fx = 4 * pi * x / 9求解最优解的matlab代码
时间: 2024-09-06 10:05:31 浏览: 50
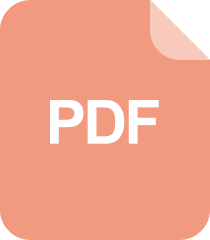
傅里叶级数展开matlab实现.pdf
要在MATLAB中求解函数`fx = 4 * pi * x / 9`的最优解,通常是指寻找使得函数值最小或最大的x值。对于这个特定的函数,它是一个正比例函数,随着x的增大,函数值线性增大,因此不存在最大值,只有最小值,而最小值在x趋近于负无穷时趋近于负无穷。
如果我们的目标是找到函数的最小值,可以简单地将x设置为一个很大的负数,因为在正比例函数中,当x为负时,函数值会减少。在MATLAB中,我们可以使用`fminbnd`函数来寻找一个闭区间上的函数最小值。但是由于`fminbnd`寻找的是区间内的局部最小值,对于线性函数来说,除非设置一个很小的区间,否则它不会在默认区间内找到最小值。
以下是一个使用`fminbnd`寻找`fx`在区间`[-100, 100]`内最小值的MATLAB代码示例:
```matlab
function fx = objective_function(x)
fx = 4 * pi * x / 9;
end
% 设置搜索区间
lb = -100; % 下界
ub = 100; % 上界
% 调用fminbnd寻找最小值
[x_min, fx_min] = fminbnd(@objective_function, lb, ub);
% 显示结果
fprintf('在区间[%d, %d]内,函数的最小值是 %f,在x = %f时取得。\n', lb, ub, fx_min, x_min);
```
请注意,这个代码只是在指定区间内寻找最小值,而不是全局最小值。对于正比例函数,由于其无限延伸的特性,没有全局最小值。
阅读全文
相关推荐
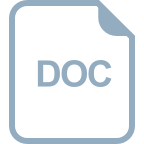
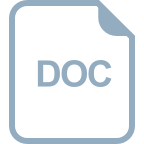
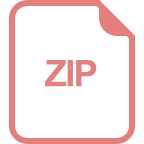
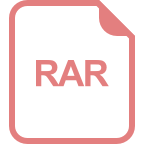
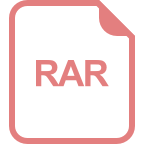
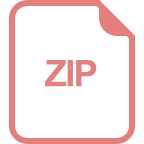
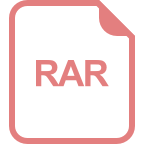
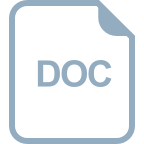
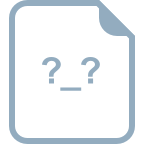
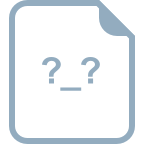
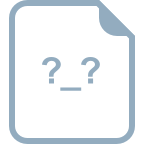
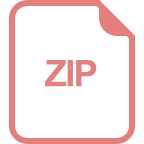
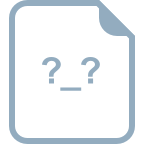