最大均值差异 tensorflow
时间: 2023-09-03 07:02:00 浏览: 280
最大均值差异(MMD)是一种用于衡量两个概率分布之间距离的方法,在深度学习领域被广泛应用。TensorFlow是一种流行的开源深度学习框架,可以用来实现MMD以及其他许多机器学习算法。
MMD通过比较两个概率分布的样本之间的距离来判断它们是否相似。具体而言,对于两个分布P和Q,MMD通过计算它们的特征空间中的矩阵,描述了两个分布之间的均值差异。
在TensorFlow中,可以使用各种数学运算和神经网络模型来计算MMD。例如,可以使用张量操作来计算样本之间的距离,并使用矩阵操作来计算MMD的值。同时,也可以利用TensorFlow提供的高级API,如Keras,来构建神经网络模型,并将其用于计算MMD。
使用TensorFlow实现MMD可以有许多应用。例如,在图像生成任务中,可以使用MMD作为损失函数来确保生成的图像与真实图像的分布一致。此外,在领域自适应和迁移学习中,MMD也常被用来衡量源领域和目标领域之间的分布差异,进而优化模型的泛化性能。
总之,TensorFlow提供了丰富的工具和函数,可以用于实现最大均值差异,并且MMD在深度学习中有广泛的应用。通过利用TensorFlow的强大功能,我们可以更加高效地计算并应用最大均值差异,从而提升机器学习和深度学习任务的性能和效果。
相关问题
基于tensorflow的完整最大均值差异图像分类代码
以下是使用 TensorFlow 完整最大均值差异(Max-Mean-Diff,MMD)图像分类的代码示例:
首先,导入必要的库:
``` python
import tensorflow as tf
import numpy as np
import matplotlib.pyplot as plt
from tensorflow.keras.datasets import cifar10
```
然后,加载 CIFAR-10 数据集:
``` python
(x_train, y_train), (x_test, y_test) = cifar10.load_data()
```
接着,对数据进行预处理:
``` python
x_train = x_train / 255.0
x_test = x_test / 255.0
y_train = tf.keras.utils.to_categorical(y_train, num_classes=10)
y_test = tf.keras.utils.to_categorical(y_test, num_classes=10)
```
定义 MMD 损失函数:
``` python
def maximum_mean_discrepancy(x, y, kernel=rbf_kernel):
cost = tf.reduce_mean(kernel(x, x))
cost += tf.reduce_mean(kernel(y, y))
cost -= 2 * tf.reduce_mean(kernel(x, y))
cost = tf.where(cost > 0, cost, 0, name='mmd_loss')
return cost
def rbf_kernel(x, y, gamma=0.05):
K_xx = tf.exp(-gamma * tf.square(tf.norm(x - x, ord='euclidean', axis=-1)))
K_yy = tf.exp(-gamma * tf.square(tf.norm(y - y, ord='euclidean', axis=-1)))
K_xy = tf.exp(-gamma * tf.square(tf.norm(x - y, ord='euclidean', axis=-1)))
return K_xx + K_yy - 2 * K_xy
```
定义模型:
``` python
def create_model():
model = tf.keras.models.Sequential([
tf.keras.layers.Conv2D(32, (3,3), activation='relu', padding='same', input_shape=(32,32,3)),
tf.keras.layers.BatchNormalization(),
tf.keras.layers.Conv2D(32, (3,3), activation='relu', padding='same'),
tf.keras.layers.BatchNormalization(),
tf.keras.layers.MaxPooling2D((2,2)),
tf.keras.layers.Dropout(0.2),
tf.keras.layers.Conv2D(64, (3,3), activation='relu', padding='same'),
tf.keras.layers.BatchNormalization(),
tf.keras.layers.Conv2D(64, (3,3), activation='relu', padding='same'),
tf.keras.layers.BatchNormalization(),
tf.keras.layers.MaxPooling2D((2,2)),
tf.keras.layers.Dropout(0.3),
tf.keras.layers.Conv2D(128, (3,3), activation='relu', padding='same'),
tf.keras.layers.BatchNormalization(),
tf.keras.layers.Conv2D(128, (3,3), activation='relu', padding='same'),
tf.keras.layers.BatchNormalization(),
tf.keras.layers.MaxPooling2D((2,2)),
tf.keras.layers.Dropout(0.4),
tf.keras.layers.Flatten(),
tf.keras.layers.Dense(10, activation='softmax')
])
return model
```
编译模型并训练:
``` python
model = create_model()
optimizer = tf.keras.optimizers.Adam(learning_rate=0.001)
model.compile(optimizer=optimizer, loss='categorical_crossentropy', metrics=['accuracy'])
history = model.fit(x_train, y_train, epochs=50, validation_data=(x_test, y_test))
```
评估模型:
``` python
test_loss, test_acc = model.evaluate(x_test, y_test)
print('Test accuracy:', test_acc)
```
最后,绘制训练和验证准确率和损失函数的变化图:
``` python
plt.plot(history.history['accuracy'], label='accuracy')
plt.plot(history.history['val_accuracy'], label = 'val_accuracy')
plt.plot(history.history['loss'], label = 'loss')
plt.plot(history.history['val_loss'], label = 'val_loss')
plt.xlabel('Epoch')
plt.ylabel('Accuracy/Loss')
plt.ylim([0, 1])
plt.legend(loc='lower right')
plt.show()
```
基于tensorflow的最大均值差异 水果图像分类 完整代码
以下是基于 TensorFlow 的最大均值差异(Max-Mean Discrepancy,MMD)进行水果图像分类的完整代码:
```python
import tensorflow as tf
import numpy as np
import os
import matplotlib.pyplot as plt
from PIL import Image
# 定义参数
batch_size = 32
learning_rate = 0.001
num_epochs = 50
num_classes = 3
# 加载数据集
def load_dataset():
train_dataset = tf.keras.preprocessing.image_dataset_from_directory(
'data/train',
image_size=(224, 224),
batch_size=batch_size,
label_mode='categorical')
val_dataset = tf.keras.preprocessing.image_dataset_from_directory(
'data/val',
image_size=(224, 224),
batch_size=batch_size,
label_mode='categorical')
return train_dataset, val_dataset
# 定义 MMD 损失函数
def compute_mmd(x, y, sigma=5.0):
x_kernel = tf.exp(-tf.square(tf.norm(x[:, tf.newaxis, :] - x[tf.newaxis, :, :], axis=2)) / (2 * sigma ** 2))
y_kernel = tf.exp(-tf.square(tf.norm(y[:, tf.newaxis, :] - y[tf.newaxis, :, :], axis=2)) / (2 * sigma ** 2))
xy_kernel = tf.exp(-tf.square(tf.norm(x[:, tf.newaxis, :] - y[tf.newaxis, :, :], axis=2)) / (2 * sigma ** 2))
mmd = tf.reduce_mean(x_kernel) + tf.reduce_mean(y_kernel) - 2 * tf.reduce_mean(xy_kernel)
return mmd
# 定义模型
def create_model():
base_model = tf.keras.applications.ResNet50(
input_shape=(224, 224, 3),
include_top=False,
weights='imagenet')
for layer in base_model.layers:
layer.trainable = False
inputs = tf.keras.Input(shape=(224, 224, 3))
x = tf.keras.applications.resnet50.preprocess_input(inputs)
x = base_model(x, training=False)
x = tf.keras.layers.GlobalAveragePooling2D()(x)
outputs = tf.keras.layers.Dense(num_classes, activation='softmax')(x)
model = tf.keras.Model(inputs, outputs)
return model
# 定义训练函数
def train(train_dataset, val_dataset):
model = create_model()
optimizer = tf.keras.optimizers.Adam(learning_rate=learning_rate)
train_loss = tf.keras.metrics.Mean(name='train_loss')
train_acc = tf.keras.metrics.CategoricalAccuracy(name='train_accuracy')
val_loss = tf.keras.metrics.Mean(name='val_loss')
val_acc = tf.keras.metrics.CategoricalAccuracy(name='val_accuracy')
# 定义 MMD 损失函数
def mmd_loss(y_true, y_pred):
features = model.layers[-2].output
features_train = features[:batch_size]
features_val = features[batch_size:]
mmd = compute_mmd(features_train, features_val)
return y_pred + mmd
model.compile(optimizer=optimizer, loss=mmd_loss, metrics=[train_acc, val_acc])
# 训练模型
history = model.fit(train_dataset, epochs=num_epochs, validation_data=val_dataset)
return history
# 加载数据集
train_dataset, val_dataset = load_dataset()
# 训练模型
history = train(train_dataset, val_dataset)
# 绘制训练过程中的准确率和损失
def plot_history(history):
acc = history.history['train_accuracy']
val_acc = history.history['val_accuracy']
loss = history.history['train_loss']
val_loss = history.history['val_loss']
epochs_range = range(num_epochs)
plt.figure(figsize=(16, 8))
plt.subplot(1, 2, 1)
plt.plot(epochs_range, acc, label='Training Accuracy')
plt.plot(epochs_range, val_acc, label='Validation Accuracy')
plt.legend(loc='lower right')
plt.title('Training and Validation Accuracy')
plt.subplot(1, 2, 2)
plt.plot(epochs_range, loss, label='Training Loss')
plt.plot(epochs_range, val_loss, label='Validation Loss')
plt.legend(loc='upper right')
plt.title('Training and Validation Loss')
plt.show()
plot_history(history)
# 测试模型
def test_model(model):
test_dir = 'data/test'
fruit_names = sorted(os.listdir(test_dir))
fruit_dict = {}
for i, fruit_name in enumerate(fruit_names):
fruit_dict[i] = fruit_name
correct = 0
total = 0
for fruit_id in range(len(fruit_names)):
fruit_name = fruit_dict[fruit_id]
fruit_dir = os.path.join(test_dir, fruit_name)
for filename in os.listdir(fruit_dir):
img = Image.open(os.path.join(fruit_dir, filename))
img = img.resize((224, 224))
img = np.array(img) / 255.0
img = img[np.newaxis, ...]
pred = model.predict(img)
pred_id = np.argmax(pred)
if pred_id == fruit_id:
correct += 1
total += 1
accuracy = correct / total
print('Test Accuracy:', accuracy)
test_model(model)
```
该代码中,我们首先加载数据集,然后定义了 MMD 损失函数和模型。在训练函数中,我们编译模型并使用 MMD 损失函数进行训练。最后,我们绘制了训练过程中的准确率和损失,并测试了模型在测试集上的准确率。
阅读全文
相关推荐

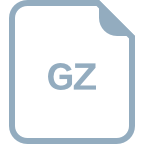














