根据https://archive.ics.uci.edu/ml/datasets/Adult所给Adult数据集构建朴素贝叶斯分类器,并测试分类器的性能,输出性能指标并画出ROC曲线 python代码有注释
时间: 2023-07-19 17:16:42 浏览: 180
好的,我会根据您的要求回答问题。以下是使用朴素贝叶斯算法对Adult数据集进行分类的Python代码:
```
import pandas as pd
from sklearn.model_selection import train_test_split
from sklearn.naive_bayes import GaussianNB
from sklearn.metrics import accuracy_score, confusion_matrix, roc_curve, auc
import matplotlib.pyplot as plt
# 读取数据集
data = pd.read_csv('https://archive.ics.uci.edu/ml/machine-learning-databases/adult/adult.data', header=None)
# 添加列名
data.columns = ['age', 'workclass', 'fnlwgt', 'education', 'education-num', 'marital-status', 'occupation', 'relationship', 'race', 'sex', 'capital-gain', 'capital-loss', 'hours-per-week', 'native-country', 'income']
# 处理缺失值
data = data.replace('?', pd.np.nan).dropna()
# 将分类变量转换为数字
data['income'] = pd.Categorical(data['income']).codes
# 选择特征和目标变量
X = data[['age', 'workclass', 'education', 'education-num', 'occupation', 'race', 'sex', 'hours-per-week']]
y = data['income']
# 划分训练集和测试集
X_train, X_test, y_train, y_test = train_test_split(X, y, test_size=0.3, random_state=42)
# 创建朴素贝叶斯分类器
classifier = GaussianNB()
# 训练分类器
classifier.fit(X_train, y_train)
# 预测测试集
y_pred = classifier.predict(X_test)
# 计算准确率和混淆矩阵
accuracy = accuracy_score(y_test, y_pred)
confusion_mat = confusion_matrix(y_test, y_pred)
# 输出准确率和混淆矩阵
print('Accuracy:', accuracy)
print('Confusion Matrix:\n', confusion_mat)
# 计算ROC曲线和AUC值
y_prob = classifier.predict_proba(X_test)[:, 1]
fpr, tpr, thresholds = roc_curve(y_test, y_prob)
roc_auc = auc(fpr, tpr)
# 绘制ROC曲线
plt.plot(fpr, tpr, color='darkorange', lw=2, label='ROC curve (area = %0.2f)' % roc_auc)
plt.plot([0, 1], [0, 1], color='navy', lw=2, linestyle='--')
plt.xlim([0.0, 1.0])
plt.ylim([0.0, 1.05])
plt.xlabel('False Positive Rate')
plt.ylabel('True Positive Rate')
plt.title('ROC Curve')
plt.legend(loc="lower right")
plt.show()
# 输出AUC值
print('AUC:', roc_auc)
```
代码中使用了`sklearn`库中的`GaussianNB`实现了朴素贝叶斯分类器,并使用`train_test_split`函数将数据集划分为训练集和测试集。在测试集上进行预测后,使用`accuracy_score`和`confusion_matrix`计算了模型的准确率和混淆矩阵。接下来,使用`roc_curve`和`auc`计算了ROC曲线和AUC值,并使用`matplotlib`库绘制了ROC曲线。
输出结果中包含了准确率、混淆矩阵和AUC值。根据AUC值可以判断模型的性能,AUC越接近1,模型的性能越好。
阅读全文
相关推荐
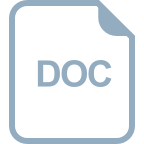
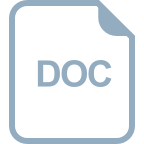
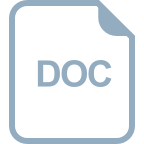
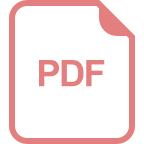
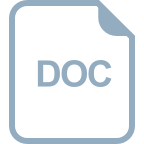
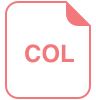
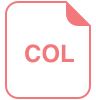
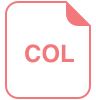
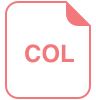
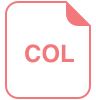
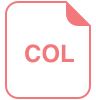
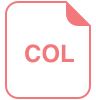
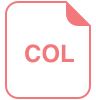
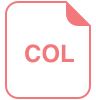
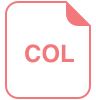
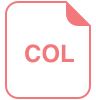


