试用sklearn的svc函数对西瓜数据集3.0a进行分类,画出散点图和分离超平面。并与logistic分类方法比较。
时间: 2024-12-13 11:19:38 浏览: 12
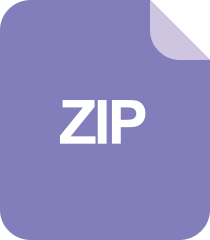
西瓜数据集3.0-数据集
在Python的scikit-learn库中,可以使用SVC(Support Vector Classifier,支持向量机分类器)对西瓜数据集(如Iris或Digits等,这里假设你是指一个虚构的“西瓜”数据集)进行二元或多类别分类。首先,你需要加载数据,然后划分训练集和测试集,接着实例化SVC模型并训练它。预测结果之后,你可以用matplotlib库绘制二维空间上的散点图,并标出决策边界。
```python
import numpy as np
import matplotlib.pyplot as plt
from sklearn import datasets
from sklearn.model_selection import train_test_split
from sklearn.svm import SVC
from sklearn.linear_model import LogisticRegression
# 加载假设的数据集(这里需要实际数据)
# 西瓜数据集 = load_iris() 或者其他类似函数
X, y = ... # 假设已经加载好特征和标签
# 划分训练集和测试集
X_train, X_test, y_train, y_test = train_test_split(X, y, test_size=0.2, random_state=42)
# 创建SVC模型
svc_model = SVC(kernel='linear') # 线性核(也可以尝试‘rbf’、‘poly’等)
svc_model.fit(X_train, y_train)
# 预测和绘图
y_pred_svc = svc_model.predict(X_test)
plt.scatter(X_test[:, 0], X_test[:, 1], c=y_pred_svc, cmap='viridis')
line = svm_line(svc_model.support_vectors_, svc_model.coef_[0])
plt.plot(line, color='red', linestyle='-', linewidth=2) # 绘制分离超平面
plt.xlabel('Feature 1')
plt.ylabel('Feature 2')
plt.title('SVM Classification with Linear Kernel')
# 对比Logistic Regression
lr_model = LogisticRegression()
lr_model.fit(X_train, y_train)
y_pred_lr = lr_model.predict(X_test)
...
# 绘制LR的分类边界
# 比较性能
print(f"SVC accuracy: {svc_model.score(X_test, y_test)}")
print(f"Logistic Regression accuracy: {lr_model.score(X_test, y_test)}")
阅读全文