在python中加载ImageNet数据库
时间: 2024-10-21 08:03:24 浏览: 24
在Python中加载ImageNet数据库通常涉及到几个步骤:
1. **安装必要的库**:首先确保你已经安装了像`torch`(对于PyTorch用户)或者`tensorflow`(对于TensorFlow用户)这样的深度学习库,它们都包含了处理ImageNet数据集的功能。
2. **下载预处理数据**:由于原始ImageNet数据集非常大,官方并没有直接提供预处理后的版本供直接加载。你可以在Kaggle或者其他地方找到已经分好类的ImageNet数据集,如ILSVRC 2012或ImageNet_LT等。
3. **数据加载**:
- PyTorch:使用`torchvision.datasets.ImageFolder`,需要指定数据集的路径,以及是否转换为Tensor:
```python
from torchvision import datasets, transforms
transform = transforms.Compose([transforms.Resize(256), transforms.CenterCrop(224), transforms.ToTensor(), transforms.Normalize(mean=[0.485, 0.456, 0.406], std=[0.229, 0.224, 0.225])])
train_data = datasets.ImageFolder('path_to_train_folder', transform=transform)
```
- TensorFlow:可以使用`tf.keras.preprocessing.image_dataset_from_directory`类似的方法,示例如下:
```python
from tensorflow.keras.preprocessing.image import ImageDataGenerator
datagen = ImageDataGenerator(rescale=1./255)
train_generator = datagen.flow_from_directory('path_to_train_folder', target_size=(224, 224))
```
4. **创建批次和迭代器**:将数据集划分为训练批次,并设置合适的批大小和数据增强选项(如随机翻转、裁剪等)。
阅读全文
相关推荐
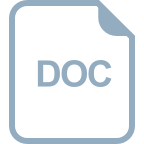
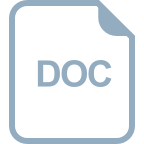
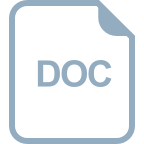
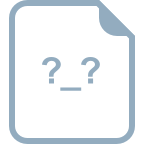
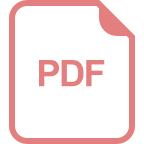
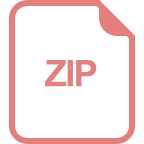
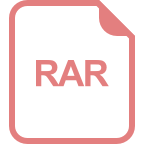
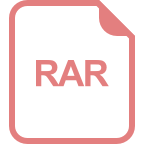
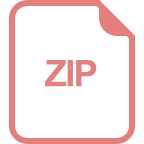
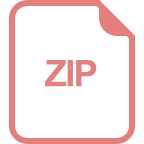
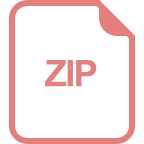
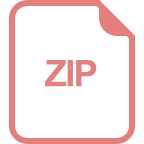
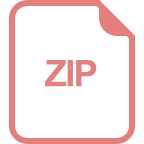
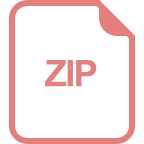
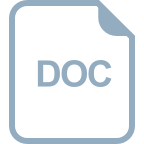
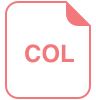
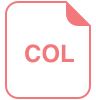
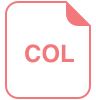
