析时间(每天仅一个站点就有超过20,000个观测数据)。
3. DCB估计中最有效的因素是使用多站网络,而不是单个站点,这从结果中表现出来,M_DCB和ZDDCBE单站分析的最大DCB平均差异从1.1866和0.7982 ns提高到了0.1477 ns的MSDCBE。因此,强烈建议使用多站网络DCB估计(如果可用)。
相关问题
Another example is the SRIOV_NET_VF resource class, which is provided by SRIOV-enabled network interface cards. In the case of multiple SRIOV-enabled NICs on a compute host, different qualitative traits may be tagged to each NIC. For example, the NIC called enp2s0 might have a trait “CUSTOM_PHYSNET_PUBLIC” indicating that the NIC is attached to a physical network called “public”. The NIC enp2s1 might have a trait “CUSTOM_PHYSNET_INTRANET” that indicates the NIC is attached to the physical network called “Intranet”. We need a way of representing that these NICs each provide SRIOV_NET_VF resources but those virtual functions are associated with different physical networks. In the resource providers data modeling, the entity which is associated with qualitative traits is the resource provider object. Therefore, we require a way of representing that the SRIOV-enabled NICs are themselves resource providers with inventories of SRIOV_NET_VF resources. Those resource providers are contained on a compute host which is a resource provider that has inventory records for other types of resources such as VCPU, MEMORY_MB or DISK_GB. This spec proposes that nested resource providers be created to allow for distinguishing details of complex components of some resource providers. During review the question came up about “rolling up” amounts of these nested providers to the root level. Imagine this scenario: I have a NIC with two PFs, each of which has only 1 VF available, and I get a request for 2 VFs without any traits to distinguish them. Since there is no single resource provider that can satisfy this request, it will not select this root provider, even though the root provider “owns” 2 VFs. This spec does not propose any sort of “rolling up” of inventory, but this may be something to consider in the future. If it is an idea that has support, another BP/spec can be created then to add this behavior.
另一个例子是SRIOV_NET_VF资源类,它由支持SRIOV的网络接口卡提供。在计算主机上存在多个支持SRIOV的网卡的情况下,可以对每个网卡标记不同的定性特征。例如,名为enp2s0的网卡可能具有一个名为“CUSTOM_PHYSNET_PUBLIC”的特征,表示该网卡连接到名为“public”的物理网络。名为enp2s1的网卡可能具有一个名为“CUSTOM_PHYSNET_INTRANET”的特征,表示该网卡连接到名为“Intranet”的物理网络。我们需要一种表示这些网卡都提供SRIOV_NET_VF资源,但这些虚拟函数与不同的物理网络相关联的方式。在资源提供者的数据建模中,与定性特征相关联的实体是资源提供者对象。因此,我们需要一种表示支持SRIOV的网卡本身是具有SRIOV_NET_VF资源库存的资源提供者的方式。这些资源提供者包含在计算主机上,计算主机本身是一个资源提供者,具有其他类型资源(如VCPU、MEMORY_MB或DISK_GB)的库存记录。
该规范提议创建嵌套资源提供者,以区分某些资源提供者的复杂组件的详细信息。在审查过程中,出现了一个问题,即如何将这些嵌套提供者的数量“合并”到根级别。想象这样的情景:我有一张网卡,其中有两个物理功能(PFs),每个PF只有1个可用的虚拟功能(VF),我收到一个请求需要2个VF,并且没有任何可区分它们的特征。由于没有单个资源提供者能够满足此请求,它将不会选择此根提供者,即使根提供者“拥有”2个VF。该规范没有提议对库存进行任何形式的“合并”,但这可能是将来要考虑的事情。如果这是一个得到支持的想法,可以创建另一个BP/spec来添加此行为。
Please revise the paper:Accurate determination of bathymetric data in the shallow water zone over time and space is of increasing significance for navigation safety, monitoring of sea-level uplift, coastal areas management, and marine transportation. Satellite-derived bathymetry (SDB) is widely accepted as an effective alternative to conventional acoustics measurements over coastal areas with high spatial and temporal resolution combined with extensive repetitive coverage. Numerous empirical SDB approaches in previous works are unsuitable for precision bathymetry mapping in various scenarios, owing to the assumption of homogeneous bottom over the whole region, as well as the limitations of constructing global mapping relationships between water depth and blue-green reflectance takes no account of various confounding factors of radiance attenuation such as turbidity. To address the assumption failure of uniform bottom conditions and imperfect consideration of influence factors on the performance of the SDB model, this work proposes a bottom-type adaptive-based SDB approach (BA-SDB) to obtain accurate depth estimation over different sediments. The bottom type can be adaptively segmented by clustering based on bottom reflectance. For each sediment category, a PSO-LightGBM algorithm for depth derivation considering multiple influencing factors is driven to adaptively select the optimal influence factors and model parameters simultaneously. Water turbidity features beyond the traditional impact factors are incorporated in these regression models. Compared with log-ratio, multi-band and classical machine learning methods, the new approach produced the most accurate results with RMSE value is 0.85 m, in terms of different sediments and water depths combined with in-situ observations of airborne laser bathymetry and multi-beam echo sounder.
回答:本文提出了一种基于底部类型自适应的卫星深度测量(BA-SDB)方法,以解决传统卫星深度测量模型中假设底部均匀的局限性以及不能充分考虑影响反射率的干扰因素,如浊度等。通过基于聚类的底部反射率自适应分割,并采用PSO-LightGBM算法考虑多种因素,实现了对不同沉积物和水深的准确深度估算,与空中激光测深和多波束回声深度声纳联合实测结果相比,均方根误差值达到0.85m,是传统比值法、多波段法和机器学习方法中最准确的方法。
相关推荐
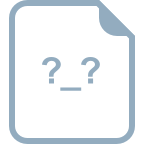
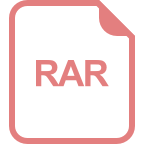
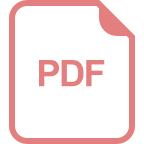












