如何定义一个使用了残差块(ResNet Block)作为基本构建单元的GRU网络
时间: 2024-12-17 22:21:49 浏览: 11
在定义一个使用残差块(ResNet Block)作为基础构建单元的GRU(Gated Recurrent Unit)网络时,实际上并没有直接将ResNet块的概念应用到RNN(Recurrent Neural Network)结构上,因为GRU和ResNet都是不同的网络架构,GRU主要用于序列数据处理,而ResNet则是用于图像识别。然而,如果你想要在某个上下文中融合这两种思想,可以尝试创造一种“启发式”设计,比如对GRU单元做一些类似残差连接的操作,让信息更好地流动。
尽管如此,这并不是标准的做法,但在某些特定任务中可能会有创新性的尝试。下面是一个简化示例,展示如何在GRU的基础上引入类似于残差块的思想,但请注意这并不是通常意义上的ResNet Block应用:
```python
import torch
import torch.nn as nn
# 创建一个自定义的GRU Cell with Residual Connection
class GRUResNetBlock(nn.Module):
def __init__(self, hidden_size):
super(GRUResNetBlock, self).__init__()
self.hidden_size = hidden_size
self.gru_cell = nn.GRUCell(hidden_size, hidden_size)
self.res_connection = nn.Linear(hidden_size, hidden_size)
def forward(self, input, prev_hidden):
# 基于GRU单元的正常计算
next_hidden = self.gru_cell(input, prev_hidden)
# 添加残差连接
residual = input if prev_hidden is None else prev_hidden
residual = self.res_connection(residual)
next_hidden += residual
return next_hidden, next_hidden
# 然后你可以像这样在RNN层中堆叠GRUResNetBlock
class ResNetBasedGRU(nn.Module):
def __init__(self, input_size, num_layers, hidden_size):
super().__init__()
self.input_size = input_size
self.num_layers = num_layers
self.hidden_size = hidden_size
self.rnn_layers = nn.ModuleList([GRUResNetBlock(hidden_size) for _ in range(num_layers)])
def forward(self, input_seq, initial_hidden=None):
hiddens = []
for layer in self.rnn_layers:
output, hidden = layer(input_seq, hidden)
hiddens.append(output)
input_seq = output # 这里假设每一层的输出都会进入下一层的输入
return torch.stack(hiddens), output
```
在这个例子中,我们并没有真正地将ResNet Block的设计应用到GRU的循环结构,而是仅在GRU单元内部添加了简单的线性转换作为残差连接。实际应用中,这样的做法需要根据具体任务和数据特点来评估是否有效。
阅读全文
相关推荐
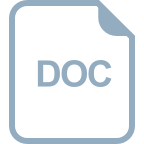
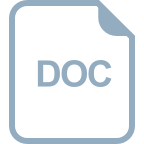
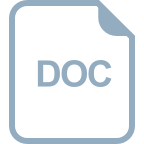
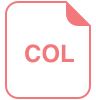
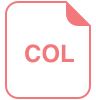
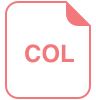
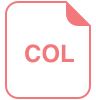
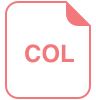
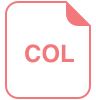

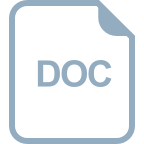
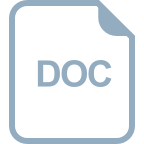
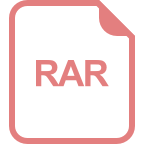
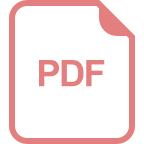
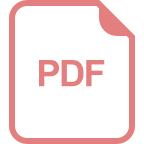
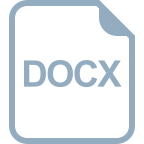
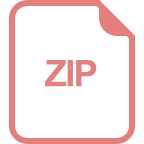