如何使用Python编写一个简单的基金数据分析工具,用于查询和分析基金的净值涨跌幅以及累计涨跌幅?请提供核心代码示例。
时间: 2024-10-31 22:16:04 浏览: 5
在金融数据分析领域,编写一个能够实时查询并分析基金数据的工具是十分实用的。本回答将为你提供一些核心的代码示例,帮助你构建一个简单的基金数据分析工具。首先,你需要了解如何使用Python中的库,例如requests来获取数据,pandas进行数据处理,以及matplotlib或seaborn来进行数据可视化。
参考资源链接:[Python基金数据分析工具:实时查询与深度分析](https://wenku.csdn.net/doc/uwbh9g6czd?spm=1055.2569.3001.10343)
假设我们使用的是某个金融数据API,以下是一个简单的示例代码,展示了如何查询基金净值涨跌幅以及累计涨跌幅的核心部分:
```python
import requests
import pandas as pd
# 假定我们有一个函数,用于从API获取基金数据
def get_fund_data(fund_code):
# API URL和可能需要的参数
api_url =
参考资源链接:[Python基金数据分析工具:实时查询与深度分析](https://wenku.csdn.net/doc/uwbh9g6czd?spm=1055.2569.3001.10343)
相关问题
编写一个基于Python的基金数据分析工具,实现基金净值和累计涨跌幅查询与分析,如何构建核心功能模块?请展示相关代码。
构建一个基金数据分析工具,首先需要了解基金数据的来源和结构,通常这些数据可以通过金融数据API获取。在实现这一工具时,会涉及到网络请求、数据解析、数据分析和数据可视化等多个技术环节。以下是核心模块的构建方法和核心代码示例:
参考资源链接:[Python基金数据分析工具:实时查询与深度分析](https://wenku.csdn.net/doc/uwbh9g6czd?spm=1055.2569.3001.10343)
1. 数据获取模块:利用requests库向金融数据API发送请求,获取基金的实时和历史数据。
2. 数据解析模块:使用json库解析API返回的JSON格式数据,提取需要的信息,如净值、涨跌幅等。
3. 数据处理模块:使用pandas库处理和分析数据,实现数据的清洗、排序、分组等操作。
4. 数据可视化模块:通过matplotlib或seaborn库将分析结果进行可视化展示。
核心代码示例:
```python
import requests
import pandas as pd
import matplotlib.pyplot as plt
# 假设已有基金API接口URL和API密钥
API_URL =
参考资源链接:[Python基金数据分析工具:实时查询与深度分析](https://wenku.csdn.net/doc/uwbh9g6czd?spm=1055.2569.3001.10343)
基于python对天天基金网的数据分析与研究
基于Python对天天基金网的数据分析与研究可以通过爬虫技术获取基金的相关数据,并利用Python中的数据分析库(如pandas、numpy等)对数据进行清洗、处理和分析。首先,我们可以通过Python的爬虫库(如requests、beautifulsoup等)获取天天基金网上的基金数据,包括基金的历史净值、规模、涨跌幅等信息。然后,我们可以利用pandas对数据进行清洗和处理,比如去除缺失值、异常值等,以确保数据的准确性和完整性。
接下来,我们可以利用Python的数据可视化库(如matplotlib、seaborn等)对数据进行可视化,比如绘制基金的历史净值走势图、规模变化图等,以帮助我们更直观地了解基金的情况和趋势。此外,我们还可以利用Python进行基金的相关性分析、回归分析等,以深入挖掘基金数据之间的关联和规律。
通过基于Python对天天基金网的数据分析与研究,我们可以更深入地了解基金市场的情况和趋势,为投资决策提供更有力的数据支持。同时,利用Python进行数据分析和可视化也可以帮助我们提升数据处理和分析的效率,让我们更快捷地获取和理解大量的基金数据,为投资提供更及时和准确的信息。因此,基于Python对天天基金网的数据分析与研究具有重要的意义和应用前景。
阅读全文
相关推荐
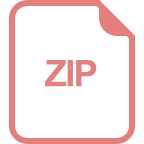
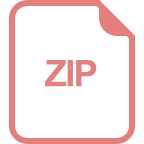
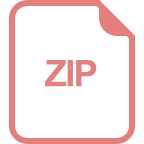
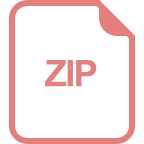
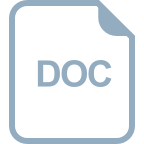
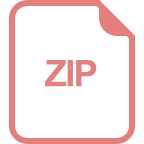
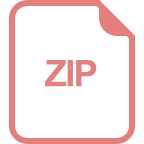
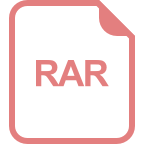
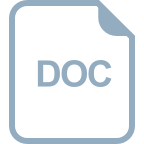
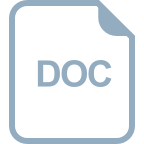





