pytorch中lstm时间序列预测
时间: 2023-11-05 19:59:24 浏览: 255
在PyTorch中,可以使用LSTM算法进行时间序列预测。首先,您需要准备时间序列数据,并将其转换为模型所需的格式。然后,您可以定义并训练一个LSTM模型。在训练过程中,模型将学习时间序列数据的模式,并使用这些模式来预测未来的值。最后,您可以使用模型对新的时间序列数据进行预测。
下面是使用PyTorch实现LSTM时间序列预测的一般步骤:
1. 准备数据: 将时间序列数据划分为输入序列和目标序列。输入序列由之前的时间步组成,而目标序列是在输入序列之后的一个时间步。确保数据的维度和类型适合LSTM模型。
2. 定义模型: 使用PyTorch的nn.LSTM类定义一个LSTM模型。指定输入维度、隐藏状态的维度和层数等参数。
3. 定义损失函数: 选择适当的损失函数来度量模型预测值与真实值之间的差异。常见的选择是均方误差(MSE)损失函数。
4. 定义优化器: 选择适当的优化器来更新模型的参数。常见的选择是随机梯度下降(SGD)或Adam优化器。
5. 训练模型: 使用训练数据对模型进行训练。在每个时期中,将输入序列提供给模型,得到预测结果,并计算损失。然后使用反向传播算法更新模型的参数。
6. 预测未来值: 使用训练好的模型对新的时间序列数据进行预测。将输入序列提供给模型,得到预测结果。
相关问题
基于pytorch的lstm时间序列预测
首先,你需要准备好你的数据集。数据集应该包括时间序列数据和相应的标签。接下来,你需要将数据集分为训练集和测试集。
然后,你需要定义你的LSTM模型。在PyTorch中,你可以使用`nn.LSTM`模块来定义LSTM网络。你需要指定输入特征的数量、隐藏层的数量和输出特征的数量。
接下来,你需要定义损失函数和优化器。对于时间序列预测,通常使用均方误差(MSE)损失函数。你可以使用`nn.MSELoss`模块来定义MSE损失函数。对于优化器,你可以使用`optim.Adam`模块来定义Adam优化器。
然后,你需要编写训练代码。在每个epoch中,你需要将数据输入到LSTM模型中,并计算损失。然后,你需要使用优化器来更新模型参数。
最后,你需要编写测试代码。在测试中,你需要将测试数据输入到LSTM模型中,并使用训练好的模型计算预测值。然后,你可以使用预测值和实际值之间的MSE来评估模型的性能。
下面是一个基于PyTorch的LSTM时间序列预测的示例代码:
```python
import torch
import torch.nn as nn
import torch.optim as optim
import numpy as np
# 准备数据
# 时间序列数据
data = np.array([1, 2, 3, 4, 5, 6, 7, 8, 9, 10])
# 标签
labels = np.array([2, 4, 6, 8, 10, 12, 14, 16, 18, 20])
# 将数据集划分为训练集和测试集
train_data = data[:8]
train_labels = labels[:8]
test_data = data[8:]
test_labels = labels[8:]
# 定义LSTM模型
class LSTM(nn.Module):
def __init__(self, input_size, hidden_size, output_size):
super(LSTM, self).__init__()
self.hidden_size = hidden_size
self.lstm = nn.LSTM(input_size, hidden_size)
self.linear = nn.Linear(hidden_size, output_size)
def forward(self, input):
output, (hidden, cell) = self.lstm(input.view(len(input), 1, -1))
output = self.linear(output.view(len(input), -1))
return output[-1]
# 定义损失函数和优化器
model = LSTM(1, 10, 1)
criterion = nn.MSELoss()
optimizer = optim.Adam(model.parameters(), lr=0.01)
# 训练模型
for epoch in range(1000):
inputs = torch.from_numpy(train_data).float()
labels = torch.from_numpy(train_labels).float()
optimizer.zero_grad()
outputs = model(inputs)
loss = criterion(outputs, labels)
loss.backward()
optimizer.step()
if epoch % 100 == 0:
print("Epoch {} Loss: {:.4f}".format(epoch, loss.item()))
# 测试模型
test_inputs = torch.from_numpy(test_data).float()
expected_outputs = torch.from_numpy(test_labels).float()
with torch.no_grad():
predicted_outputs = model(test_inputs)
test_loss = criterion(predicted_outputs, expected_outputs)
print("Test Loss: {:.4f}".format(test_loss.item()))
print("Expected Outputs: {}".format(expected_outputs))
print("Predicted Outputs: {}".format(predicted_outputs))
```
这段代码演示了如何使用PyTorch实现基于LSTM的时间序列预测。在这个示例中,我们使用一个简单的时间序列数据集来训练和测试模型。你可以将这个示例代码作为参考,根据你的数据集进行必要的修改。
写一个基于pytorch的LSTM时间序列数据预测程序
以下是一个基于PyTorch的LSTM时间序列数据预测程序,这里以预测某股票的价格为例:
```python
import numpy as np
import pandas as pd
import torch
import torch.nn as nn
from torch.autograd import Variable
# 数据预处理
def create_dataset(data, look_back=1):
dataX, dataY = [], []
for i in range(len(data)-look_back):
dataX.append(data[i:(i+look_back)])
dataY.append(data[i+look_back])
return np.array(dataX), np.array(dataY)
# 导入数据
df = pd.read_csv('data.csv')
df = df.dropna()
data = df['close'].values.astype('float32')
# 数据归一化
from sklearn.preprocessing import MinMaxScaler
scaler = MinMaxScaler(feature_range=(0, 1))
data = scaler.fit_transform(data.reshape(-1, 1))
# 创建训练集和测试集
train_size = int(len(data) * 0.7)
test_size = len(data) - train_size
train, test = data[0:train_size,:], data[train_size:len(data),:]
# 创建输入输出数据
look_back = 5
trainX, trainY = create_dataset(train, look_back)
testX, testY = create_dataset(test, look_back)
# 转换为PyTorch tensor
trainX = torch.from_numpy(trainX).type(torch.Tensor)
trainY = torch.from_numpy(trainY).type(torch.Tensor)
testX = torch.from_numpy(testX).type(torch.Tensor)
testY = torch.from_numpy(testY).type(torch.Tensor)
# 定义LSTM模型
class LSTM(nn.Module):
def __init__(self, input_size, hidden_size, output_size):
super(LSTM, self).__init__()
self.hidden_size = hidden_size
self.lstm = nn.LSTM(input_size, hidden_size, batch_first=True)
self.fc = nn.Linear(hidden_size, output_size)
def forward(self, x):
h0 = torch.zeros(1, x.size(0), self.hidden_size).requires_grad_()
c0 = torch.zeros(1, x.size(0), self.hidden_size).requires_grad_()
out, (hn, cn) = self.lstm(x, (h0.detach(), c0.detach()))
out = self.fc(out[:, -1, :])
return out
# 训练模型
input_size = 1
hidden_size = 32
output_size = 1
num_epochs = 1000
learning_rate = 0.01
lstm = LSTM(input_size, hidden_size, output_size)
criterion = nn.MSELoss()
optimizer = torch.optim.Adam(lstm.parameters(), lr=learning_rate)
for epoch in range(num_epochs):
outputs = lstm(trainX)
optimizer.zero_grad()
loss = criterion(outputs, trainY)
loss.backward()
optimizer.step()
if epoch % 100 == 0:
print('Epoch [{}/{}], Loss: {:.4f}'.format(epoch+1, num_epochs, loss.item()))
# 测试模型
lstm.eval()
train_predict = lstm(trainX)
test_predict = lstm(testX)
# 反归一化
train_predict = scaler.inverse_transform(train_predict.detach().numpy())
trainY = scaler.inverse_transform(trainY.detach().numpy())
test_predict = scaler.inverse_transform(test_predict.detach().numpy())
testY = scaler.inverse_transform(testY.detach().numpy())
# 计算MSE
from sklearn.metrics import mean_squared_error
trainScore = mean_squared_error(trainY, train_predict)
testScore = mean_squared_error(testY, test_predict)
print('Train Score: {:.2f} MSE'.format(trainScore))
print('Test Score: {:.2f} MSE'.format(testScore))
```
在这个程序中,我们首先对原始数据进行了数据归一化,并将数据分为训练集和测试集。然后,我们使用create_dataset函数将时间序列数据转换为有监督学习的格式,并使用PyTorch的Variable来存储数据。接着,我们定义了一个LSTM模型,并使用均方误差作为损失函数进行训练。最后,我们使用训练好的模型对训练集和测试集进行预测,并计算了预测的均方误差。
阅读全文
相关推荐
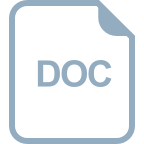
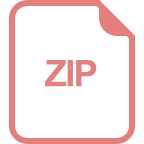
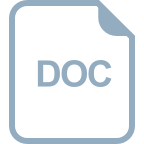
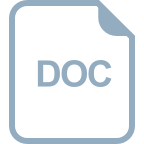
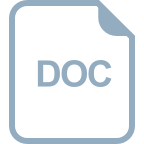
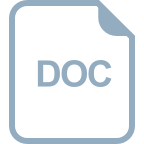
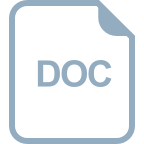







