deeplabv3安装教程
时间: 2024-04-21 21:21:44 浏览: 125
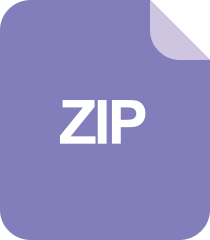
DeepLabv3FineTuning:在您的PyTorch中为自己的细分任务微调DeepLabv3细分网络的教程
DeepLabv3是一种用于语义分割任务的深度学习模型,它在图像中对每个像素进行分类,从而实现像素级别的语义理解。下面是DeepLabv3的安装教程:
1. 确保你已经安装了Python和pip,并且已经配置好了相应的环境变量。
2. 创建一个新的虚拟环境(可选但推荐),可以使用conda或者virtualenv等工具。
3. 在命令行中使用以下命令安装TensorFlow和其他依赖项:
```
pip install tensorflow
pip install numpy
pip install matplotlib
```
4. 下载DeepLabv3的源代码,可以从GitHub上找到官方的代码仓库。
5. 解压下载的源代码,并进入解压后的文件夹。
6. 在命令行中使用以下命令安装DeepLabv3所需的其他依赖项:
```
pip install -r requirements.txt
```
7. 下载预训练的模型权重文件,可以从官方网站或者其他可靠的来源获取。
8. 将下载的模型权重文件放入DeepLabv3源代码文件夹中。
9. 使用命令行进入DeepLabv3源代码文件夹,并执行以下命令运行DeepLabv3:
```
python deeplab.py
```
10. 根据需要进行配置和调整,例如输入图像路径、输出结果路径、模型参数等。
以上是DeepLabv3的基本安装教程,希望对你有帮助!
阅读全文
相关推荐
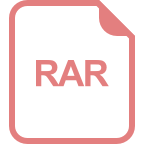
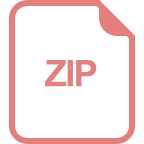
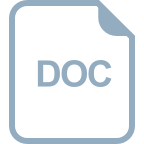

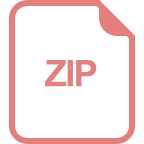
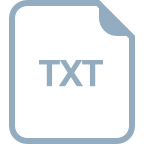
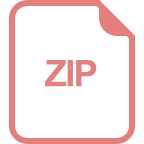

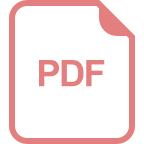
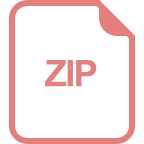
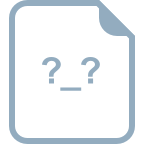
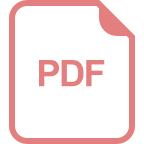
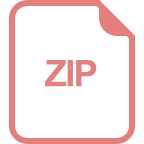
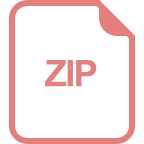
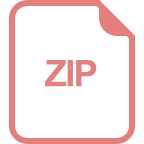
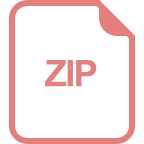