已知ANN网络中某组神经元连接权重为 w=[0.5, 1.0, 2.0],偏置b=[-1.0, 0.0, 1.0],输入 X=np.linspace(-10, 10, 100),,输出V
时间: 2024-12-13 17:27:06 浏览: 3
在Artificial Neural Network (ANN) 中,给定一组神经元的连接权重 \( w \) 和偏置 \( b \),计算输出 \( V \) 的过程通常涉及到激活函数的使用。对于线性模型来说,每个神经元的输出是其输入信号与权重的乘积加上偏置项,然后可能会通过非线性的激活函数如Sigmoid、ReLU等进行转换。
假设这里采用的是简单的线性激活函数,即没有使用额外的激活函数,那么输出 \( V \) 可以按照下面的方式计算:
1. 首先将输入数组 \( X \) 与权重向量 \( w \) 进行逐元素相乘,形成一个新的矩阵。
2. 然后将这个结果与偏置向量 \( b \) 相加。这一步可以用 Python 数学运算表示为:
```python
weighted_sum = np.dot(X, w) + b
```
由于没有激活函数,输出 \( V \) 就等于这个加权求和的结果,即 \( V = weighted_sum \)。
如果需要考虑激活函数的影响,例如 Sigmoid 函数 \( f(x) = \frac{1}{1+e^{-x}} \),则每个神经元的输出会取该值。在这种情况下,输出 \( V \) 应该是个数组,每个元素都是相应输入经过激活函数后的值。
相关问题
已知y依赖于x,数据如下: x 0.0 0.5 1.0 1.5 1.9 2.5 3.0 3.5 4.0 4.5 5.0 5.5 6.0 6.6 7.0 7.6 8.5 9.0 10 y 1.0 0.9 0.7 1.5 2.0 2.4 3.2 2.0 2.7 3.5 1.0 4.0 3.6 2.7 5.7 4.6 6.0 6.8 7.3 用内置函数fit求其1次、3次、5次拟合多项式,并分析误差。
首先需要导入numpy和matplotlib模块:
```
import numpy as np
import matplotlib.pyplot as plt
```
然后将数据转换为numpy数组:
```
x = np.array([0.0, 0.5, 1.0, 1.5, 1.9, 2.5, 3.0, 3.5, 4.0, 4.5, 5.0, 5.5, 6.0, 6.6, 7.0, 7.6, 8.5, 9.0, 10])
y = np.array([1.0, 0.9, 0.7, 1.5, 2.0, 2.4, 3.2, 2.0, 2.7, 3.5, 1.0, 4.0, 3.6, 2.7, 5.7, 4.6, 6.0, 6.8, 7.3])
```
1次拟合多项式:
```
p1 = np.polyfit(x, y, 1)
y1 = np.polyval(p1, x)
```
3次拟合多项式:
```
p3 = np.polyfit(x, y, 3)
y3 = np.polyval(p3, x)
```
5次拟合多项式:
```
p5 = np.polyfit(x, y, 5)
y5 = np.polyval(p5, x)
```
计算误差:
```
error1 = np.sum((y - y1) ** 2)
error3 = np.sum((y - y3) ** 2)
error5 = np.sum((y - y5) ** 2)
```
将结果绘制成图表:
```
plt.plot(x, y, 'o')
plt.plot(x, y1, label='1次拟合')
plt.plot(x, y3, label='3次拟合')
plt.plot(x, y5, label='5次拟合')
plt.legend()
plt.show()
```
最后输出每种拟合的误差:
```
print("1次拟合误差:", error1)
print("3次拟合误差:", error3)
print("5次拟合误差:", error5)
```
完整代码如下:
```
import numpy as np
import matplotlib.pyplot as plt
# 数据
x = np.array([0.0, 0.5, 1.0, 1.5, 1.9, 2.5, 3.0, 3.5, 4.0, 4.5, 5.0, 5.5, 6.0, 6.6, 7.0, 7.6, 8.5, 9.0, 10])
y = np.array([1.0, 0.9, 0.7, 1.5, 2.0, 2.4, 3.2, 2.0, 2.7, 3.5, 1.0, 4.0, 3.6, 2.7, 5.7, 4.6, 6.0, 6.8, 7.3])
# 拟合
p1 = np.polyfit(x, y, 1)
y1 = np.polyval(p1, x)
p3 = np.polyfit(x, y, 3)
y3 = np.polyval(p3, x)
p5 = np.polyfit(x, y, 5)
y5 = np.polyval(p5, x)
# 计算误差
error1 = np.sum((y - y1) ** 2)
error3 = np.sum((y - y3) ** 2)
error5 = np.sum((y - y5) ** 2)
# 绘图
plt.plot(x, y, 'o')
plt.plot(x, y1, label='1次拟合')
plt.plot(x, y3, label='3次拟合')
plt.plot(x, y5, label='5次拟合')
plt.legend()
plt.show()
# 输出误差
print("1次拟合误差:", error1)
print("3次拟合误差:", error3)
print("5次拟合误差:", error5)
```
阅读全文
相关推荐
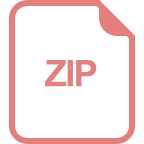
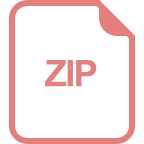
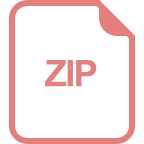
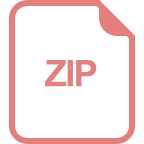
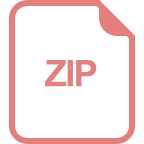
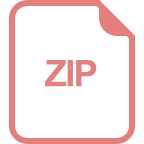
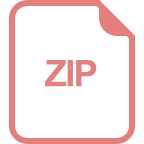
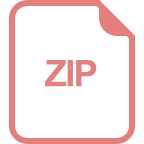
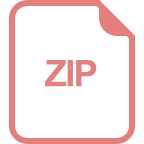
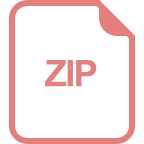
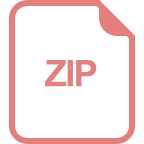
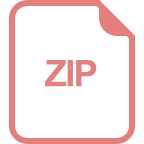
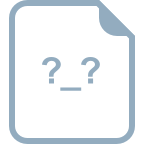
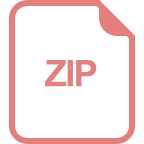
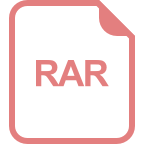
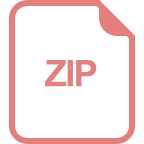
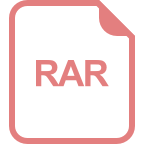
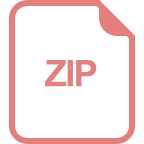